LaViP: Language-Grounded Visual Prompting
AAAI 2024(2024)
摘要
We introduce a language-grounded visual prompting method to adapt the visual encoder of vision-language models for downstream tasks. By capitalizing on language integration, we devise a parameter-efficient strategy to adjust the input of the visual encoder, eliminating the need to modify or add to the model's parameters. Due to this design choice, our algorithm can operate even in black-box scenarios, showcasing adaptability in situations where access to the model's parameters is constrained. We will empirically demonstrate that, compared to prior art, grounding visual prompts with language enhances both the accuracy and speed of adaptation. Moreover, our algorithm excels in base-to-novel class generalization, overcoming limitations of visual prompting and exhibiting the capacity to generalize beyond seen classes. We thoroughly assess and evaluate our method across a variety of image recognition datasets, such as EuroSAT, UCF101, DTD, and CLEVR, spanning different learning situations, including few-shot adaptation, base-to-novel class generalization, and transfer learning.
更多查看译文
关键词
CV: Multi-modal Vision,CV: Language and Vision,ML: Multimodal Learning
AI 理解论文
溯源树
样例
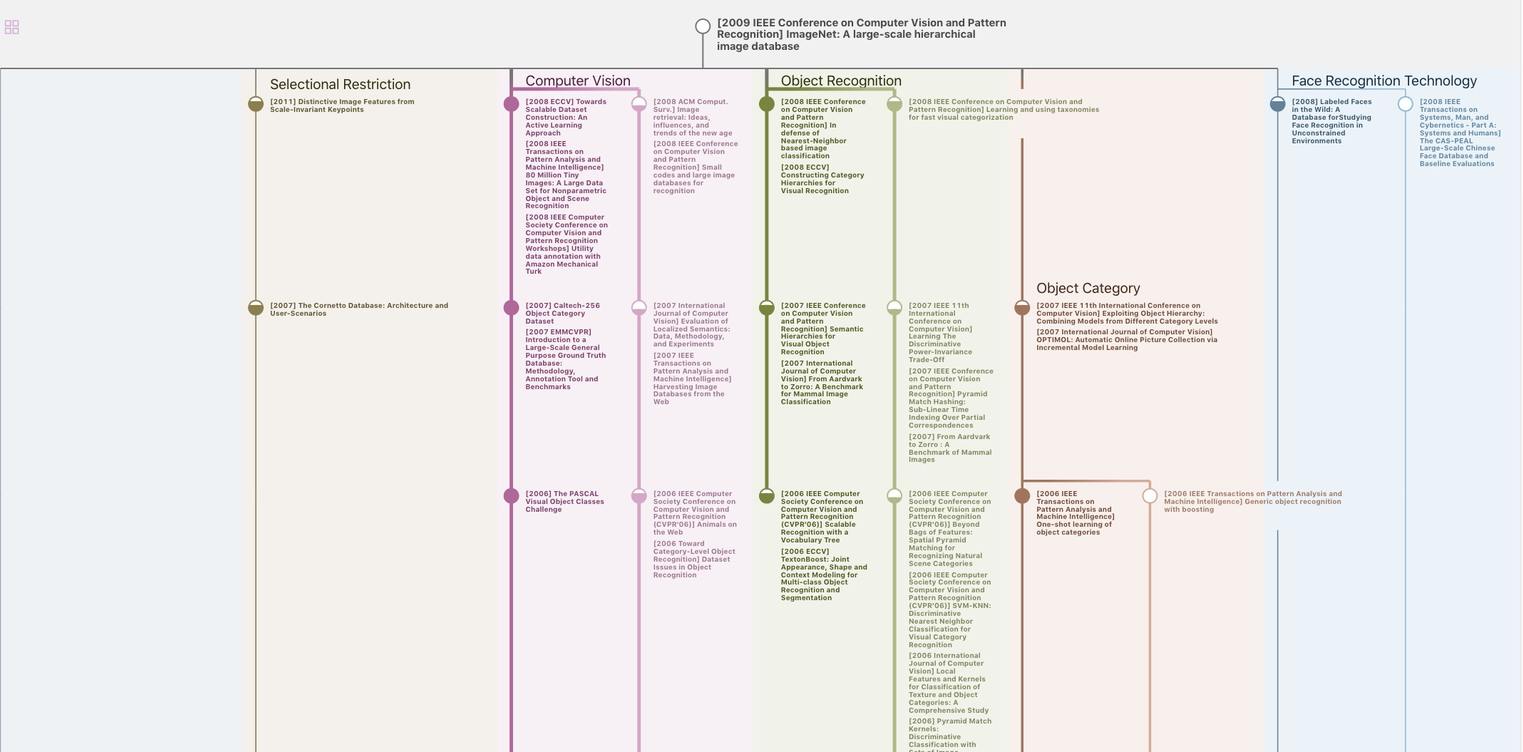
生成溯源树,研究论文发展脉络
Chat Paper
正在生成论文摘要