DeepIC3: Guiding IC3 Algorithms by Graph Neural Network Clause Prediction
2024 29th Asia and South Pacific Design Automation Conference (ASP-DAC)(2024)
摘要
In recent years, machine learning has demonstrated its potential in many challenging problems. In this paper, we extend its use to hardware formal property verification and propose DeepIC3, a method that takes advantage of graph learning in the classic IC3/PDR algorithm. In DeepIC3, graph neural networks are integrated to improve the result of local inductive generalization. This helps provide a global view of the state transition system and can potentially lead the algorithm out of local optima in the search of inductive invariants. Our experiments demonstrate that DeepIC3 accelerates the vanilla algorithm in nontrivial test cases of hardware model checking competition benchmarks (HWMCC2020) with up to 10. 8x speed-up. The proposed machine-learning integration preserves soundness and is universally applicable to various IC3/PDR implementations.
更多查看译文
关键词
model checking,property directed reachability,inductive generalization,graph learning
AI 理解论文
溯源树
样例
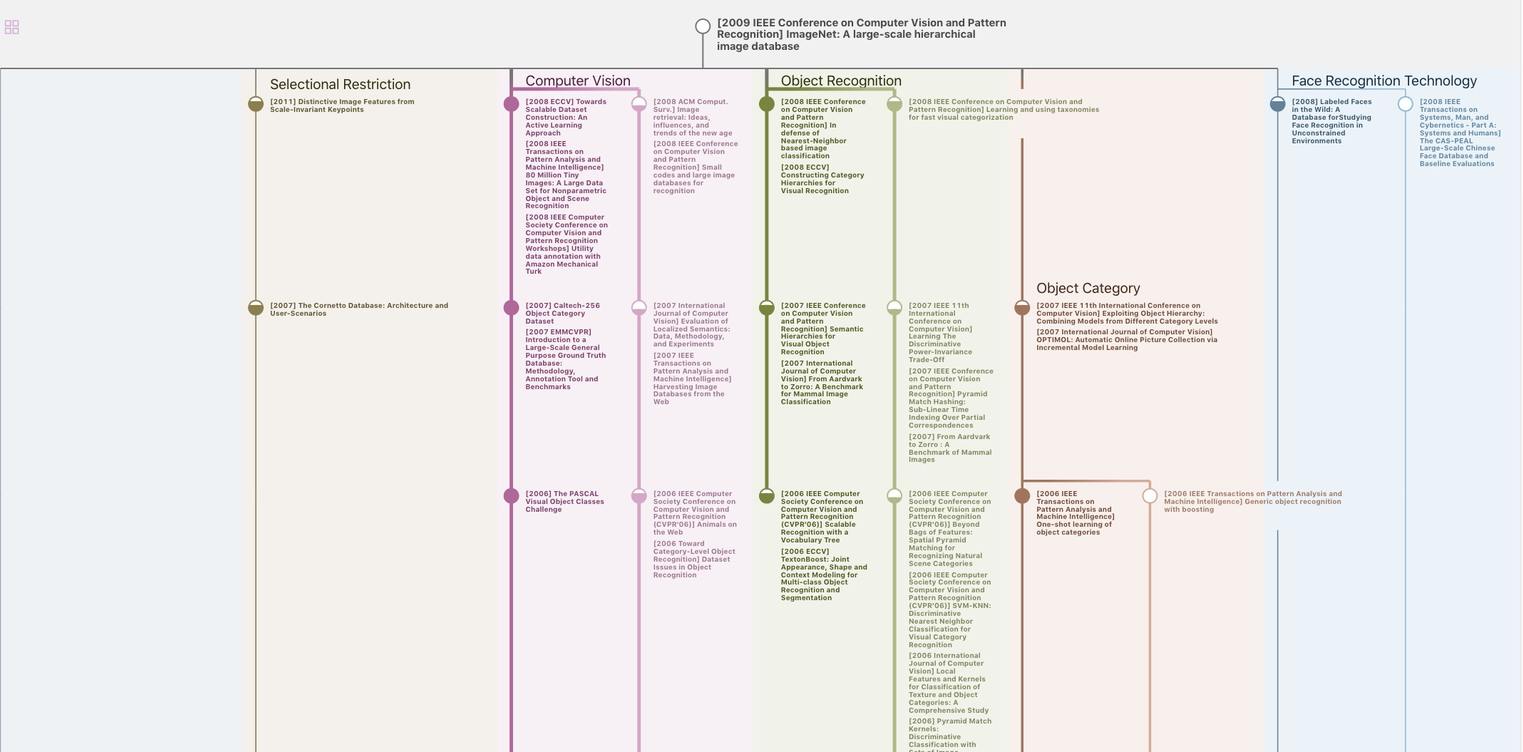
生成溯源树,研究论文发展脉络
Chat Paper
正在生成论文摘要