A Correlation And Order-Aware Rule Learning Method For Knowledge Graph Reasoning.
International Conference on Parallel and Distributed Systems(2023)
摘要
Mining high-quality logical rules is crucial as they can provide beneficial interpretability for predictions. Recent methods that incorporate logical rules into learning tasks have been proven to yield high-quality logical rules successfully. However, existing methods either rely on the rule instances observed to support rule mining, or simply embed the rule head and the rule body to learn from them. Additionally, they can not fully utilize the rich semantic information contained in logical rules and overlook the intrinsic correlations between all relations within the domain. In this paper, we propose a model called Correlation and order-Aware Rule Learning (CARL) that captures deeper semantic information in rules by allowing relations to be co-aware of each other and paying attention to logical sequence sensitivity. CARL utilizes semantic consistency between the rule body and rule head as its learning objective, continuously introducing more semantic information and logically simplifying the rule body while considering logical sequence sensitivity. We explored the internal correlations between domain relations and used the thought of knowledge distillation to simplify modules so that relations in CARL can share or perceive each other’s information or state efficiently. Experiments on link prediction tasks have demonstrated that CARL can learn higher-quality rules and yield state-of-the-art results on four popular public datasets. https://github.com/burning5112/CARL
更多查看译文
关键词
knowledge graph reasoning,rule learning
AI 理解论文
溯源树
样例
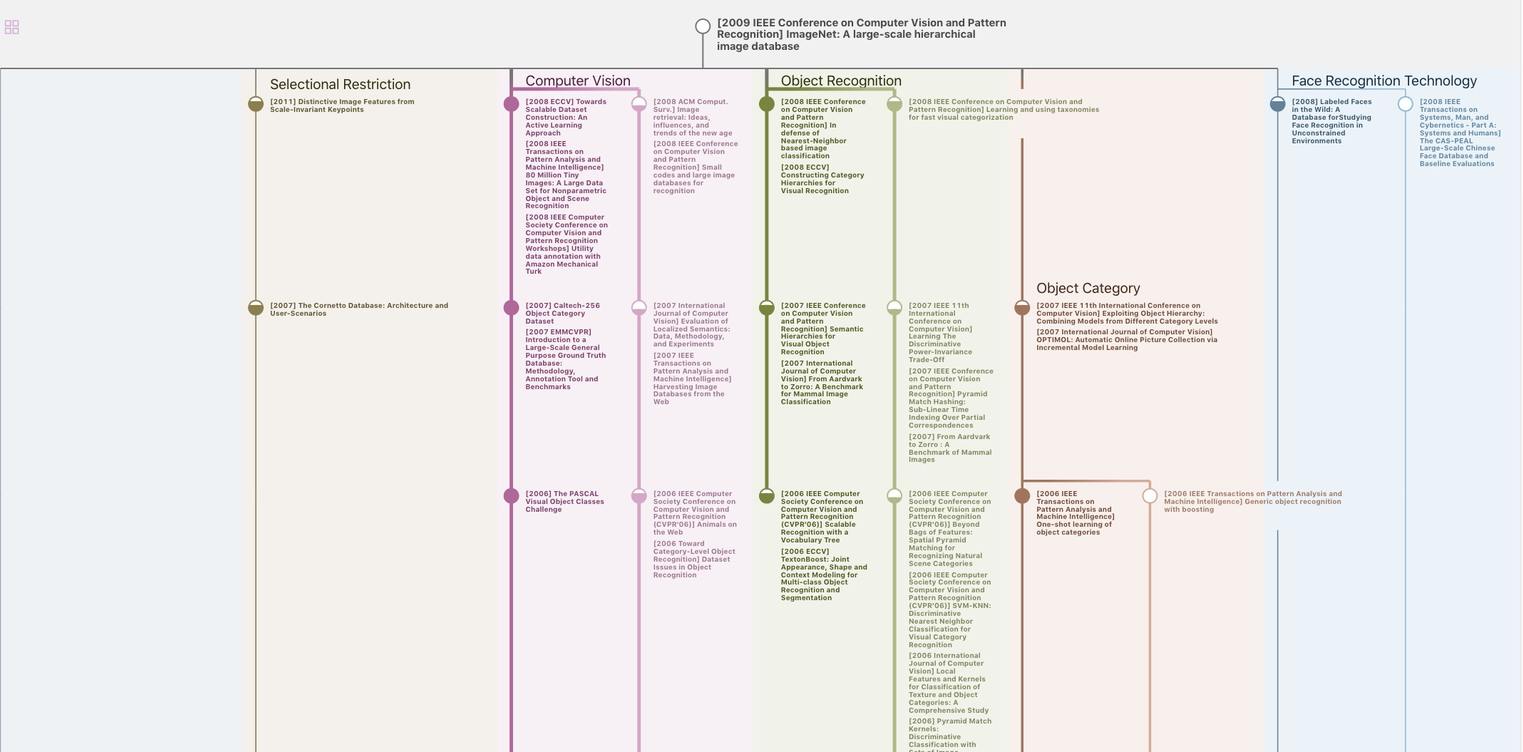
生成溯源树,研究论文发展脉络
Chat Paper
正在生成论文摘要