Learning Dynamic Spatial Graphs and Spatial Patterns for Accurate Traffic Prediction.
International Conference on Parallel and Distributed Systems(2023)
摘要
Traffic prediction poses a formidable challenge due to the dynamic and intricate spatial-temporal dependencies inherent in the task. Adaptive Spatial-Temporal Graph Neural Networks (ASTGNNs) have emerged as a promising solution, endeavoring to discern node-specific spatial patterns and autonomously deduce the spatial graphs among disparate traffic series. Nonetheless, the efficacy of existing ASTGNNs is often compromised as they struggle to apprehend the dynamic patterns of traffic series and deduce the dynamic spatial graphs in labyrinthine road networks, primarily due to their reliance on static node embeddings. In this paper, we introduce the Adaptive Dynamic Graph Convolutional Recurrent Network (ADGCRN), an innovative ASTGNN archetype adept at learning both dynamic spatial dependencies and dynamic spatial patterns from traffic data. Our model is distinguished by two instance-wise dynamic adaptive modules: i) the Instance-wise Dynamic Adaptive Graph Generation module, which integrates instance-wise state information and temporal periodicity into node embeddings; and ii) the Instance-wise Node Adaptive Parameter Learning module, which is capable of learning dynamic, instance-wise, and node-specific patterns for each traffic series. Rigorous experiments conducted on four benchmark datasets demonstrate that ADGCRN significantly outperforms the state-of-the-art ASTGNNs, achieving an average improvement of 3.45%, 3.11%, and 8.78% in terms of Mean Absolute Error (MAE), Root Mean Square Error (RMSE), and Mean Absolute Percentage Error (MAPE), respectively.
更多查看译文
关键词
traffic prediction,spatial-temporal graph neural networks
AI 理解论文
溯源树
样例
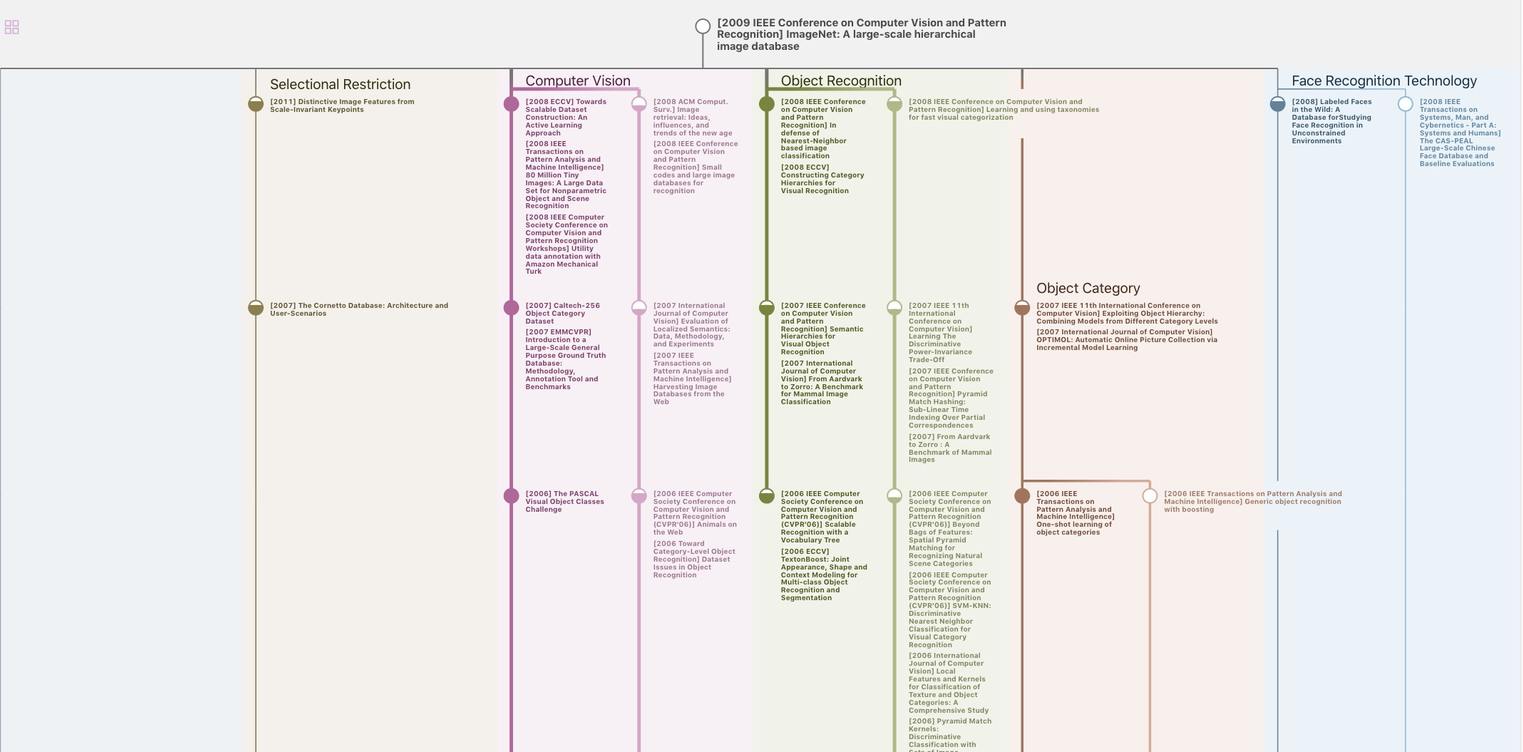
生成溯源树,研究论文发展脉络
Chat Paper
正在生成论文摘要