Online state and time-varying parameter estimation using the implicit equal-weights particle filter
QUARTERLY JOURNAL OF THE ROYAL METEOROLOGICAL SOCIETY(2024)
摘要
A method is proposed for resilient and efficient estimation of the states and time-varying parameters in nonlinear high-dimensional systems through a sequential data assimilation process. The importance of estimating time-varying parameters lies not only in improving prediction accuracy but also in determining when model characteristics change. We propose a particle-filter-based method that incorporates nudging techniques inspired by optimization algorithms in machine learning by taking advantage of the flexibility of the proposal density in particle filtering. However, as the model resolution and number of observations increase, filter degeneracy tends to be the obstacle to implementing the particle filter. Therefore, this proposed method is combined with the implicit equal-weights particle filter (IEWPF), in which all particle weights are equal. The method is validated using the 1000-dimensional linear model with an additive parameter and the 1000-dimensional Lorenz-96 model, where the forcing term is parameterized. The method is shown to be capable of resilient and efficient parameter estimation for parameter changes over time in our application with a linear observation operator. This leads to the conjecture that it applies to realistic geophysical, climate, and other problems. We developed a state and parameter estimation method based on an implicit equal-weight particle filter, incorporating optimization-inspired nudging. The graphs compare the parameter trajectories of (a) the augmented method, (b) results with a correlated covariance matrix, and (c) results with nudging in addition to (b), using a 1000-dimensional Lorenz-96 model with parameterized forcing terms. The solid line (blue) represents each of the 20 ensemble members, and the dashed line (red) represents the true parameter values. The proposed method (c) avoids filter degeneracy and can track abrupt changes in all three parameters. image
更多查看译文
关键词
data assimilation,nondegeneracy,parameter estimation,particle filter
AI 理解论文
溯源树
样例
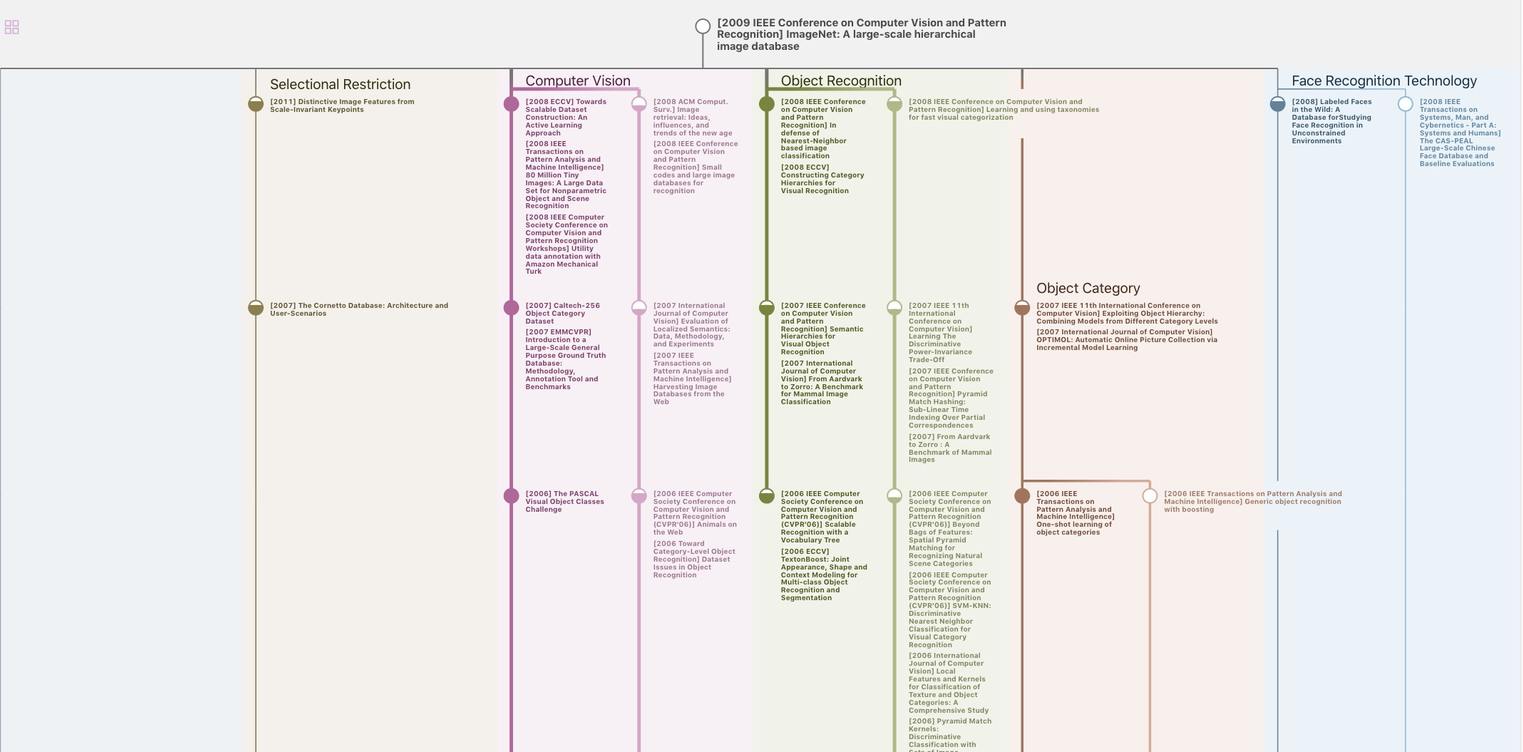
生成溯源树,研究论文发展脉络
Chat Paper
正在生成论文摘要