UMA: Facilitating Backdoor Scanning via Unlearning-Based Model Ablation
AAAI 2024(2024)
摘要
Recent advances in backdoor attacks, like leveraging complex triggers or stealthy implanting techniques, have introduced new challenges in backdoor scanning, limiting the usability of Deep Neural Networks (DNNs) in various scenarios. In this paper, we propose Unlearning-based Model Ablation (UMA), a novel approach to facilitate backdoor scanning and defend against advanced backdoor attacks. UMA filters out backdoor-irrelevant features by ablating the inherent features of the target class within the model and subsequently reveals the backdoor through dynamic trigger optimization. We evaluate our method on 1700 models (700 benign and 1000 trojaned) with 6 model structures, 7 different backdoor attacks and 4 datasets. Our results demonstrate that the proposed methodology effectively detect these advanced backdoors. Specifically, our method can achieve 91% AUC-ROC and 86.6% detection accuracy on average, which outperforms the baselines, including Neural Cleanse, ABS, K-Arm and MNTD.
更多查看译文
关键词
General
AI 理解论文
溯源树
样例
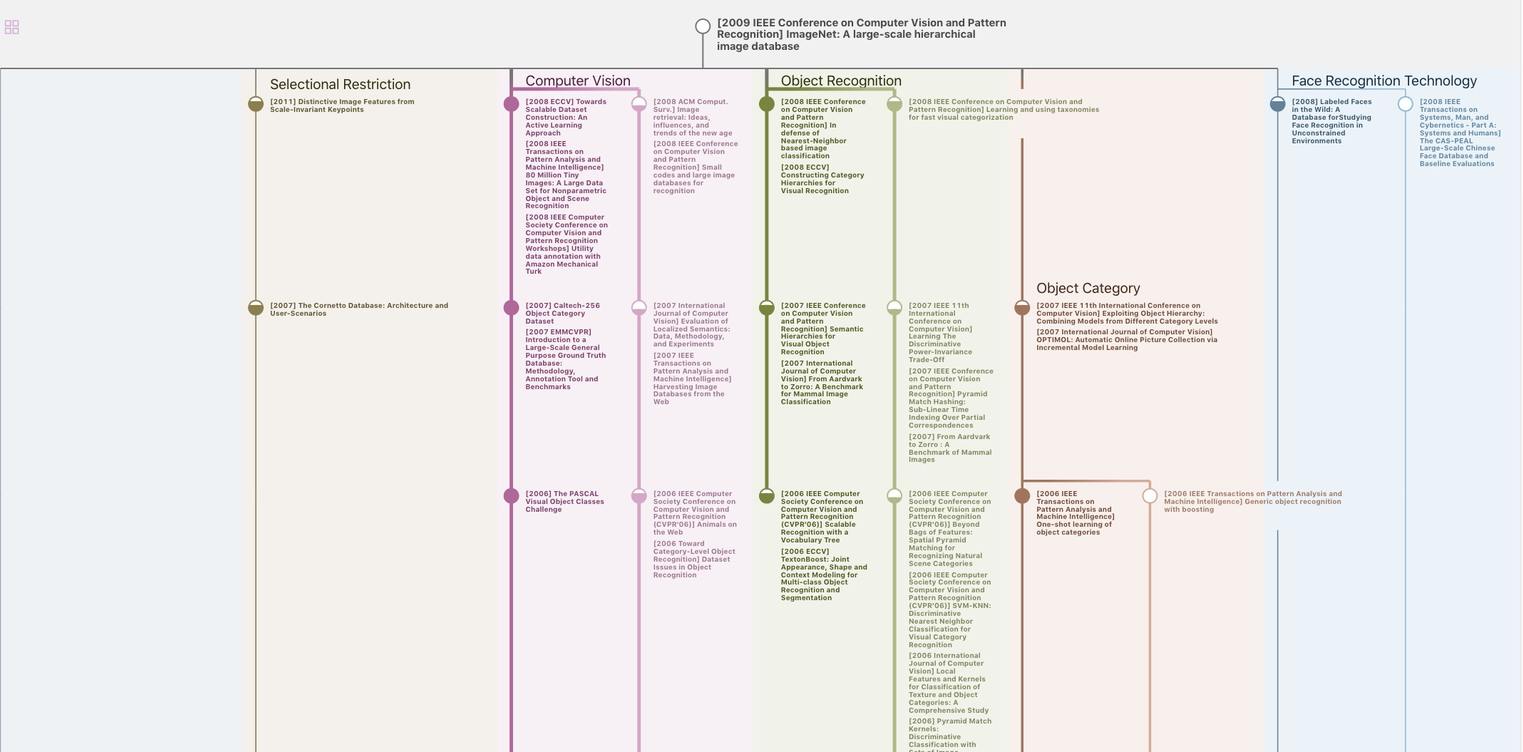
生成溯源树,研究论文发展脉络
Chat Paper
正在生成论文摘要