Towards Fine-Grained HBOE with Rendered Orientation Set and Laplace Smoothing
AAAI 2024(2024)
摘要
Human body orientation estimation (HBOE) aims to estimate the orientation of a human body relative to the camera’s frontal view. Despite recent advancements in this field, there still exist limitations in achieving fine-grained results. We identify certain defects and propose corresponding approaches as follows: 1). Existing datasets suffer from non-uniform angle distributions, resulting in sparse image data for certain angles. To provide comprehensive and high-quality data, we introduce RMOS (Rendered Model Orientation Set), a rendered dataset comprising 150K accurately labeled human instances with a wide range of orientations. 2). Directly using one-hot vector as labels may overlook the similarity between angle labels, leading to poor supervision. And converting the predictions from radians to degrees enlarges the regression error. To enhance supervision, we employ Laplace smoothing to vectorize the label, which contains more information. For fine-grained predictions, we adopt weighted Smooth-L1-loss to align predictions with the smoothed-label, thus providing robust supervision. 3). Previous works ignore body-part-specific information, resulting in coarse predictions. By employing local-window self-attention, our model could utilize different body part information for more precise orientation estimations. We validate the effectiveness of our method in the benchmarks with extensive experiments and show that our method outperforms state-of-the-art. Project is available at: https://github.com/Whalesong-zrs/Towards-Fine-grained-HBOE.
更多查看译文
关键词
CV: Vision for Robotics & Autonomous Driving,CV: Applications
AI 理解论文
溯源树
样例
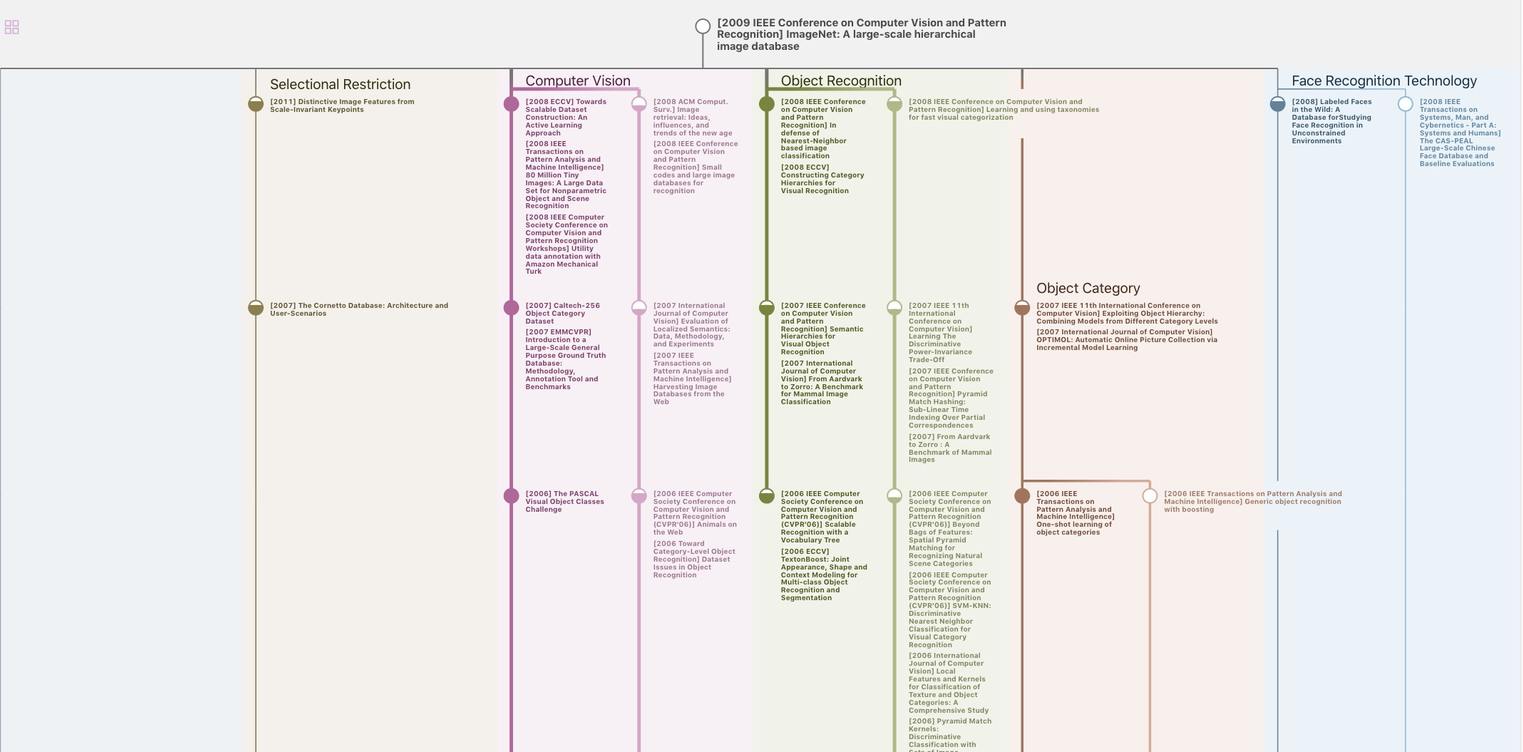
生成溯源树,研究论文发展脉络
Chat Paper
正在生成论文摘要