Mixed-Effects Contextual Bandits
AAAI 2024(2024)
摘要
We study a novel variant of a contextual bandit problem with multi-dimensional reward feedback formulated as a mixed-effects model, where the correlations between multiple feedback are induced by sharing stochastic coefficients called random effects. We propose a novel algorithm, Mixed-Effects Contextual UCB (ME-CUCB), achieving tildeO(d sqrt(mT)) regret bound after T rounds where d is the dimension of contexts and m is the dimension of outcomes, with either known or unknown covariance structure. This is a tighter regret bound than that of the naive canonical linear bandit algorithm ignoring the correlations among rewards. We prove a lower bound of Omega(d sqrt(mT)) matching the upper bound up to logarithmic factors. To our knowledge, this is the first work providing a regret analysis for mixed-effects models and algorithms involving weighted least-squares estimators. Our theoretical analysis faces a significant technical challenge in that the error terms do not constitute martingales since the weights depend on the rewards. We overcome this challenge by using covering numbers, of theoretical interest in its own right. We provide numerical experiments demonstrating the advantage of our proposed algorithm, supporting the theoretical claims.
更多查看译文
关键词
ML: Online Learning & Bandits
AI 理解论文
溯源树
样例
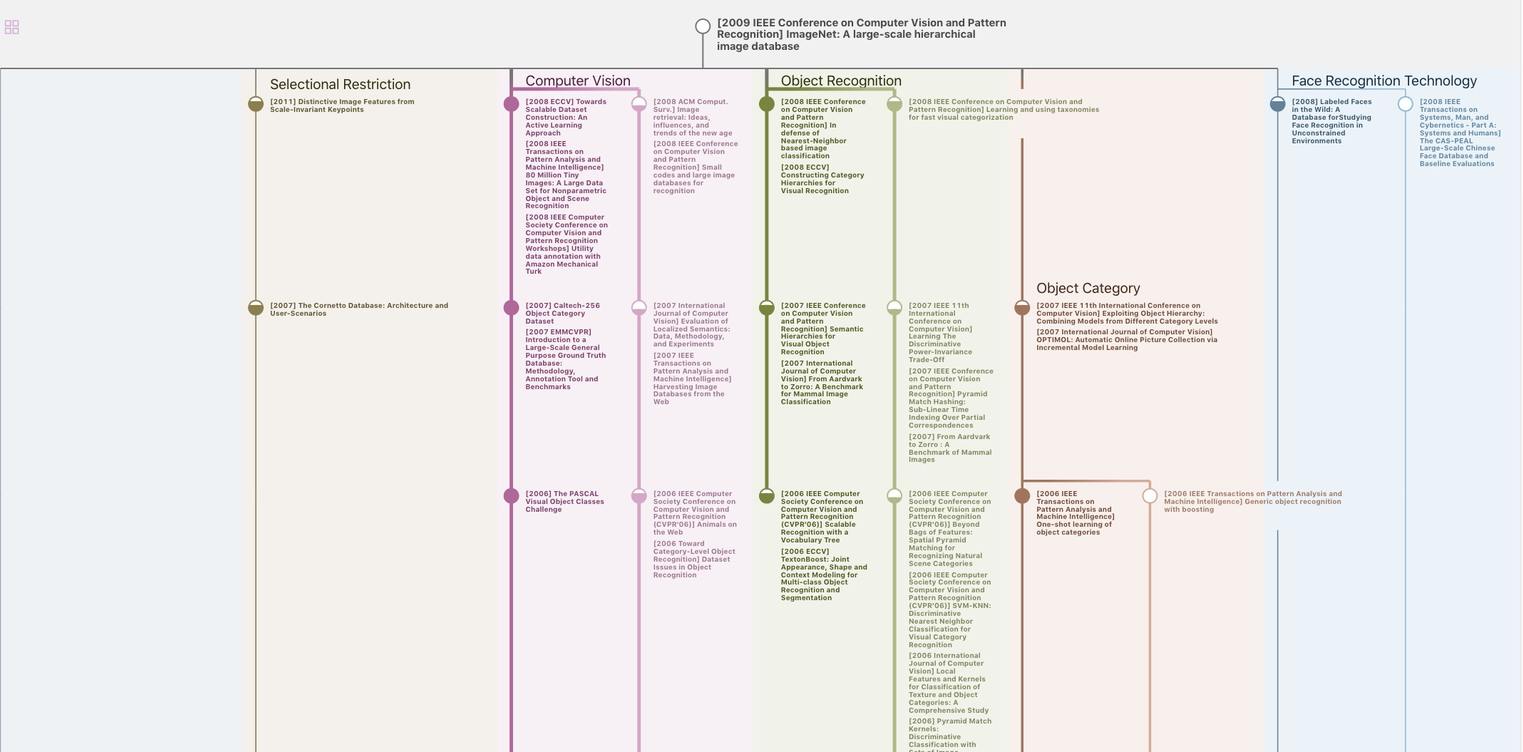
生成溯源树,研究论文发展脉络
Chat Paper
正在生成论文摘要