Robust Test-Time Adaptation for Zero-Shot Prompt Tuning
AAAI 2024(2024)
摘要
CLIP has demonstrated remarkable generalization across diverse downstream tasks. By aligning images and texts in a shared feature space, they enable zero-shot classification via hand-crafted prompts. However, recent studies have shown that hand-crafted prompts may be unsuitable in practical applications. Specifically, choosing an appropriate prompt for a given task requires accurate data and knowledge, which may not be obtainable in practical situations. An inappropriate prompt can result in poor performance. Moreover, if there is no training data, tuning prompts arbitrarily through unlabeled test data may lead to serious performance degradation when giving hand-crafted prompts. Our study reveals that the aforementioned problems are mainly due to the biases in testing data (Data Bias) and pre-trained CLIP model (Model Bias). The Data Bias makes it challenging to choose an appropriate prompt, while Model Bias renders some predictions inaccurate and biased, which leads to error accumulation. To address these biases, we propose robust test-time Adaptation for zeroshot Prompt tuning (ADAPROMPT). Specifically, we ensemble multiple prompts to avoid the worst-case results and dynamically tune prompts to adapt to Data Bias during testing. Furthermore, we adopt a confidence-aware buffer to store balanced and confident unlabeled test data to tune prompts in order to overcome Model Bias. Our extensive experiments on several benchmarks demonstrate that ADAPROMPT alleviates model bias, adapts to data bias and mostly outperforms the state-of-the-art methods at a small time cost. Moreover, our experimental results reveal that ADAPROMPT hardly encounters any performance degradation on these datasets.
更多查看译文
关键词
ML: Time-Series/Data Streams,ML: Unsupervised & Self-Supervised Learning,ML: Semi-Supervised Learning
AI 理解论文
溯源树
样例
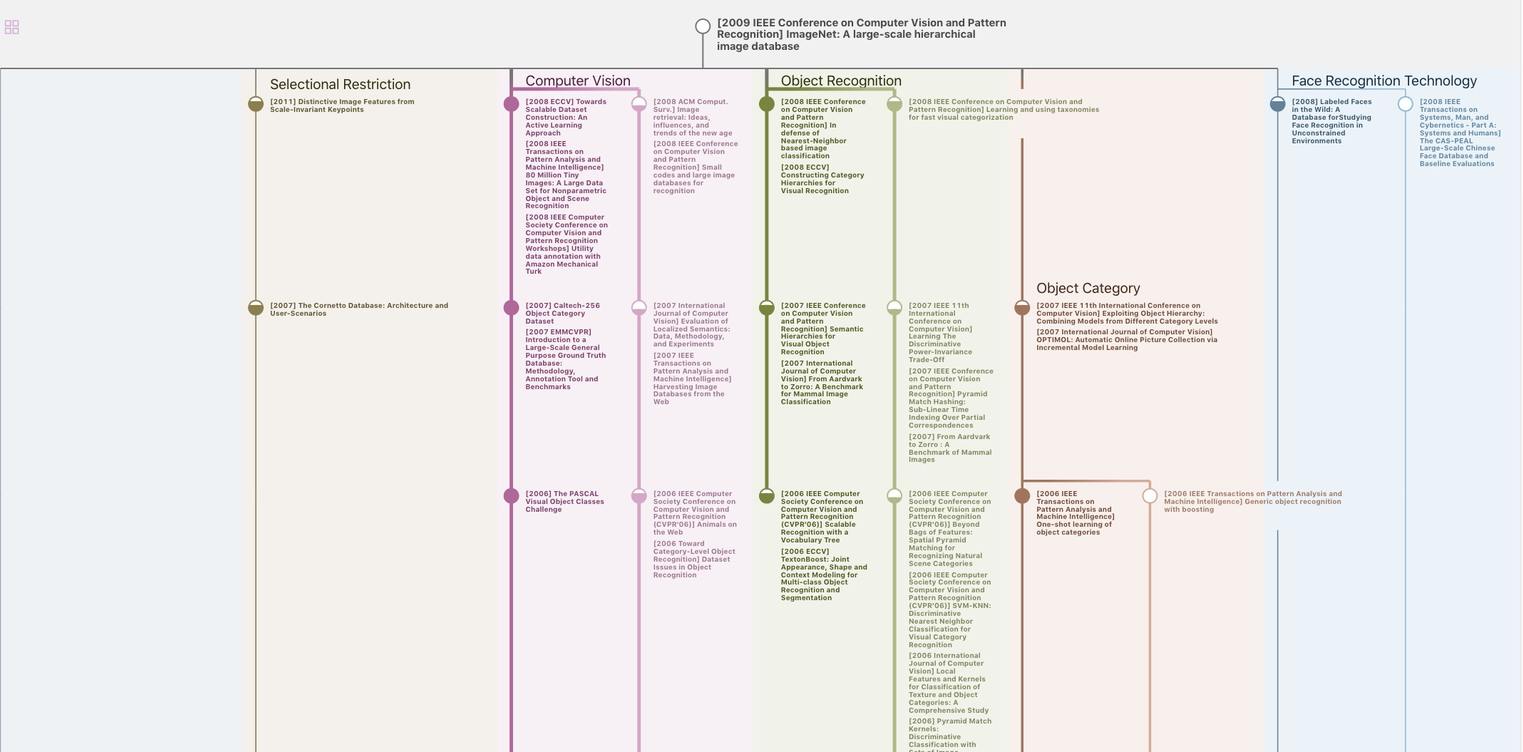
生成溯源树,研究论文发展脉络
Chat Paper
正在生成论文摘要