Enhancing predictive modeling of photovoltaic materials’ solar power conversion efficiency using explainable AI
Energy Reports(2024)
摘要
We present a study on Explainable AI-based prediction of power conversion efficiency (PCE) of organic solar cells, conducted on a dataset of 566 small-molecule organic solar cell materials samples with varying donor and acceptor species combinations. This research uncovers an interesting phenomenon, the first of its kind to be reported, of PCE quantization, where the PCE values increase in steps with the increase in feature values. Our findings have significant implications for the development of efficient organic solar cells, as they provide a better understanding of the factors that influence PCE, and highlight the feature value ranges for which more efficient PCE would be achieved. Our study demonstrates the power of XAI techniques in uncovering hidden patterns in scientific datasets and highlights the importance of interdisciplinary research in the field of materials science.
更多查看译文
关键词
XAI,Quantum phenomenom,Photovoltaic,Machine learning,Power conversion efficiency,SHAP values,Materials science,Explainable AI
AI 理解论文
溯源树
样例
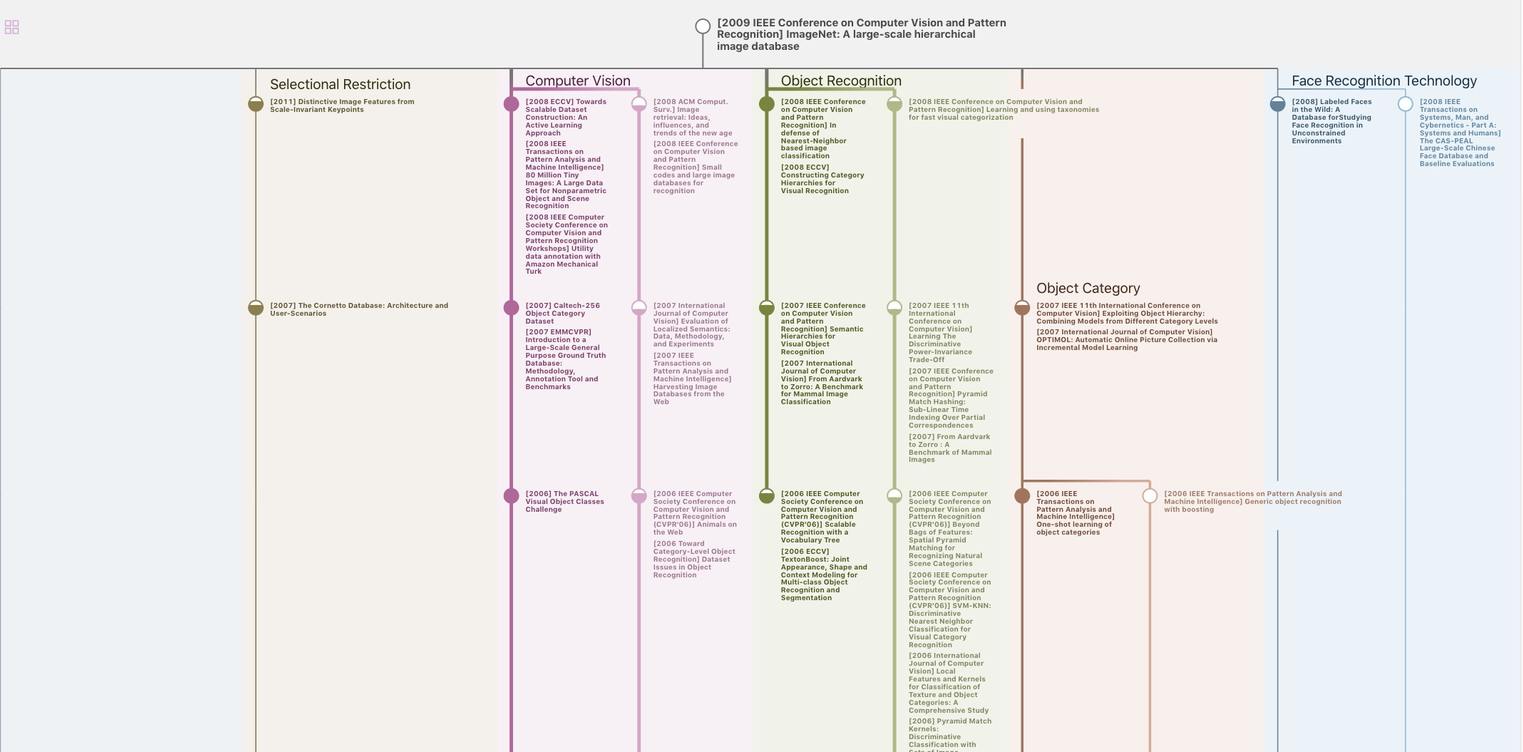
生成溯源树,研究论文发展脉络
Chat Paper
正在生成论文摘要