msLPCC: A Multimodal-Driven Scalable Framework for Deep LiDAR Point Cloud Compression
AAAI 2024(2024)
摘要
LiDAR sensors are widely used in autonomous driving, and the growing storage and transmission demands have made LiDAR point cloud compression (LPCC) a hot research topic. To address the challenges posed by the large-scale and uneven-distribution (spatial and categorical) of LiDAR point data, this paper presents a new multimodal-driven scalable LPCC framework. For the large-scale challenge, we decouple the original LiDAR data into multi-layer point subsets, compress and transmit each layer separately, so as to ensure the reconstruction quality requirement under different scenarios. For the uneven-distribution challenge, we extract, align, and fuse heterologous feature representations, including point modality with position information, depth modality with spatial distance information, and segmentation modality with category information. Extensive experimental results on the benchmark SemanticKITTI database validate that our method outperforms 14 recent representative LPCC methods.
更多查看译文
关键词
CV: Other Foundations of Computer Vision,DMKM: Data Compression
AI 理解论文
溯源树
样例
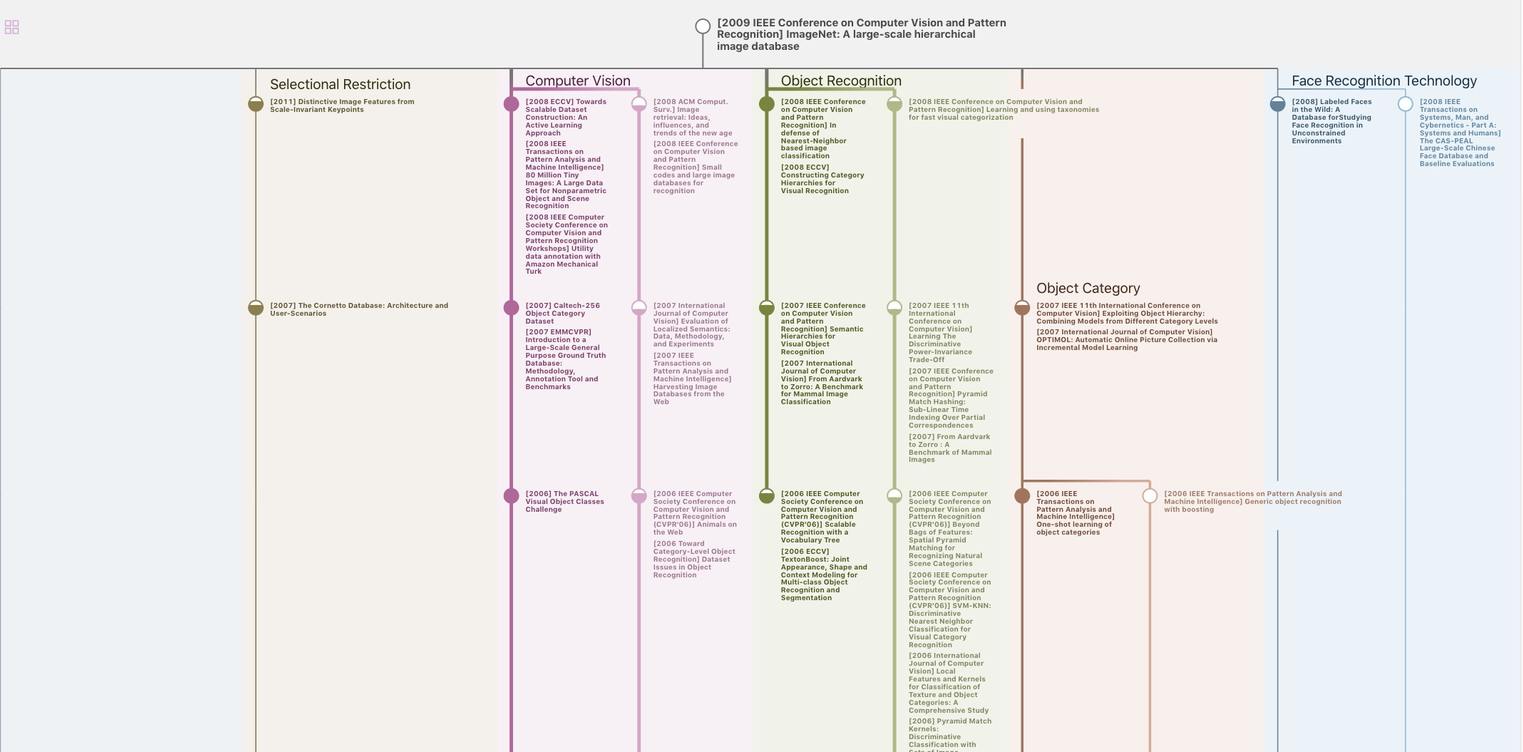
生成溯源树,研究论文发展脉络
Chat Paper
正在生成论文摘要