When CEGAR Meets Regression: A Love Story in Optimal Classical Planning
AAAI 2024(2024)
摘要
Counterexample-Guided Abstraction Refinement (CEGAR) is a prominent technique to generate Cartesian abstractions for guiding search in cost- optimal planning. The core idea is to iteratively refine the abstraction, finding a flaw of the current optimal abstract plan. All existing approaches find these flaws by executing the abstract plan using progression in the original state space.
Instead, we propose to do backward refinements by using regression from the goals. This results in a new type of flaw, that can identify invalid plan suffixes. The resulting abstractions are less focused on the initial state, but more informative on average, significantly improving the performance of current CEGAR-based techniques. Furthermore, they can be combined with forward refinements in several bidirectional strategies that provide the benefits of both methods.
更多查看译文
关键词
PRS: Deterministic Planning,SO: Heuristic Search
AI 理解论文
溯源树
样例
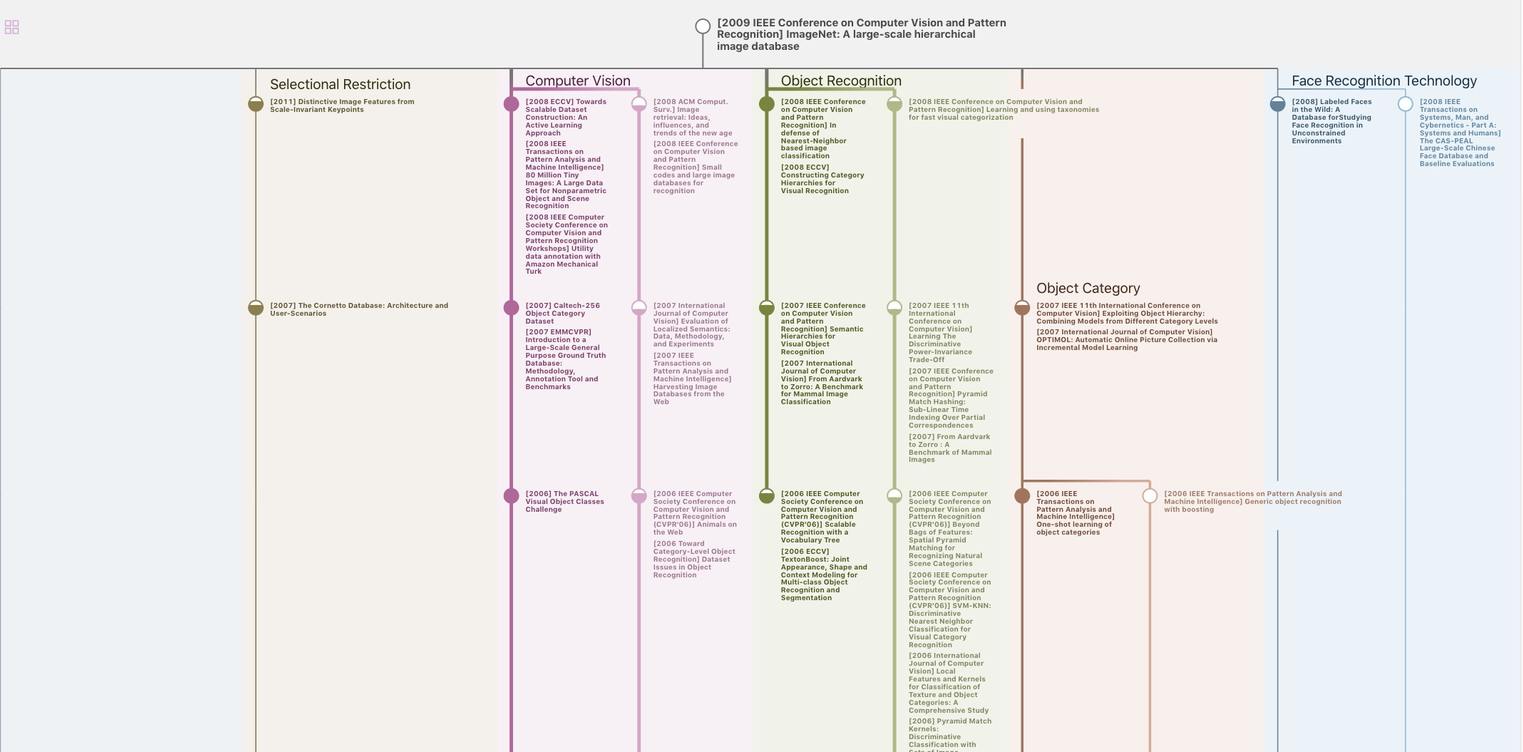
生成溯源树,研究论文发展脉络
Chat Paper
正在生成论文摘要