Probability-Polarized Optimal Transport for Unsupervised Domain Adaptation
AAAI 2024(2024)
摘要
Optimal transport (OT) is an important methodology to measure distribution discrepancy, which has achieved promising performance in artificial intelligence applications, e.g., unsupervised domain adaptation. However, from the view of transportation, there are still limitations: 1) the local discriminative structures for downstream tasks, e.g., cluster structure for classification, cannot be explicitly admitted by the learned OT plan; 2) the entropy regularization induces a dense OT plan with increasing uncertainty. To tackle these issues, we propose a novel Probability-Polarized OT (PPOT) framework, which can characterize the structure of OT plan explicitly. Specifically, the probability polarization mechanism is proposed to guide the optimization direction of OT plan, which generates a clear margin between similar and dissimilar transport pairs and reduces the uncertainty. Further, a dynamic mechanism for margin is developed by incorporating task-related information into the polarization, which directly captures the intra/inter class correspondence for knowledge transportation. A mathematical understanding for PPOT is provided from the view of gradient, which ensures interpretability. Extensive experiments on several datasets validate the effectiveness and empirical efficiency of PPOT.
更多查看译文
关键词
ML: Classification and Regression,ML: Transfer, Domain Adaptation, Multi-Task Learning
AI 理解论文
溯源树
样例
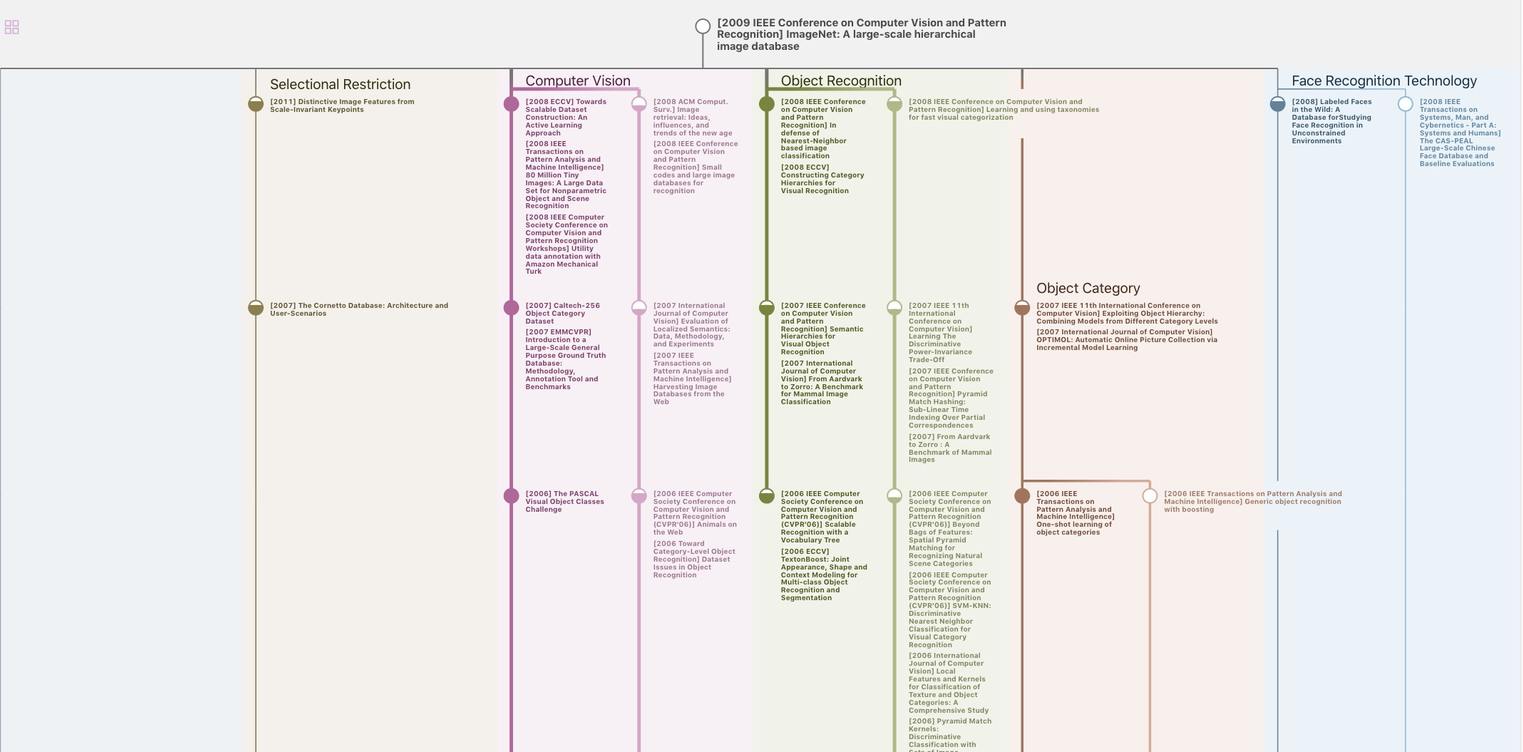
生成溯源树,研究论文发展脉络
Chat Paper
正在生成论文摘要