Robustness of Updatable Learning-based Index Advisors against Poisoning Attack
Proceedings of the ACM on Management of Data(2024)
摘要
Despite the promising performance of recent learning-based Index Advisors (IAs), they exhibited the robustness issue when poisoning attacks polluted training data. This paper presents the first attempt to study the robustness of updatable learning-based IAs against poisoning attack, i.e., whether the IAs can maintain robust performance if their training/updating is disturbed by injecting an extraneous toxic workload. The goal is to provide an opaque-box stress test that is generally effective in evaluating the robustness of different learning-based IAs without using the users' private data. There are three challenges, i.e., how to probe "index preference" from opaque-box IAs, how to design effective injecting strategies even if the IAs can be fine-tuned, and how to generate queries to meet the specific constraints for IA probing and injecting. The presented stress-test framework PIPA consists of a probing stage, an injecting stage, and a query generator. To address the first challenge, the probing stage estimates the IA's indexing preference by observing its responses to the probing workload. To address the second challenge, the injecting stage injects workloads that spoof the IA to demote the top-ranked indexes in the estimated indexing preference and promote mid-ranked indexes. The stress test is effective because the IA is trapped in a local optimum even after fine-tuning. To address the third challenge, PIPA utilizes IABART (Index Aware BART) to generate queries that can be optimized by building indexes on a given set of indexes. Extensive experiments on different benchmarks against various learning-based IAs demonstrate the effectiveness of PIPA and that existing learning-based IAs are non-robust when faced with even a subtle amount of injected extraneous toxic workloads.
更多查看译文
关键词
AI4DB,learning-based index advisor,poisoning attack
AI 理解论文
溯源树
样例
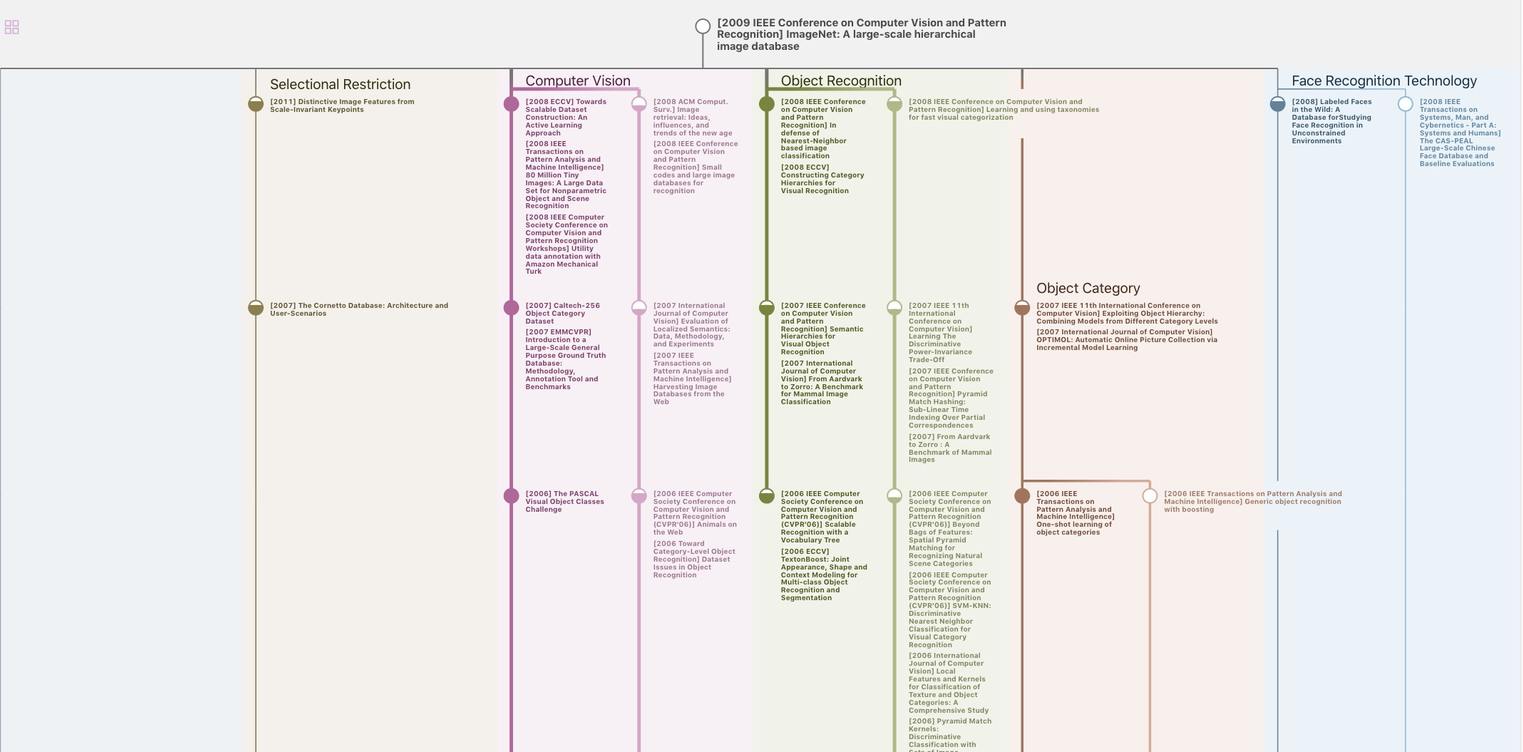
生成溯源树,研究论文发展脉络
Chat Paper
正在生成论文摘要