Toward End-to-End Analog Design Automation with ML and Data-Driven Approaches (Invited Paper)
2024 29th Asia and South Pacific Design Automation Conference (ASP-DAC)(2024)
摘要
Designing analog circuits poses significant challenges due to their knowledge-intensive nature and the diverse range of requirements. There has been limited success in achieving a fully automated framework for designing analog circuits. However, the advent of advanced machine learning algorithms is invigorating design automation efforts by enabling tools to replicate the techniques employed by experienced designers. In this paper, we aim to provide an overview of the recent progress in ML-driven analog circuit sizing and layout automation tool developments. In advanced technology nodes, layout effects must be considered during circuit sizing to avoid costly rerun of the flow. We will discuss the latest research in layout-aware sizing. In the end-to-end analog design automation flow, topology selection plays an important role, as the final performance depends on the choice of topology. We will discuss recent developments in ML-driven topology selection before delving into our vision of an end-to-end data-driven framework that leverages ML techniques to facilitate the selection of optimal topology from a library of topologies.
更多查看译文
关键词
Parallel Design,ML Approaches,Learning Algorithms,Topology Optimization,Analog Circuits,Technology Node,Circuit Size,Advanced Machine Learning Algorithms,Neural Network,Artificial Neural Network,Performance Metrics,Generative Adversarial Networks,Kriging,Design Space,Graph Convolutional Network,Variational Autoencoder,Graph Neural Networks,Gated Recurrent Unit,Design Specifications,Bayesian Optimization,Generative Adversarial Networks Model,Reinforcement Learning Agent,Bayesian Neural Network,Circuit Performance,SPICE Simulations,Circuit Simulation,Reinforcement Learning Framework,Discrete Action,Complex Circuits,Simulation-based Methods
AI 理解论文
溯源树
样例
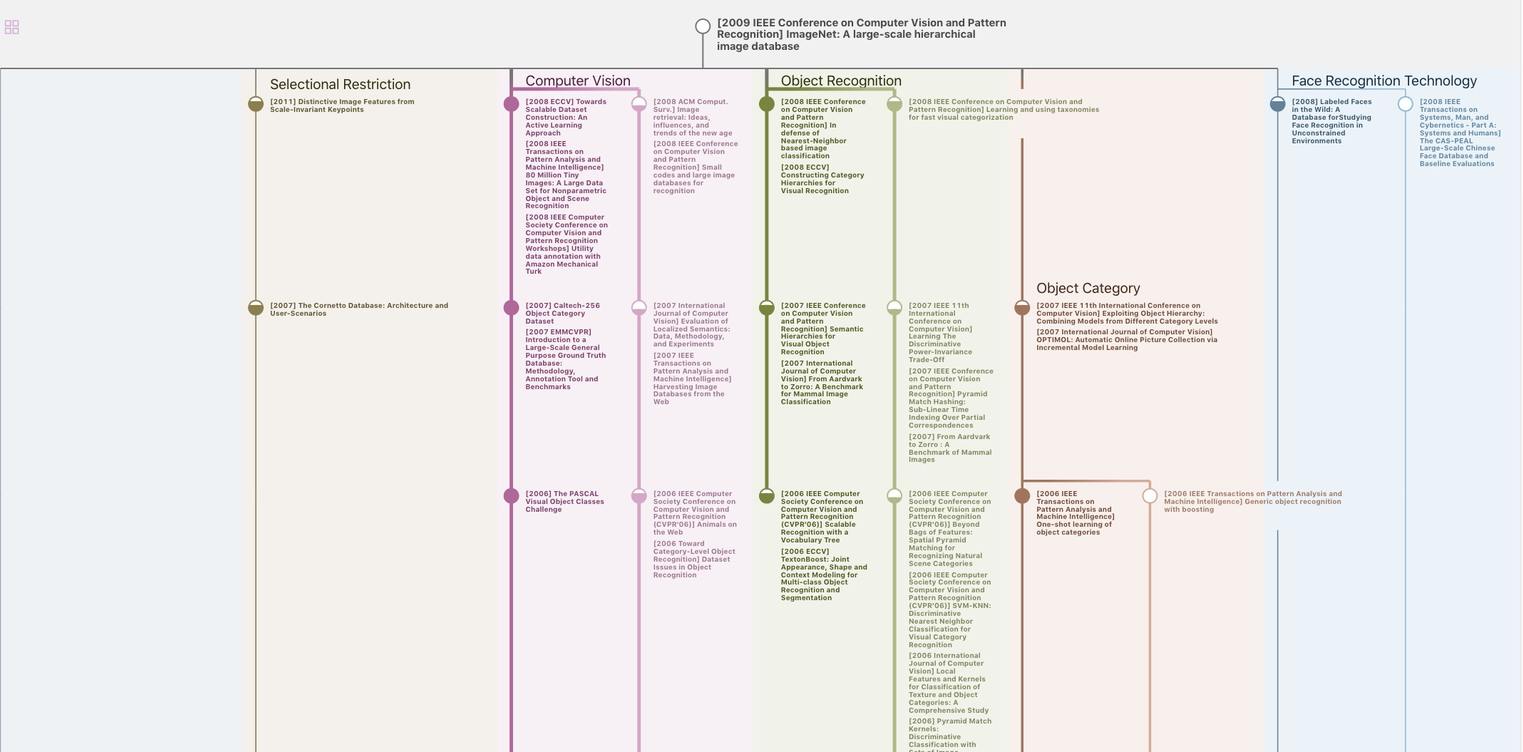
生成溯源树,研究论文发展脉络
Chat Paper
正在生成论文摘要