A Diffusion Model with State Estimation for Degradation-Blind Inverse Imaging
AAAI 2024(2024)
摘要
Solving the task of inverse imaging problems can restore unknown clean images from input measurements that have incomplete information. Utilizing powerful generative models, such as denoising diffusion models, could better tackle the ill-posed issues of inverse problems with the distribution prior of the unknown clean images. We propose a learnable state-estimator-based diffusion model to incorporate the measurements into the reconstruction process. Our method makes efficient use of the pre-trained diffusion models with computational feasibility compared to the conditional diffusion models, which need to be trained from scratch. In addition, our pipeline does not require explicit knowledge of the image degradation operator or make the assumption of its form, unlike many other works that use the pre-trained diffusion models at the test time. The experiments on three typical inverse imaging problems (both linear and non-linear), inpainting, deblurring, and JPEG compression restoration, have comparable results with the state-of-the-art methods.
更多查看译文
关键词
CV: Computational Photography, Image & Video Synthesis,CV: Large Vision Models,CV: Low Level & Physics-based Vision,ML: Deep Generative Models & Autoencoders
AI 理解论文
溯源树
样例
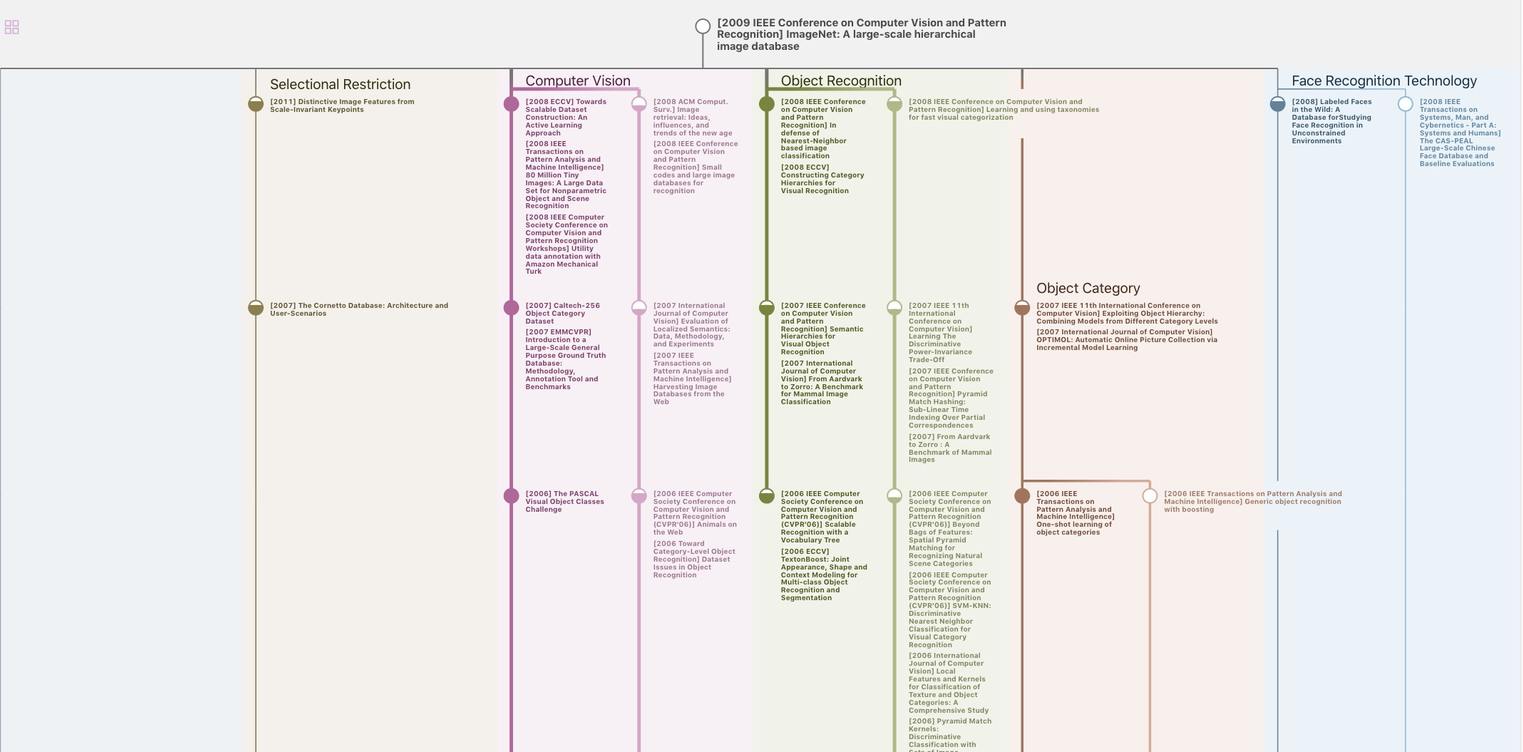
生成溯源树,研究论文发展脉络
Chat Paper
正在生成论文摘要