Energy Efficient Streaming Time Series Classification with Attentive Power Iteration
AAAI 2024(2024)
摘要
Efficiently processing time series data streams in real-time on resource-constrained devices offers significant advantages in terms of enhanced computational energy efficiency and reduced time-related risks. We introduce an innovative streaming time series classification network that utilizes attentive power iteration, enabling real-time processing on resource-constrained devices. Our model continuously updates a compact representation of the entire time series, enhancing classification accuracy while conserving energy and processing time. Notably, it excels in streaming scenarios without requiring complete time series access, enabling swift decisions. Experimental results show that our approach excels in classification accuracy and energy efficiency, with over 70% less consumption and threefold faster task completion than benchmarks. This work advances real-time responsiveness, energy conservation, and operational effectiveness for constrained devices, contributing to optimizing various applications.
更多查看译文
关键词
ML: Time-Series/Data Streams,DMKM: Data Stream Mining,DMKM: Mining of Spatial, Temporal or Spatio-Temporal Data,ML: Deep Learning Algorithms
AI 理解论文
溯源树
样例
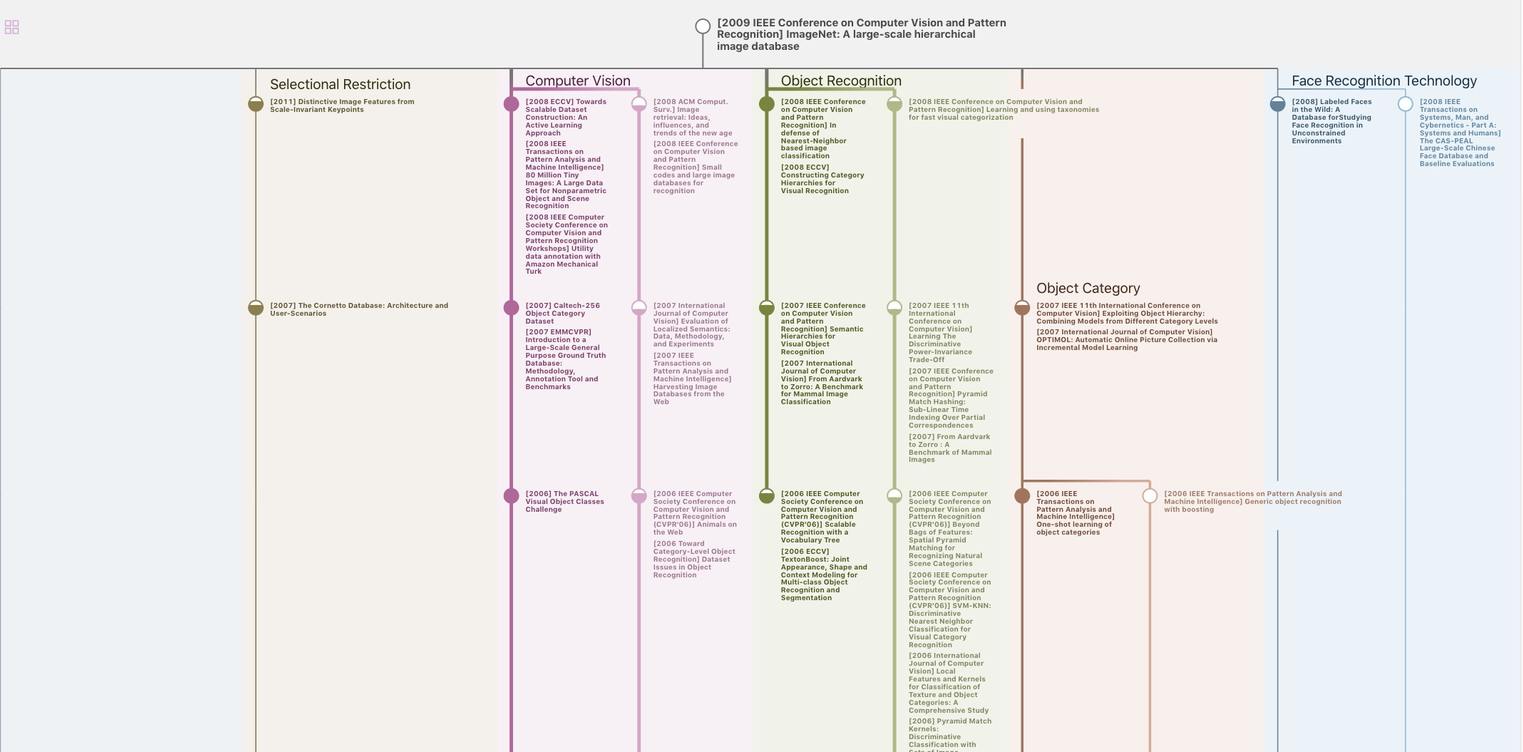
生成溯源树,研究论文发展脉络
Chat Paper
正在生成论文摘要