PECJ: Stream Window Join on Disorder Data Streams with Proactive Error Compensation
Proceedings of the ACM on Management of Data(2024)
摘要
Stream Window Join (SWJ), a vital operation in stream analytics, struggles with achieving a balance between accuracy and latency due to out-of-order data arrivals. Existing methods predominantly rely on adaptive buffering, but often fall short in performance, thereby constraining practical applications. We introduce PECJ, a solution that proactively incorporates unobserved data to enhance accuracy while reducing latency, thus requiring robust predictive modeling of stream oscillation. At the heart of PECJ lies a mathematical formulation of the posterior distribution approximation (PDA) problem using variational inference (VI). This approach circumvents error propagation while meeting the low-latency demands of SWJ. We detail the implementation of PECJ, striking a balance between complexity and generality, and discuss both analytical and learning-based approaches. Experimental evaluations reveal PECJ's superior performance. The successful integration of PECJ into a multi-threaded SWJ benchmark testbed further establishes its practical value, demonstrating promising advancements in enhancing data stream processing capabilities amidst out-of-order data.
更多查看译文
关键词
data stream,error compensation,out-of-order arrival,variational methods
AI 理解论文
溯源树
样例
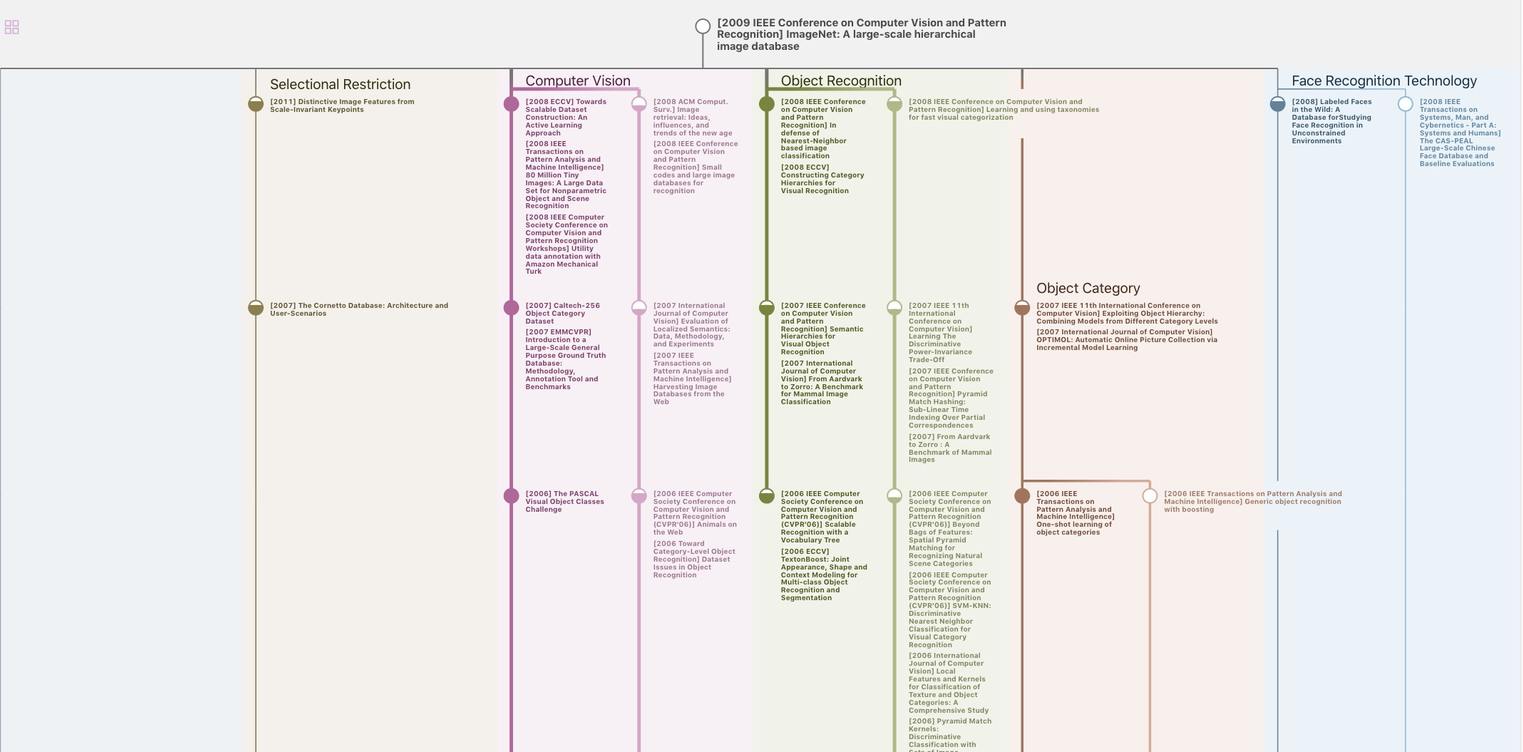
生成溯源树,研究论文发展脉络
Chat Paper
正在生成论文摘要