Communication-aware Quantization for Deep Learning Inference Parallelization on Chiplet-based Accelerators.
International Conference on Parallel and Distributed Systems(2023)
摘要
It has recently become trendy for neural network accelerators to scale from single-core to chiplet-based multichip architecture, as the growth of neural network depth and complexity are calling for the promotion of computation and memory capabilities. However, the unintended extensive inter-chip communication of chiplet-based accelerator may bottleneck the parallelism of deep learning inference, which is undesirable for many real-time applications and energy-efficient devices. Although it is imperative for novel schemes to be devised to alleviate this problem, related works are scarce. In this work, we present CampQ, a fine-grained communication-aware mixed-precision quantization method to accelerate inference parallelization by reducing the major inter-chiplet communication overhead. By leveraging the AutoML technique, CampQ is capable of determining different bit-width to activation groups according to thier transmission distances in on-package network. The experimental results show 1.4×-2.6× performance benefits and 29%-60% energy reduction over the 16-bit models for various neural networks and parallelism approaches.
更多查看译文
关键词
deep learning inference,chiplet,AutoML,quantization,inter-chip communication
AI 理解论文
溯源树
样例
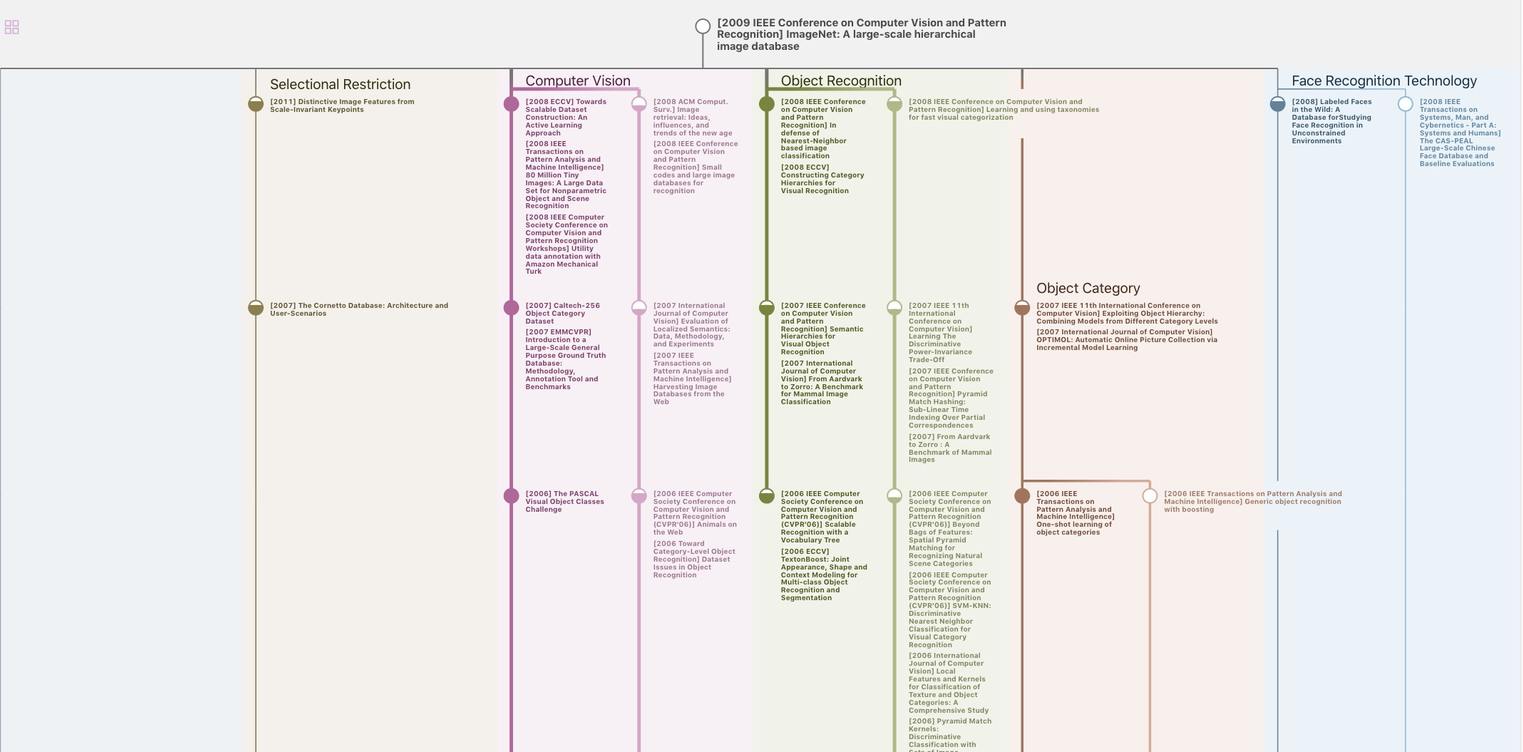
生成溯源树,研究论文发展脉络
Chat Paper
正在生成论文摘要