Placement Path Optimization of Placement Machine Based on Rule Learning Iterative Method
2024 12th International Conference on Intelligent Control and Information Processing (ICICIP)(2024)
摘要
This paper integrates deep reinforcement learning with meta-heuristic search rules to address the placement path optimization problem for placement machine. As a critical component of the surface mounting technology (SMT) production line, the production speed of the placement machine directly influences overall production efficiency. While the prevalent approach utilizes meta-heuristic algorithms for search, which enhances solution quality but consumes more time, this article introduces a reinforcement learning model to accelerate the search process. By organizing existing metaheuristic algorithm rules into an operator library, establishing and fine-tuning the corresponding policy network, and creating training and test datasets based on real-world conditions, the algorithm is developed and subjected to data training. Ultimately, a comparison with the machine's native algorithm and the classic genetic algorithm demonstrates the superiority of this approach.
更多查看译文
关键词
Placement machine,placement path optimization,deep reinforcement learning,genetic algorithm
AI 理解论文
溯源树
样例
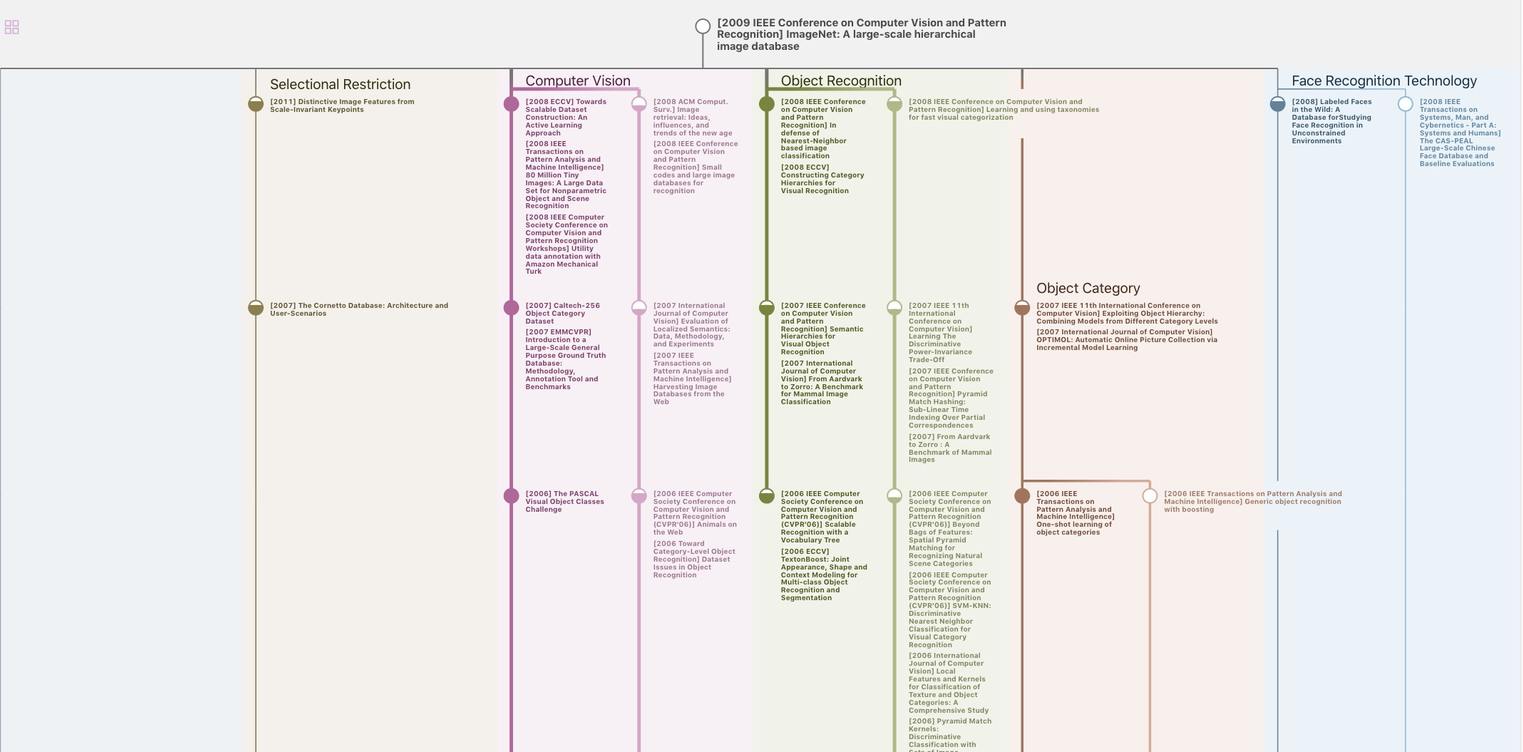
生成溯源树,研究论文发展脉络
Chat Paper
正在生成论文摘要