Embedded Feature Selection on Graph-Based Multi-View Clustering
AAAI 2024(2024)
摘要
Recently, anchor graph-based multi-view clustering has been proven to be highly efficient for large-scale data processing. However, most existing anchor graph-based clustering methods necessitate post-processing to obtain clustering labels and are unable to effectively utilize the information within anchor graphs. To solve these problems, we propose an Embedded Feature Selection on Graph-Based Multi-View Clustering (EFSGMC) approach to improve the clustering performance. Our method decomposes anchor graphs, taking advantage of memory efficiency, to obtain clustering labels in a single step without the need for post-processing. Furthermore, we introduce the l2,p-norm for graph-based feature selection, which selects the most relevant data for efficient graph factorization. Lastly, we employ the tensor Schatten p-norm as a tensor rank approximation function to capture the complementary information between different views, ensuring similarity between cluster assignment matrices. Experimental results on five real-world datasets demonstrate that our proposed method outperforms state-of-the-art approaches.
更多查看译文
关键词
ML: Multi-instance/Multi-view Learning,ML: Clustering,ML: Multi-class/Multi-label Learning & Extreme Classification,ML: Multimodal Learning
AI 理解论文
溯源树
样例
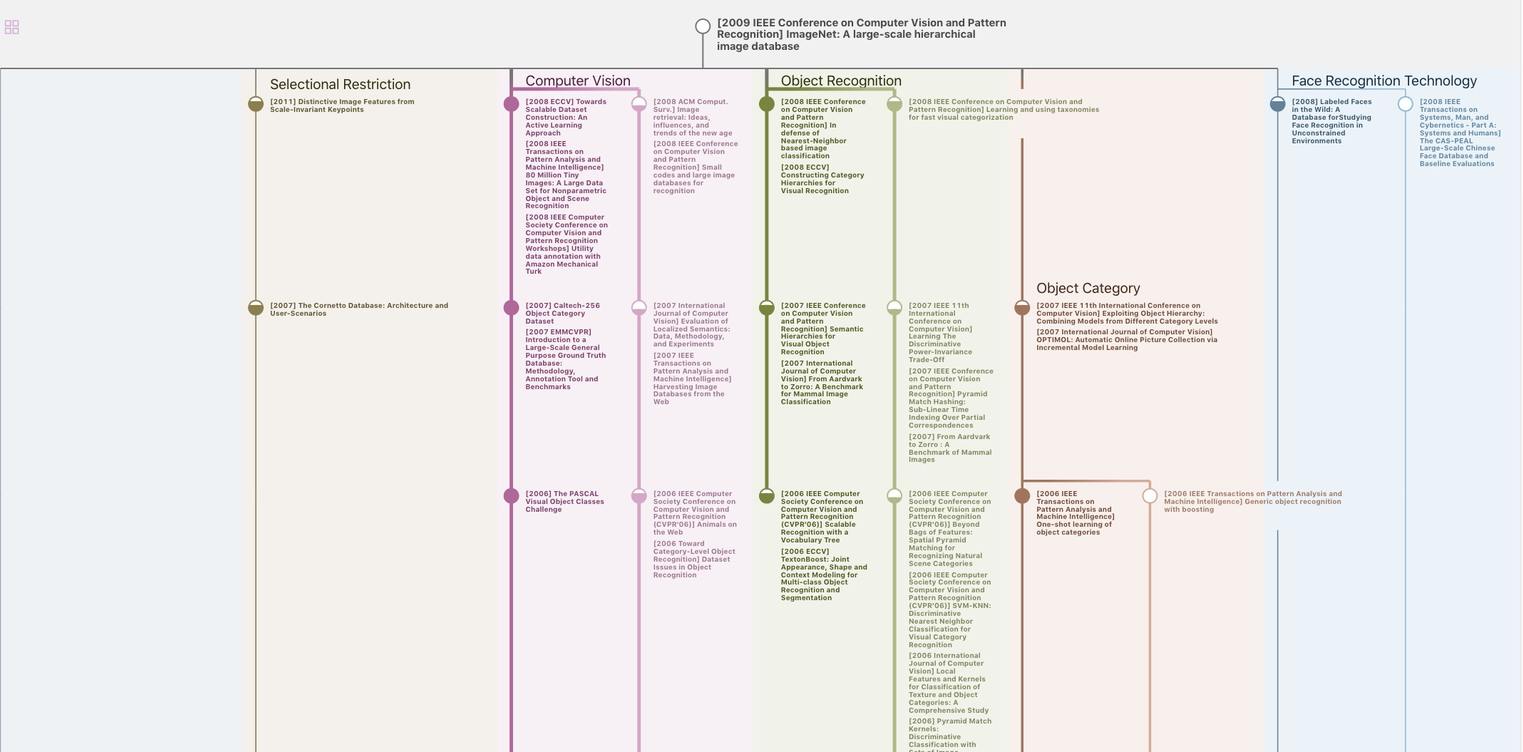
生成溯源树,研究论文发展脉络
Chat Paper
正在生成论文摘要