Short-Term Load Forecasting Method Based on Autoencoder and LSTNet Models
2024 IEEE 4th International Conference on Power, Electronics and Computer Applications (ICPECA)(2024)
摘要
The accuracy of load forecasting is very important to the safe and economical operation of power system. This paper presents a LSTNet model based on Autoencoder feature extraction for load time series prediction. In this model, the Autoencoder extracts the key features of the electrical load time series data by minimizing the reconstruction error, encodes these features into a lower-dimensional vector, and inputs the features into the LSTNet model after dimensionality reduction for network training and prediction. The experimental results show that the Autoencoder-based LSTNet model has more advantages than the traditional LSTNet model and the long short-term memory (LSTM) neural network model in the Tianjin electric load forecasting task, and the prediction accuracy is increased by about 1.5% in the experimental case. This study provides a new method for power system load forecasting and demonstrates the potential of Autoencoders and LSTNet models.
更多查看译文
关键词
electric load forecasting,Deep learning,LSTNet,Autoencoder
AI 理解论文
溯源树
样例
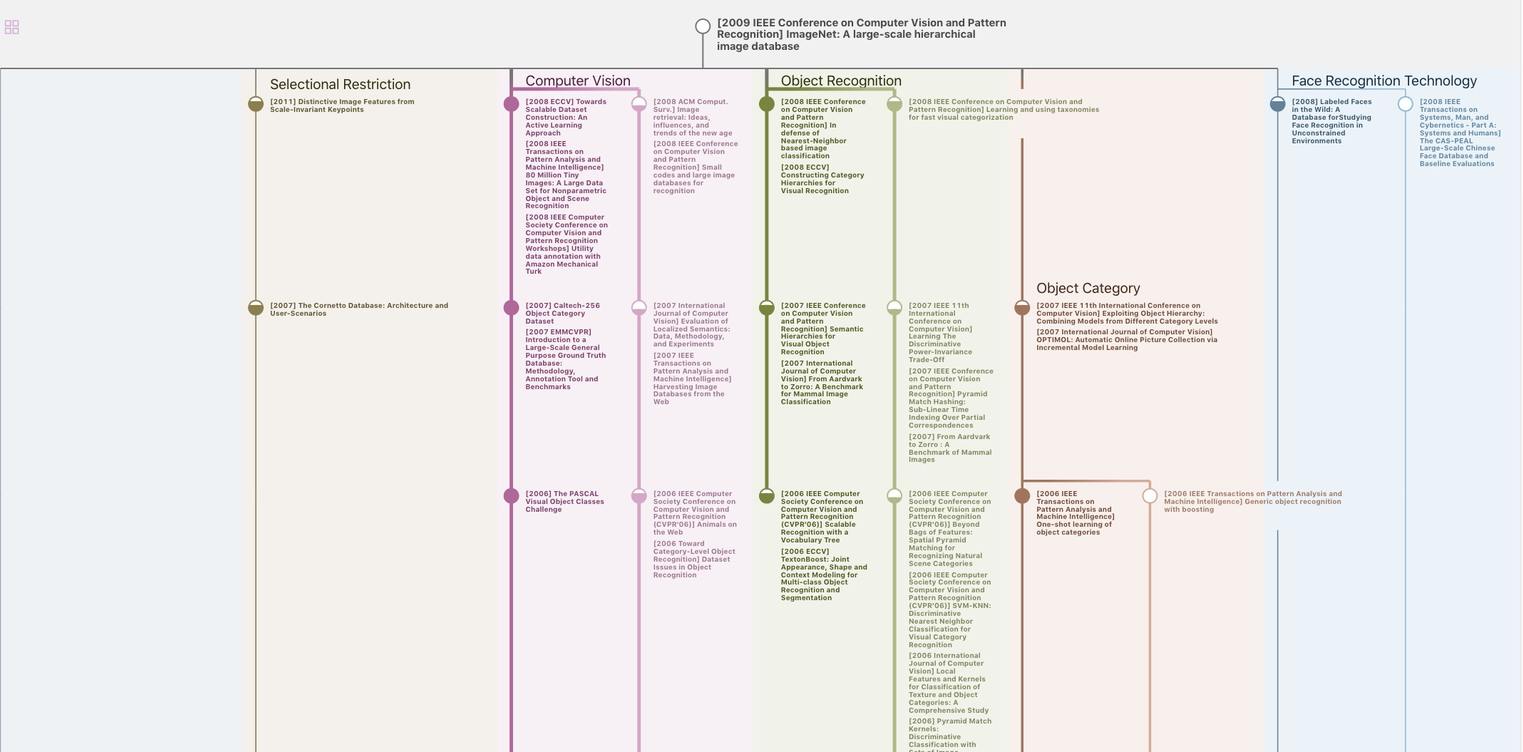
生成溯源树,研究论文发展脉络
Chat Paper
正在生成论文摘要