ZOOM: Learning Video Mirror Detection with Extremely-Weak Supervision
Proceedings of the AAAI Conference on Artificial Intelligence(2024)
摘要
Mirror detection is an active research topic in computer vision. However, all existing mirror detectors learn mirror representations from large-scale pixel-wise datasets, which are tedious and expensive to obtain. Although weakly-supervised learning has been widely explored in related topics, we note that popular weak supervision signals (e.g., bounding boxes, scribbles, points) still require some efforts from the user to locate the target objects, with a strong assumption that the images to annotate always contain the target objects. Such an assumption may result in the over-segmentation of mirrors. Our key idea of this work is that the existence of mirrors over a time period may serve as a weak supervision to train a mirror detector, for two reasons. First, if a network can predict the existence of mirrors, it can essentially locate the mirrors. Second, we observe that the reflected contents of a mirror tend to be similar to those in adjacent frames, but exhibit considerable contrast to regions in far-away frames (e.g., non-mirror frames). To this end, in this paper, we propose ZOOM, the first method to learn robust mirror representations from extremely-weak annotations of per-frame ZerO-One Mirror indicators in videos. The key insight of ZOOM is to model the similarity and contrast (between mirror and non-mirror regions) in temporal variations to locate and segment the mirrors. To this end, we propose a novel fusion strategy to leverage temporal consistency information for mirror localization, and a novel temporal similarity-contrast modeling module for mirror segmentation. We construct a new video mirror dataset for training and evaluation. Experimental results under new and standard metrics show that ZOOM performs favorably against existing fully-supervised mirror detection methods.
更多查看译文
AI 理解论文
溯源树
样例
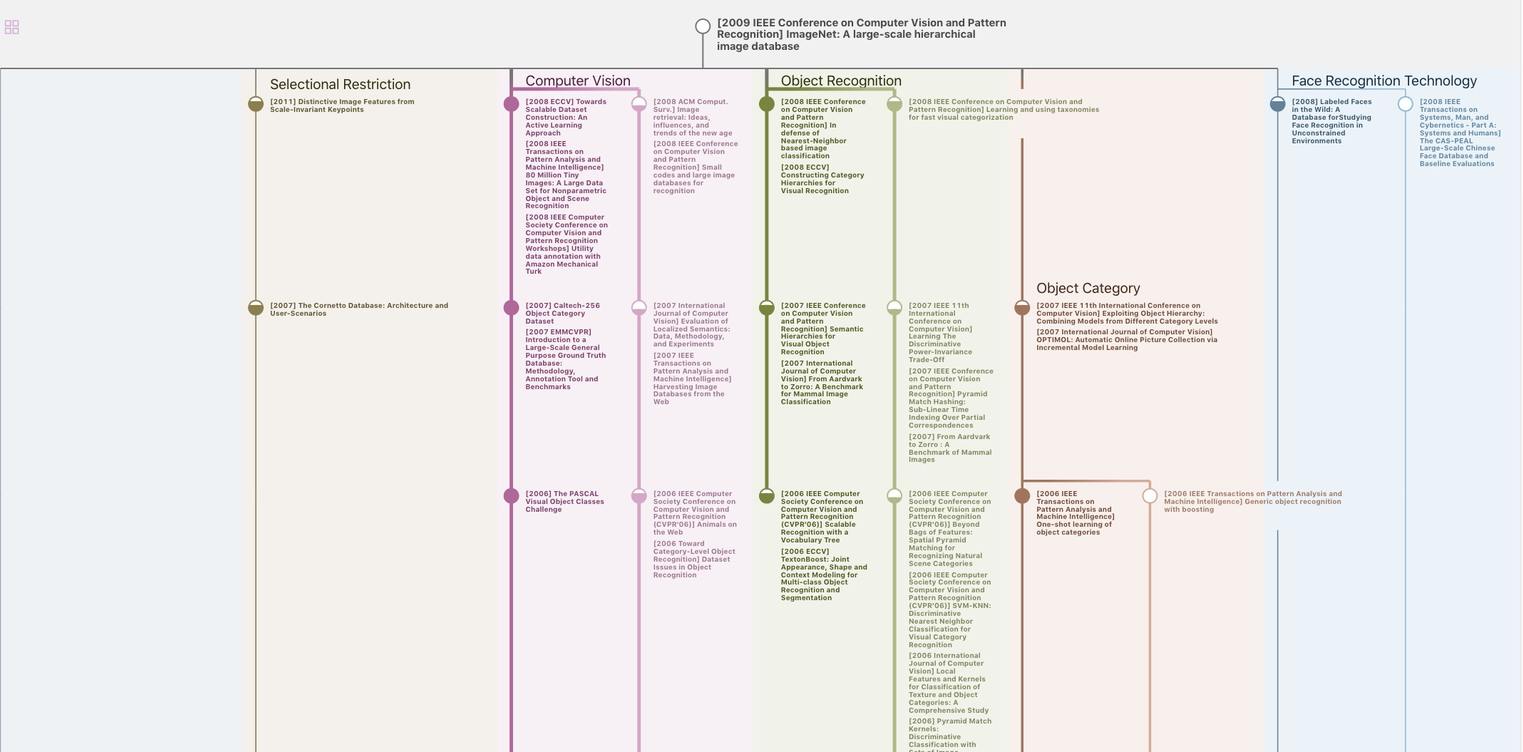
生成溯源树,研究论文发展脉络
Chat Paper
正在生成论文摘要