Diversity-Authenticity Co-constrained Stylization for Federated Domain Generalization in Person Re-identification
AAAI 2024(2024)
摘要
This paper tackles the problem of federated domain generalization in person re-identification (FedDG re-ID), aiming to learn a model generalizable to unseen domains with decentralized source domains. Previous methods mainly focus on preventing local overfitting. However, the direction of diversifying local data through stylization for model training is largely overlooked. This direction is popular in domain generalization but will encounter two issues under federated scenario: (1) Most stylization methods require the centralization of multiple domains to generate novel styles but this is not applicable under decentralized constraint. (2) The authenticity of generated data cannot be ensured especially given limited local data, which may impair the model optimization. To solve these two problems, we propose the Diversity-Authenticity Co-constrained Stylization (DACS), which can generate diverse and authentic data for learning robust local model. Specifically, we deploy a style transformation model on each domain to generate novel data with two constraints: (1) A diversity constraint is designed to increase data diversity, which enlarges the Wasserstein distance between the original and transformed data; (2) An authenticity constraint is proposed to ensure data authenticity, which enforces the transformed data to be easily/hardly recognized by the local-side global/local model. Extensive experiments demonstrate the effectiveness of the proposed DACS and show that DACS achieves state-of-the-art performance for FedDG re-ID.
更多查看译文
关键词
CV: Image and Video Retrieval,CV: Object Detection & Categorization
AI 理解论文
溯源树
样例
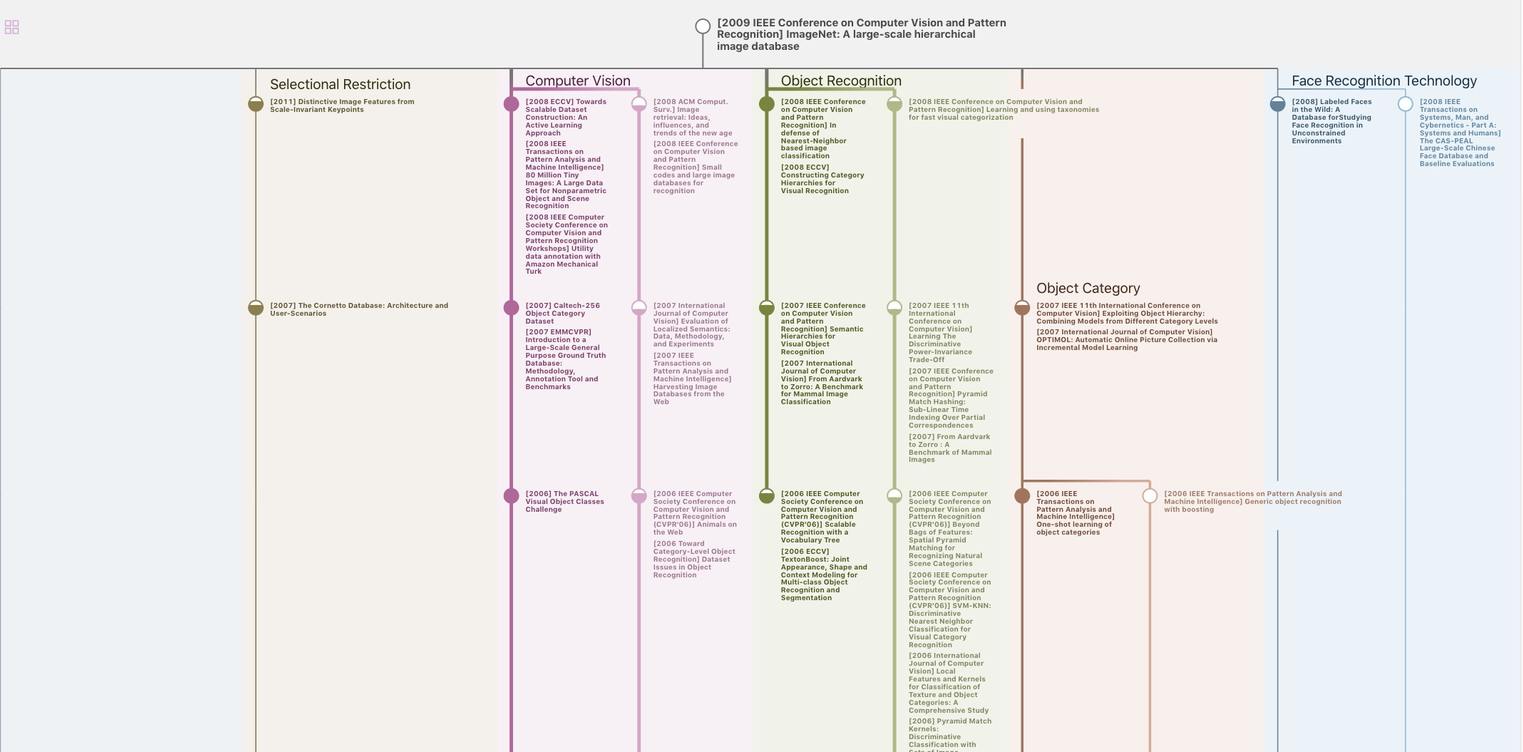
生成溯源树,研究论文发展脉络
Chat Paper
正在生成论文摘要