Estimation under inequality constraints in univariate and multivariate linear models
crossref(2024)
Abstract
In this paper least squares and maximum likelihood estimates under univariate and multivariate linear models with a priori information related to maximum effects in the models are determined. Both loss functions (the least squares and negative log-likelihood) and the constraints are convex, so the convex optimization theory can be utilized to obtain estimates, which in this paper are called Safety belt estimates. In particular, the complementary slackness condition, common in convex optimization, implies two alternative types of solutions, strongly dependent on the data and the restriction. It is experimentally shown that, despite of the similarity to the ridge regression estimation under the univariate linear model, the Safety belt estimates behave usually better than estimates obtained via ridge regression. Moreover, concerning the multivariate model, the proposed technique represents a completely novel approach.
MoreTranslated text
AI Read Science
Must-Reading Tree
Example
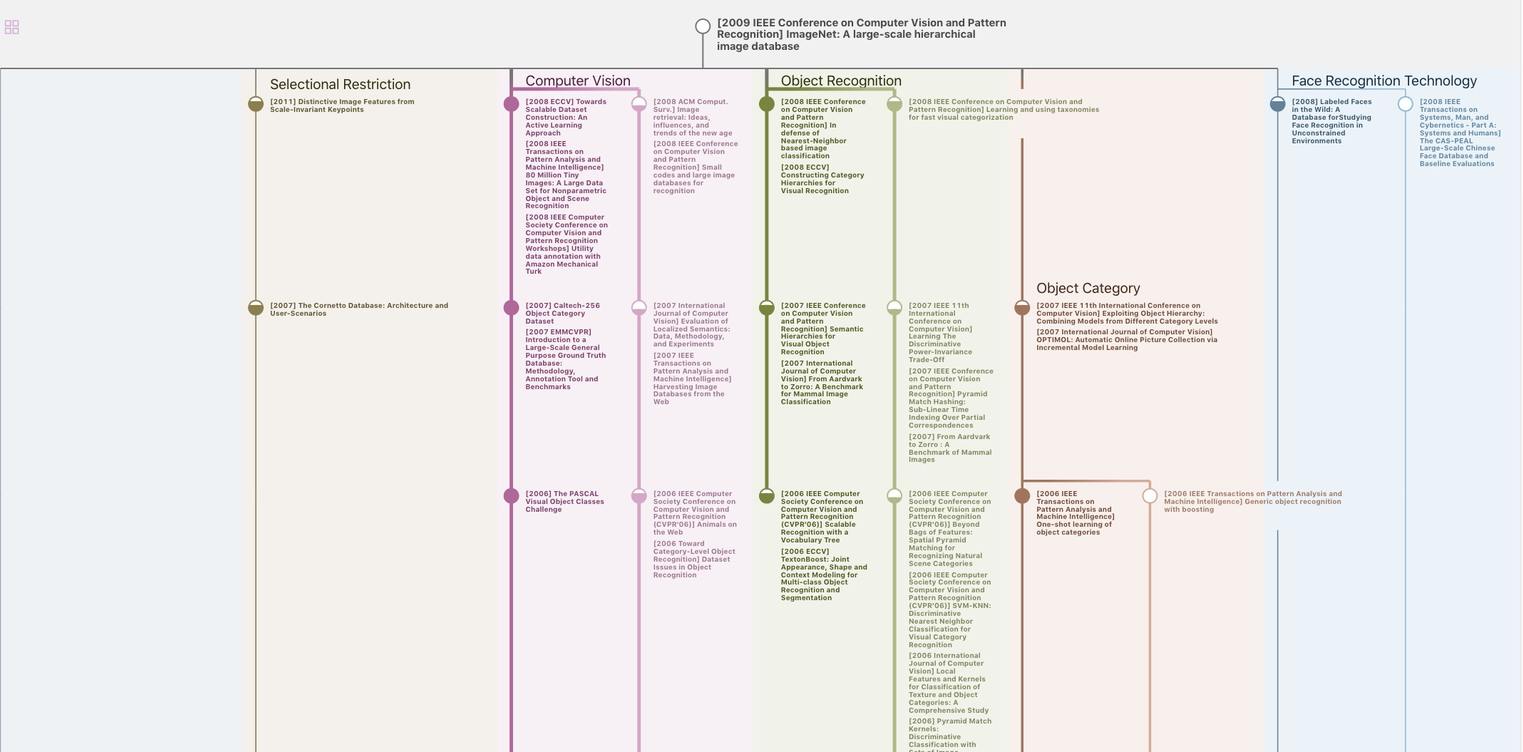
Generate MRT to find the research sequence of this paper
Chat Paper
Summary is being generated by the instructions you defined