CaseLink: Inductive Graph Learning for Legal Case Retrieval
arxiv(2024)
摘要
In case law, the precedents are the relevant cases that are used to support
the decisions made by the judges and the opinions of lawyers towards a given
case. This relevance is referred to as the case-to-case reference relation. To
efficiently find relevant cases from a large case pool, retrieval tools are
widely used by legal practitioners. Existing legal case retrieval models mainly
work by comparing the text representations of individual cases. Although they
obtain a decent retrieval accuracy, the intrinsic case connectivity
relationships among cases have not been well exploited for case encoding,
therefore limiting the further improvement of retrieval performance. In a case
pool, there are three types of case connectivity relationships: the case
reference relationship, the case semantic relationship, and the case legal
charge relationship. Due to the inductive manner in the task of legal case
retrieval, using case reference as input is not applicable for testing. Thus,
in this paper, a CaseLink model based on inductive graph learning is proposed
to utilise the intrinsic case connectivity for legal case retrieval, a novel
Global Case Graph is incorporated to represent both the case semantic
relationship and the case legal charge relationship. A novel contrastive
objective with a regularisation on the degree of case nodes is proposed to
leverage the information carried by the case reference relationship to optimise
the model. Extensive experiments have been conducted on two benchmark datasets,
which demonstrate the state-of-the-art performance of CaseLink. The code has
been released on https://github.com/yanran-tang/CaseLink.
更多查看译文
AI 理解论文
溯源树
样例
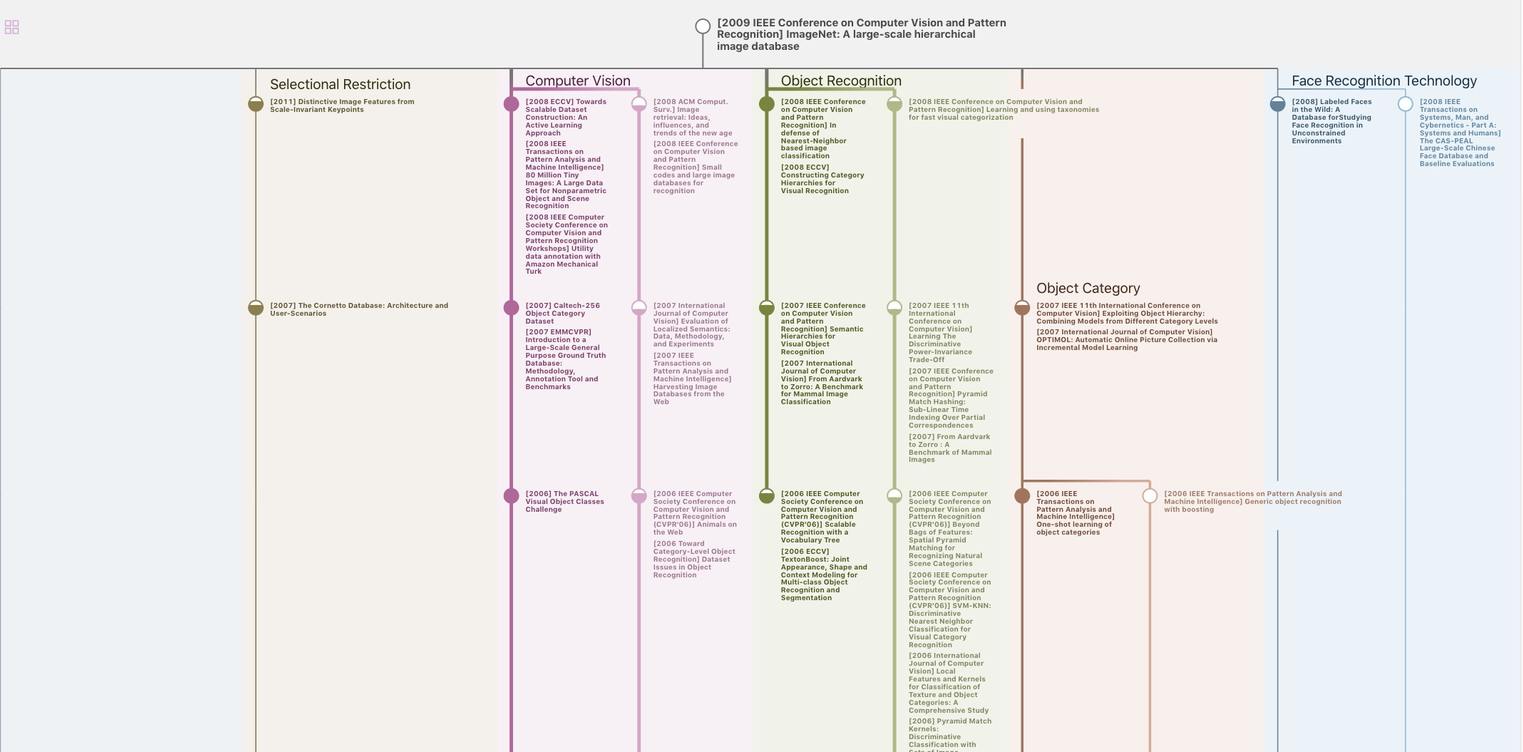
生成溯源树,研究论文发展脉络
Chat Paper
正在生成论文摘要