CCML: Curriculum and Contrastive Learning Enhanced Meta-Learner for Personalized Spatial Trajectory Prediction
IEEE Transactions on Knowledge and Data Engineering(2024)
摘要
Spatial trajectory prediction is a fundamental problem for diverse location-based applications. However, existing methods fall short in learning and generalization, and cannot sufficiently capture users’ spatiotemporal preferences, especially for cold-start users. Moreover, these methods do not explicitly consider the diversity of moving patterns among users and trajectories, i.e., the learning difficulty of different user and trajectory samples, thus hindering the improvement of prediction accuracy. To solve these problems, we propose a novel Curriculum and Contrastive Learning Enhanced Meta-Learner (CCML) that transfers knowledge from users with rich data to cold-start users. Specifically, a Contrastive-based Trajectory Predictor (CTP) is designed as the base model, which utilizes contrastive learning technique on both user-level and trajectory-level, aiming to facilitate a more profound understanding and differentiation of the varied travel behaviors and preferences exhibited by individuals. Meanwhile, CCML also incorporates the curriculum learning and the hard sample mining strategies. It simultaneously considers the learning difficulty of both user and trajectory samples, and presents the learning tasks by an easy-to-hard curriculum. By learning more challenging combinations of user and trajectory samples in each meta-learning iteration, the meta-learner can converge to a better status. Extensive experiments on two real-world datasets demonstrate the superiority of our models.
更多查看译文
关键词
Spatial trajectory prediction,contrastive learning,meta learning,curriculum learning
AI 理解论文
溯源树
样例
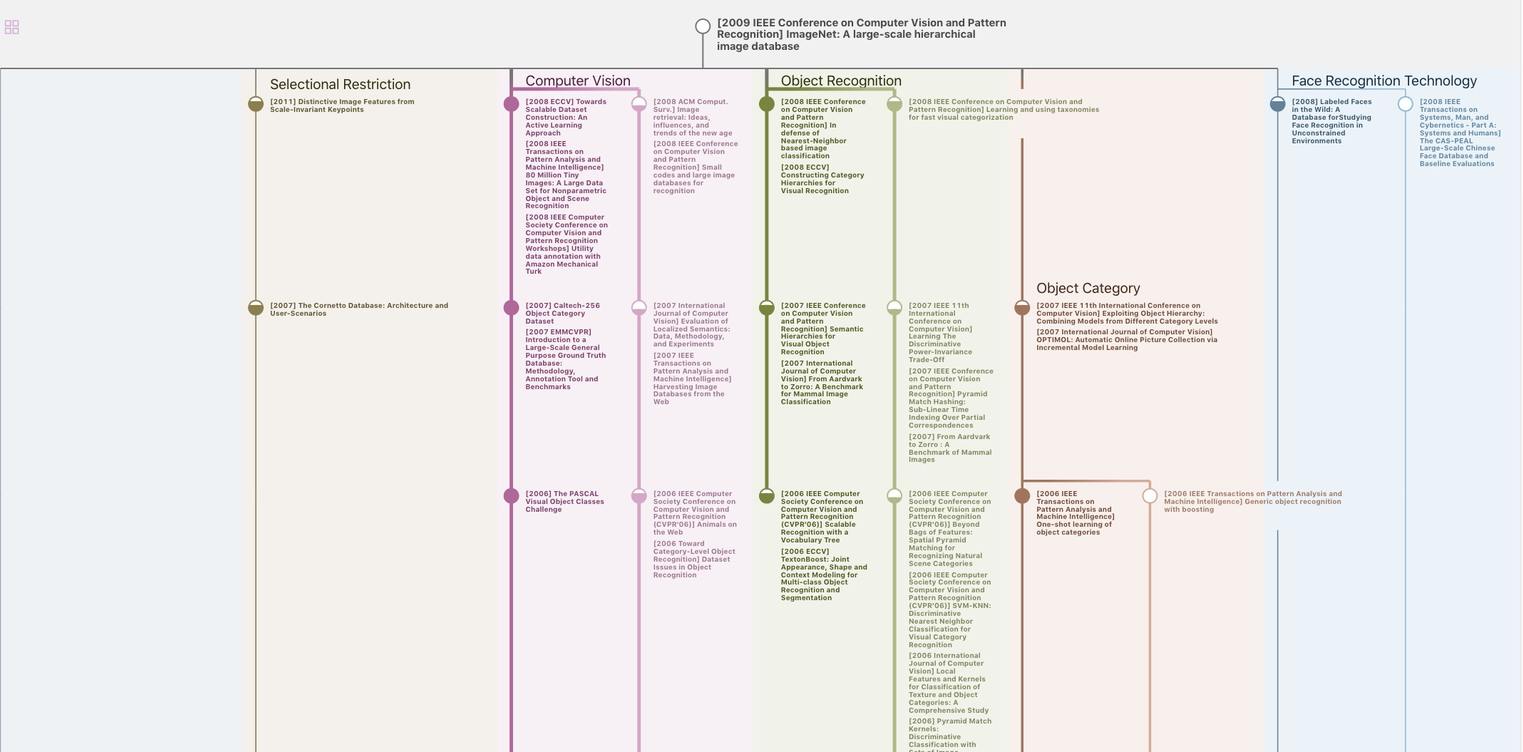
生成溯源树,研究论文发展脉络
Chat Paper
正在生成论文摘要