AI-Driven Prediction of Sugarcane Quality Attributes Using Satellite Imagery
Sugar Tech(2024)
Abstract
Anticipating the sugar content of sugarcane crop is a crucial aspect that holds the key to develop innovative data-driven solutions for determining the ideal time of mechanized harvest. However, traditional laboratory-based approaches are laborious, time-consuming, and limited in their scalability. Thus, we explored the potential of integrating multispectral data and cutting-edge artificial intelligence algorithms to predict the sugar content attributes of sugarcane, namely Brix and Purity. The sugarcane quality attributes were measured in a routine laboratory using 510 georeferenced samples from two commercial areas. Crop canopy reflectance values and growing degree days (GDD) were used as inputs on developing predictive models. Two artificial intelligence (AI) algorithms, artificial neural network (ANN) and random forest (RF), and multiple linear regression (MLR) were performed to create the predictive models for mapping the sugarcane quality. The models’ performance proved that RF regression was better for °Brix prediction. In contrast, Purity values were better predicted by ANN algorithm. GDD was the most important variable on performance of RF modeling for both outputs, followed by Green spectral band from satellite imagery. Timely results were achieved integrating satellite imagery and AI-based model on prediction of qualitative attributes for sugarcane. It can provide useful data layers to support site-specific management strategies within season by agroindustry and supporting the decision-making of harvesting in large scale.
MoreTranslated text
Key words
Brix,Crop monitoring,Machine learning,Saccharum spp
AI Read Science
Must-Reading Tree
Example
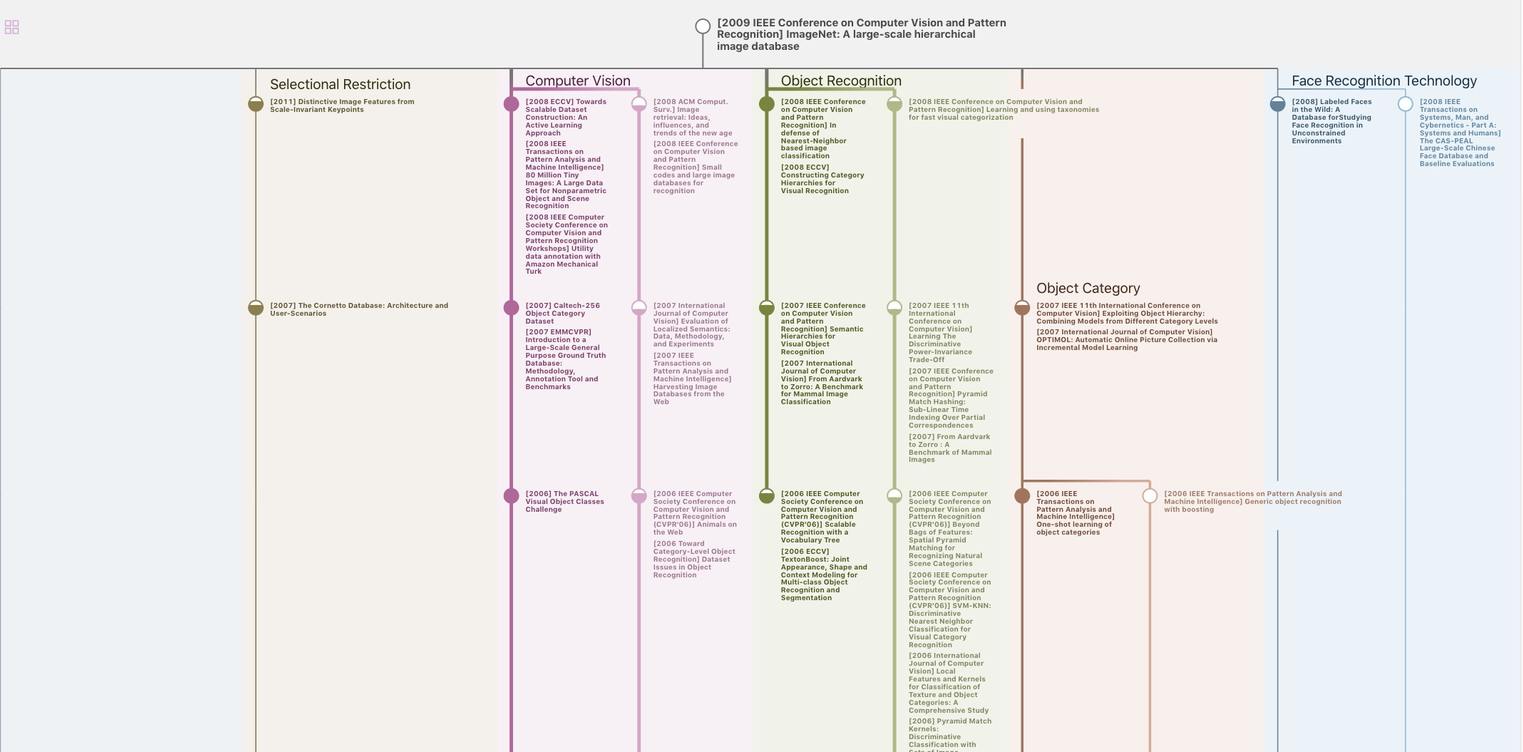
Generate MRT to find the research sequence of this paper
Chat Paper
Summary is being generated by the instructions you defined