Analysis on reservoir activation with the nonlinearity harnessed from solution-processed MoS2 devices
CoRR(2024)
Abstract
Reservoir computing is a recurrent neural network that has been applied
across various domains in machine learning. The implementation of reservoir
computing, however, often demands heavy computations for activating the
reservoir. Configuring physical reservoir networks and harnessing the
nonlinearity from the underlying devices for activation is an emergent solution
to address the computational challenge. Herein, we analyze the feasibility of
employing the nonlinearity from solution-processed molybdenum disulfide (MoS2)
devices for reservoir activation. The devices, fabricated using liquid-phase
exfoliated MoS2, exhibit a high-order nonlinearity achieved by Stark modulation
of the MoS2 material. We demonstrate that this nonlinearity can be fitted and
employed as the activation function to facilitate reservoir computing
implementation. Notably, owing to the high-order nonlinearity, the network
exhibits long-term synchronization and robust generalization abilities for
approximating complex dynamical systems. Given the remarkable reservoir
activation capability, coupled with the scalability of the device fabrication,
our findings open the possibility for the physical realization of lightweight,
efficient reservoir computing for, for instance, signal classification, motion
tracking, and pattern recognition of complex time series as well as secure
cryptography. As an example, we show the network can be appointed to generate
chaotic random numbers for secure data encryption.
MoreTranslated text
AI Read Science
Must-Reading Tree
Example
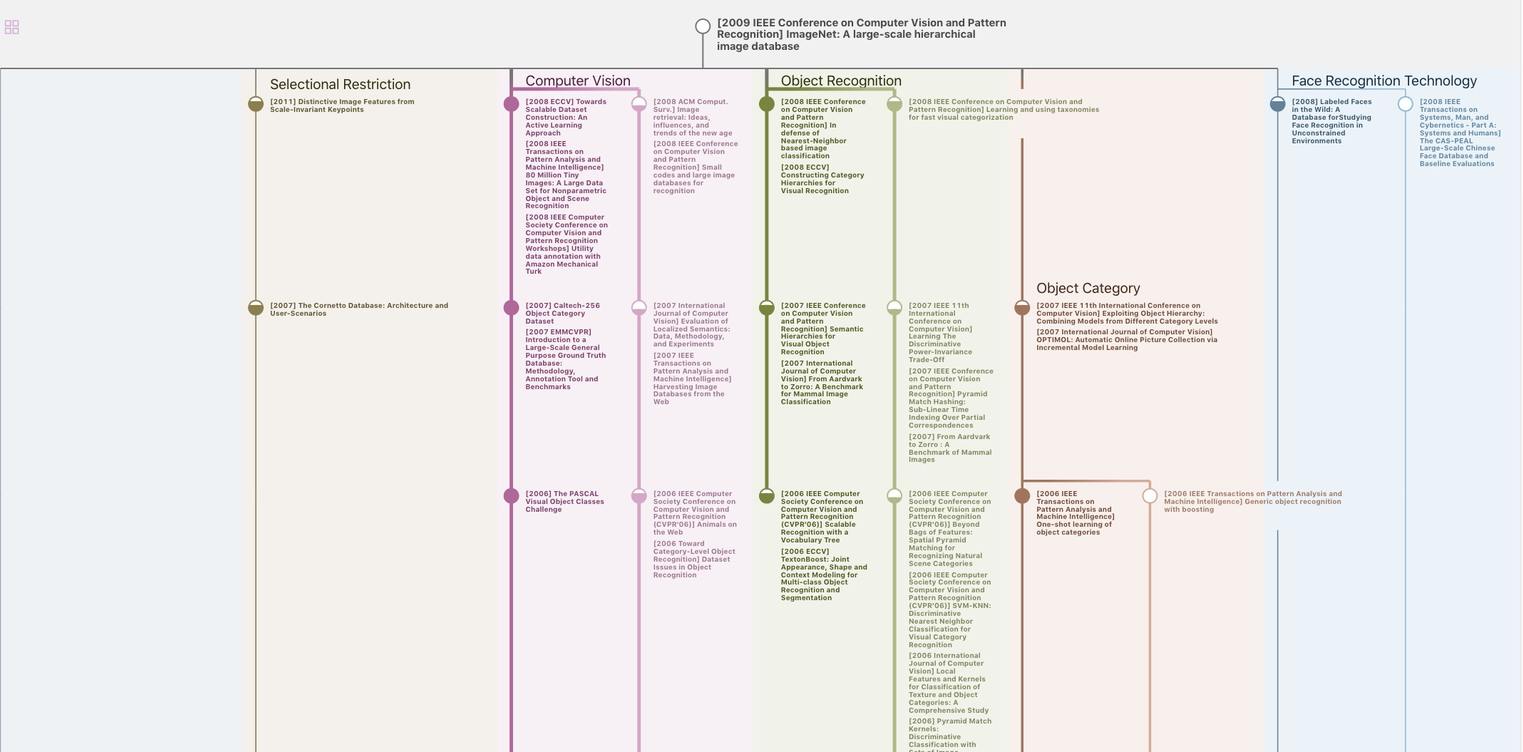
Generate MRT to find the research sequence of this paper
Chat Paper
Summary is being generated by the instructions you defined