A coarse-to-fine parallelizable surface defect detection approach for railway trackside equipment.
International Conference on Parallel and Distributed Systems(2023)
摘要
Surface defects of railway trackside equipment pose a serious risk on the safety of railway transportation systems. Image-based surface defect detection methods have made significant progress. However, the image background of trackside equipment is complex, and there is a large amount of noise, which makes existing methods inadequate in accurately detecting small surface defect regions. To tackle with this issue, we propose a coarse-to-fine parallelizable surface defect detection approach to hierarchically detect the defects of trackside equipment. Firstly, a detection network is designed to locate and extract trackside equipment, which aims at roughly focusing the detection field from the original image to the region of interest of individual trackside equipment. Then, a novel semantic segmentation network is proposed to segment the major components of trackside equipment, so as to further finely focus on the defect regions. We apply multiple segmentation networks to parallelly segment various trackside equipment. In the segmentation network, a dense feature enhancement method is introduced to strengthen the high-level semantic information, and a feature partitioning enhancement strategy is designed to improve the segmentation performance for small defect regions. Finally, according to the visual characteristics of the segmentation output, we propose a defect recognizer to discriminate the defects. Extensive experimental results demonstrate that the proposed surface defect detection approach achieves higher accuracy for trackside equipment.
更多查看译文
关键词
defect detection,railway trackside equipment,coarse-to-fine,semantic segmentation
AI 理解论文
溯源树
样例
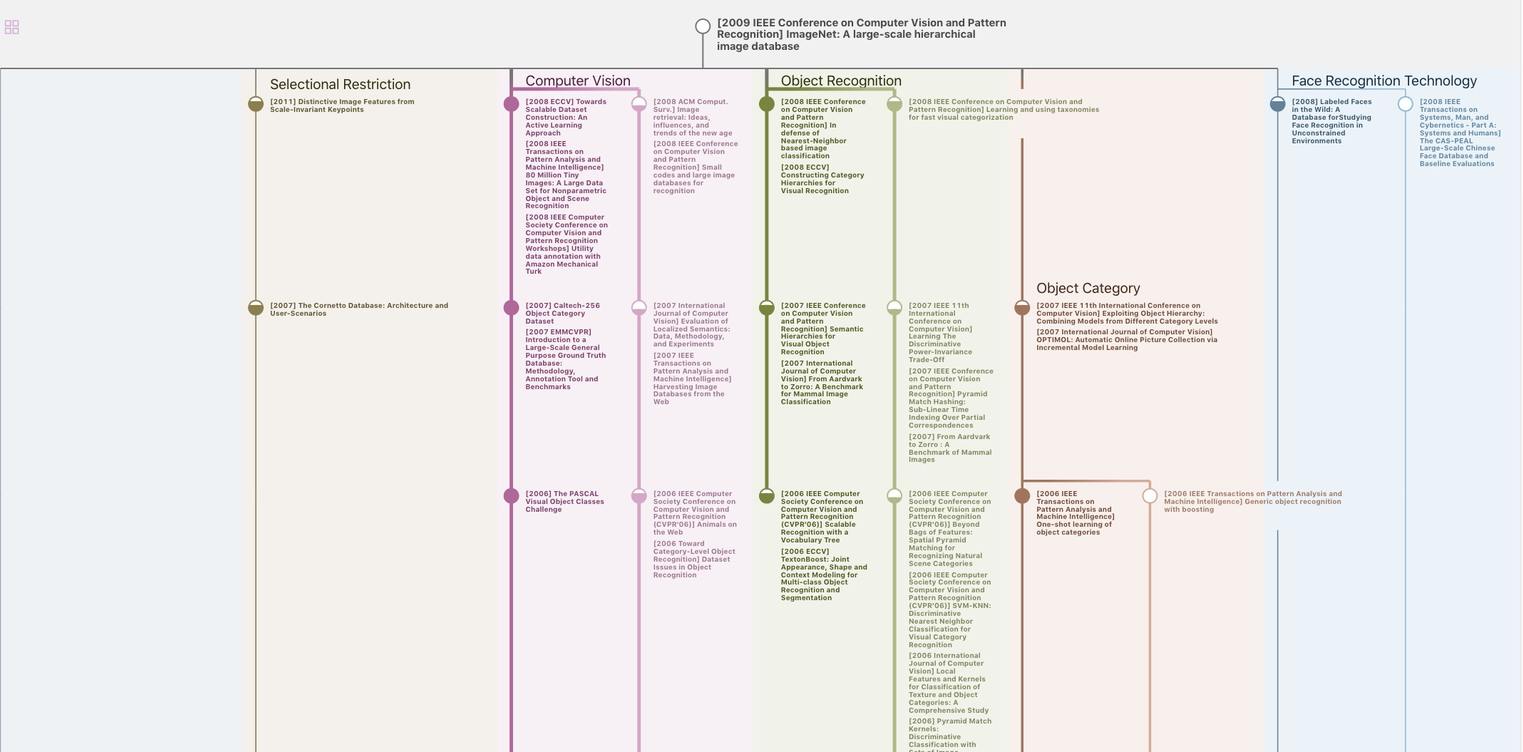
生成溯源树,研究论文发展脉络
Chat Paper
正在生成论文摘要