High-capacity data hiding for medical images based on the mask-RCNN model
Scientific Reports(2024)
摘要
This study introduces a novel approach for integrating sensitive patient information within medical images with minimal impact on their diagnostic quality. Utilizing the mask region-based convolutional neural network for identifying regions of minimal medical significance, the method embeds information using discrete cosine transform-based steganography. The focus is on embedding within “insignificant areas”, determined by deep learning models, to ensure image quality and confidentiality are maintained. The methodology comprises three main steps: neural network training for area identification, an embedding process for data concealment, and an extraction process for retrieving embedded information. Experimental evaluations on the CHAOS dataset demonstrate the method’s effectiveness, with the model achieving an average intersection over union score of 0.9146, indicating accurate segmentation. Imperceptibility metrics, including peak signal-to-noise ratio, were employed to assess the quality of stego images, with results showing high capacity embedding with minimal distortion. Furthermore, the embedding capacity and payload analysis reveal the method’s high capacity for data concealment. The proposed method outperforms existing techniques by offering superior image quality, as evidenced by higher peak signal-to-noise ratio values, and efficient concealment capacity, making it a promising solution for secure medical image handling.
更多查看译文
关键词
Medical image security,Data hiding techniques,Mask-RCNN,Deep learning in medical imaging,High-capacity embedding,DCT-based steganography
AI 理解论文
溯源树
样例
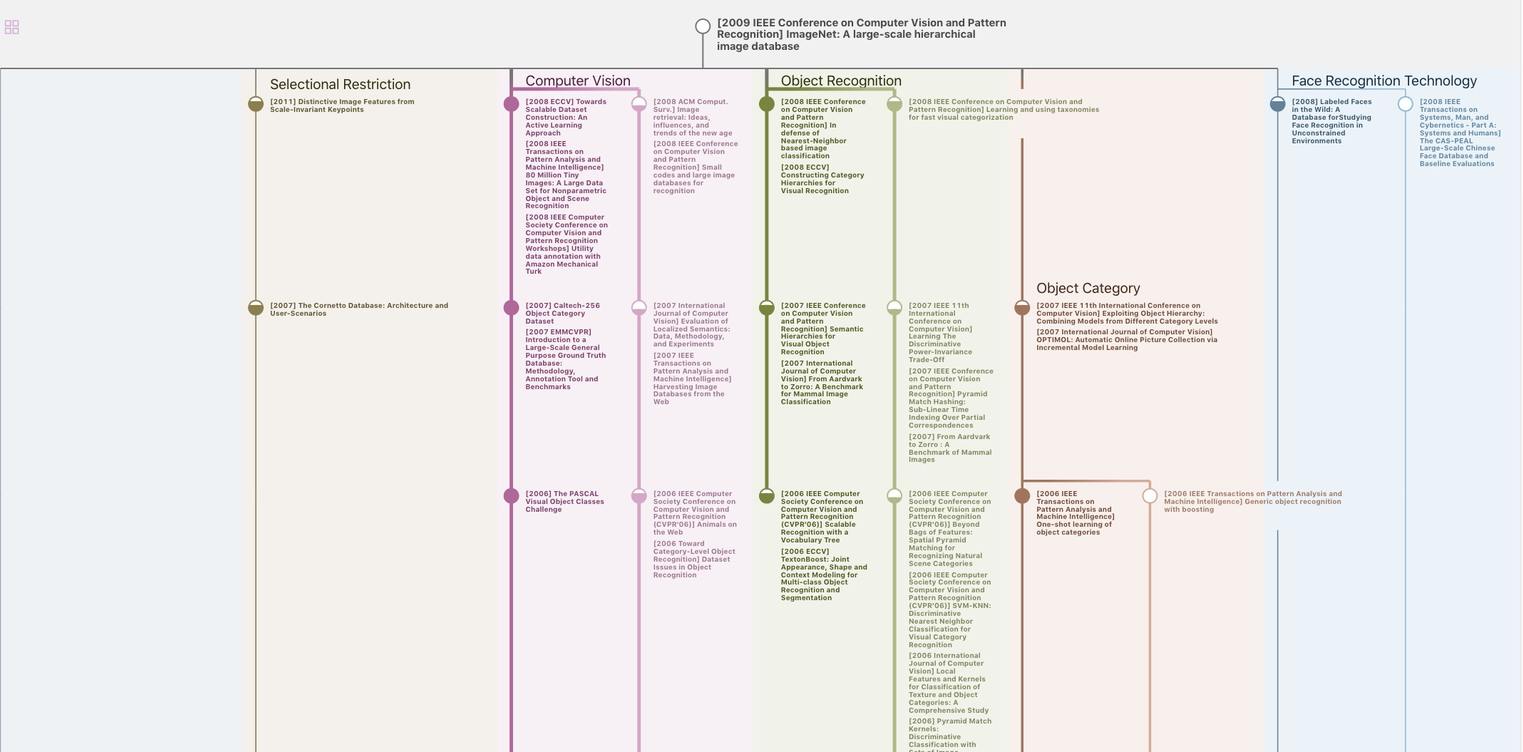
生成溯源树,研究论文发展脉络
Chat Paper
正在生成论文摘要