Spatio-Temporal Pivotal Graph Neural Networks for Traffic Flow Forecasting
AAAI 2024(2024)
摘要
Traffic flow forecasting is a classical spatio-temporal data mining problem with many real-world applications. Recently, various methods based on Graph Neural Networks (GNN) have been proposed for the problem and achieved impressive prediction performance. However, we argue that the majority of existing methods disregarding the importance of certain nodes (referred to as pivotal nodes) that naturally exhibit extensive connections with multiple other nodes. Predicting on pivotal nodes poses a challenge due to their complex spatio-temporal dependencies compared to other nodes. In this paper, we propose a novel GNN-based method called Spatio-Temporal Pivotal Graph Neural Networks (STPGNN) to address the above limitation. We introduce a pivotal node identification module for identifying pivotal nodes. We propose a novel pivotal graph convolution module, enabling precise capture of spatio-temporal dependencies centered around pivotal nodes. Moreover, we propose a parallel framework capable of extracting spatio-temporal traffic features on both pivotal and non-pivotal nodes. Experiments on seven real-world traffic datasets verify our proposed method's effectiveness and efficiency compared to state-of-the-art baselines.
更多查看译文
关键词
DMKM: Mining of Spatial, Temporal or Spatio-Temporal Data
AI 理解论文
溯源树
样例
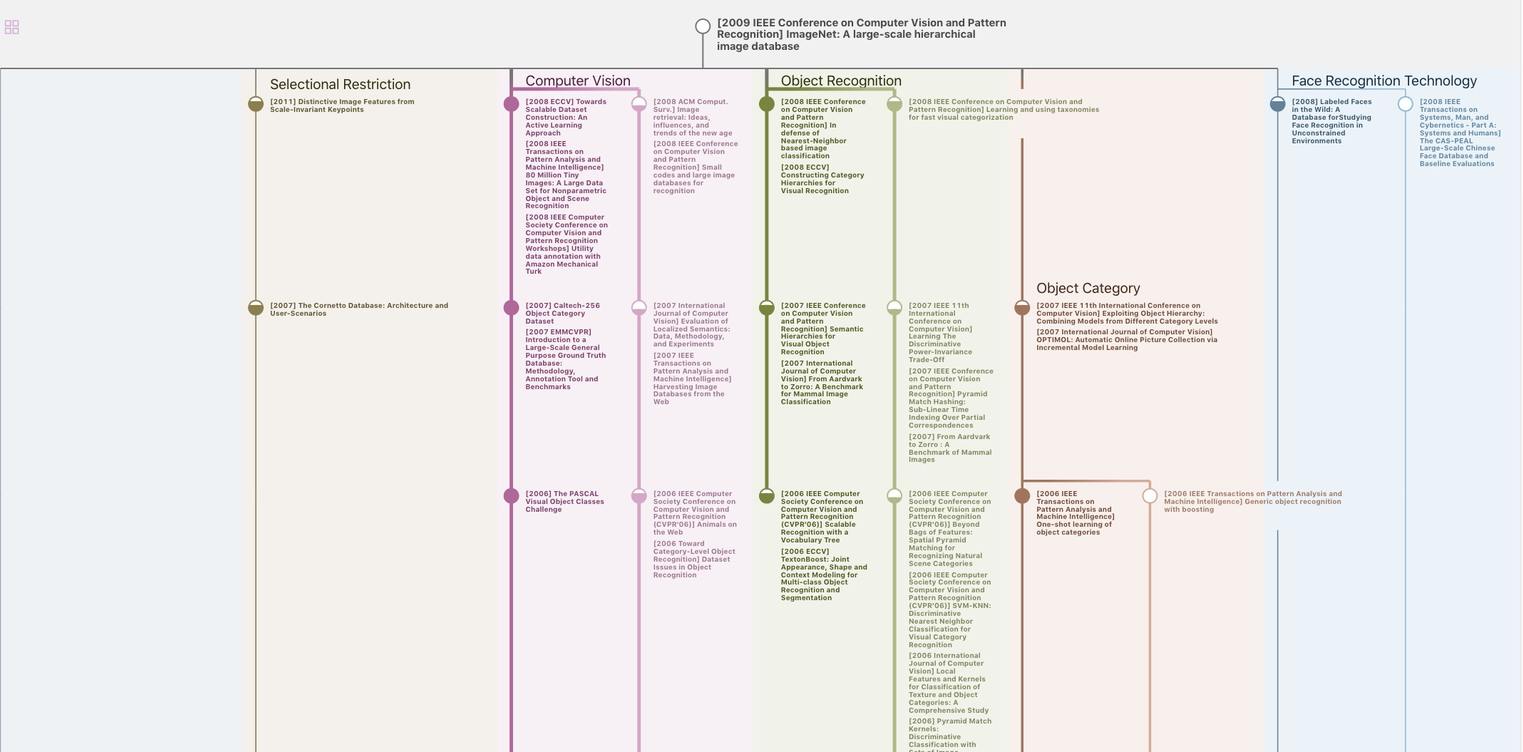
生成溯源树,研究论文发展脉络
Chat Paper
正在生成论文摘要