Data-Driven Discovery of Design Specifications (Student Abstract)
AAAI 2024(2024)
摘要
Ensuring a machine learning model’s trustworthiness is crucial to prevent potential harm. One way to foster trust is through the formal verification of the model’s adherence to essential design requirements. However, this approach relies on well-defined, application-domain-centric criteria with which to test the model, and such specifications may be cumbersome to collect in practice. We propose a data-driven approach for creating specifications to evaluate a trained model effectively. Implementing this framework allows us to prove that the model will exhibit safe behavior while minimizing the false-positive prediction rate. This strategy enhances predictive accuracy and safety, providing deeper insight into the model’s strengths and weaknesses, and promotes trust through a systematic approach.
更多查看译文
关键词
Design Specifications,Formal Verification,Trustworthy,Data-driven
AI 理解论文
溯源树
样例
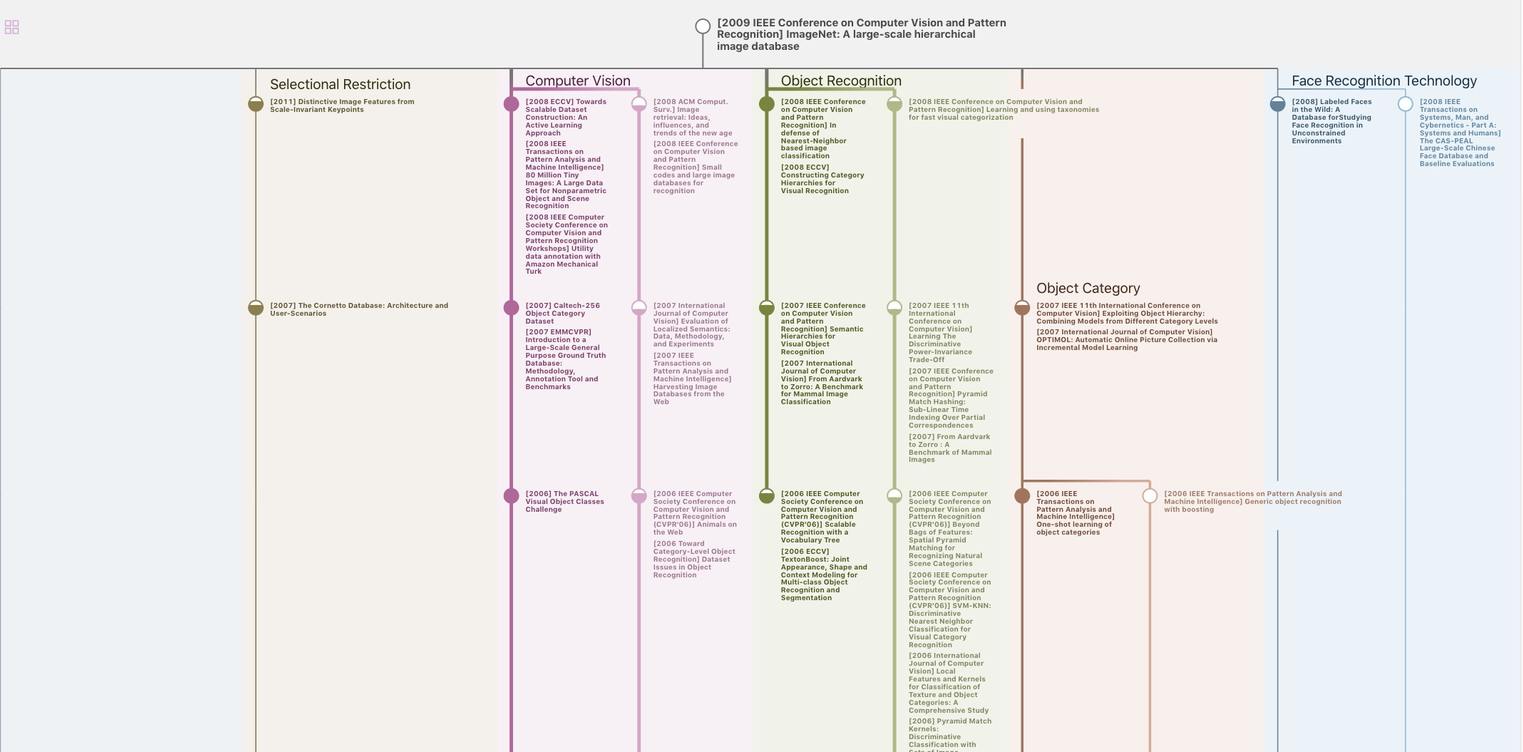
生成溯源树,研究论文发展脉络
Chat Paper
正在生成论文摘要