Evaluating the Portability of SepsisWatch: A Multi-Site External Validation of a Sepsis Machine Learning Model
crossref(2024)
摘要
Importance Sepsis accounts for a substantial portion of global deaths and healthcare costs. Early detection using machine learning (ML) models offers a critical opportunity to improve care and reduce the burden of sepsis.
Objective To externally validate the SepsisWatch ML model, initially developed at Duke University, in a community healthcare and assess its performance and clinical utility in early sepsis detection.
Design This retrospective external validation study evaluated the performance of the SepsisWatch model in a new environment. Data from patient encounters at Summa Health’s emergency departments between 2020 and 2021 were used. The study analyzed the model’s ability to predict sepsis using a combination of static and dynamic patient data.
Setting The study was conducted at Summa Health, a nonprofit healthcare system in Northeast Ohio, covering two emergency departments (EDs) associated with acute care hospitals, and two standalone EDs.
Participants Encounters associated with adult patients in any of Summa Health’s four EDs were included. Encounters lasting <1 hour were excluded. Only the first 36 hours of each encounter were used in model evaluation.
Intervention(s)/Exposure(s) The SepsisWatch model was used to predict sepsis based on patient data.
Main Outcome(s) and Measure(s) The primary outcomes measured were the model’s area under the precision-recall curve (AUPRC), and area under the receiver operator curve (AUROC).
Results The study included 205,005 encounters from 101,584 unique patients. 54.7% (n = 112,223) patients were female and the mean age was 50 (IQR, [38,71]). The model demonstrated strong performance across the Summa Health system, with little variation across different sites. The AUROC ranged from 0.906 to 0.960, and the AUPRC ranged from 0.177 to 0.252 across the four sites.
Conclusions and Relevance The external validation of the SepsisWatch model in a community health system setting confirmed its robust performance and portability across different geographical and demographic contexts. The study underscores the potential of advanced ML models in improving sepsis detection in both academic and community hospital settings, paving the way for prospective studies to measure the clinical and operational impact of such models in healthcare.
### Competing Interest Statement
Mr. Ratliff reported writing software licensed to Fullsteam Health. Mr. Gao reported writing software licensed to Clinetic, Cohere Med, and Fullsteam Health. Mr. Gao reported owning equity in Clinetic. Mr. Gardner reported writing software licensed to Fullsteam Health. Mr. Nichols reported writing software licensed to Clinetic, Cohere Med, and Fullsteam Health. Mr. Nichols reported owning equity in Clinetic. Mr. Revoir reported writing software licensed to Clinetic, Cohere Med, and Fullsteam Health. Mr. Revoir reported owning equity in Clinetic. Dr. Patel reported receiving grants from HeartFlow, Bayer, Janssen, and Novartis outside the submitted work. Mr. Balu reported writing software licensed to Clinetic, Cohere Med, and Fullsteam Health. Mr. Balu reported owning equity in Clinetic. Dr. Sendak reported writing software licensed to Clinetic, Cohere Med, KelaHealth, and Fullsteam Health. Dr. Sendak reported owning equity in Clinetic. Dr. Sendak and Mr. Balu reported receiving grants from the Gordon and Betty Moore Foundation and the Patrick J McGovern Foundation. No other disclosures were reported.
### Funding Statement
This study was funded by National Institute of General Medical Sciences (NIGMS) Valid : Bruno Valan, Anusha Prakash, William Ratliff, Michael Gao, Srikanth Muthya, Ajit Thomas, Jennifer L Eaton, Matt Gardner, Marshall Nichols, Mike Revoir, Dustin Tart, Cara O'Brien, Manesh Patel, Suresh Balu, Mark Sendak 5R42GM144999
### Author Declarations
I confirm all relevant ethical guidelines have been followed, and any necessary IRB and/or ethics committee approvals have been obtained.
Yes
The details of the IRB/oversight body that provided approval or exemption for the research described are given below:
The Summa Health Institutional Review Board granted IRB approval for this project.
I confirm that all necessary patient/participant consent has been obtained and the appropriate institutional forms have been archived, and that any patient/participant/sample identifiers included were not known to anyone (e.g., hospital staff, patients or participants themselves) outside the research group so cannot be used to identify individuals.
Yes
I understand that all clinical trials and any other prospective interventional studies must be registered with an ICMJE-approved registry, such as ClinicalTrials.gov. I confirm that any such study reported in the manuscript has been registered and the trial registration ID is provided (note: if posting a prospective study registered retrospectively, please provide a statement in the trial ID field explaining why the study was not registered in advance).
Yes
I have followed all appropriate research reporting guidelines, such as any relevant EQUATOR Network research reporting checklist(s) and other pertinent material, if applicable.
Yes
All data produced in the present study are available upon reasonable request to the authors
更多查看译文
AI 理解论文
溯源树
样例
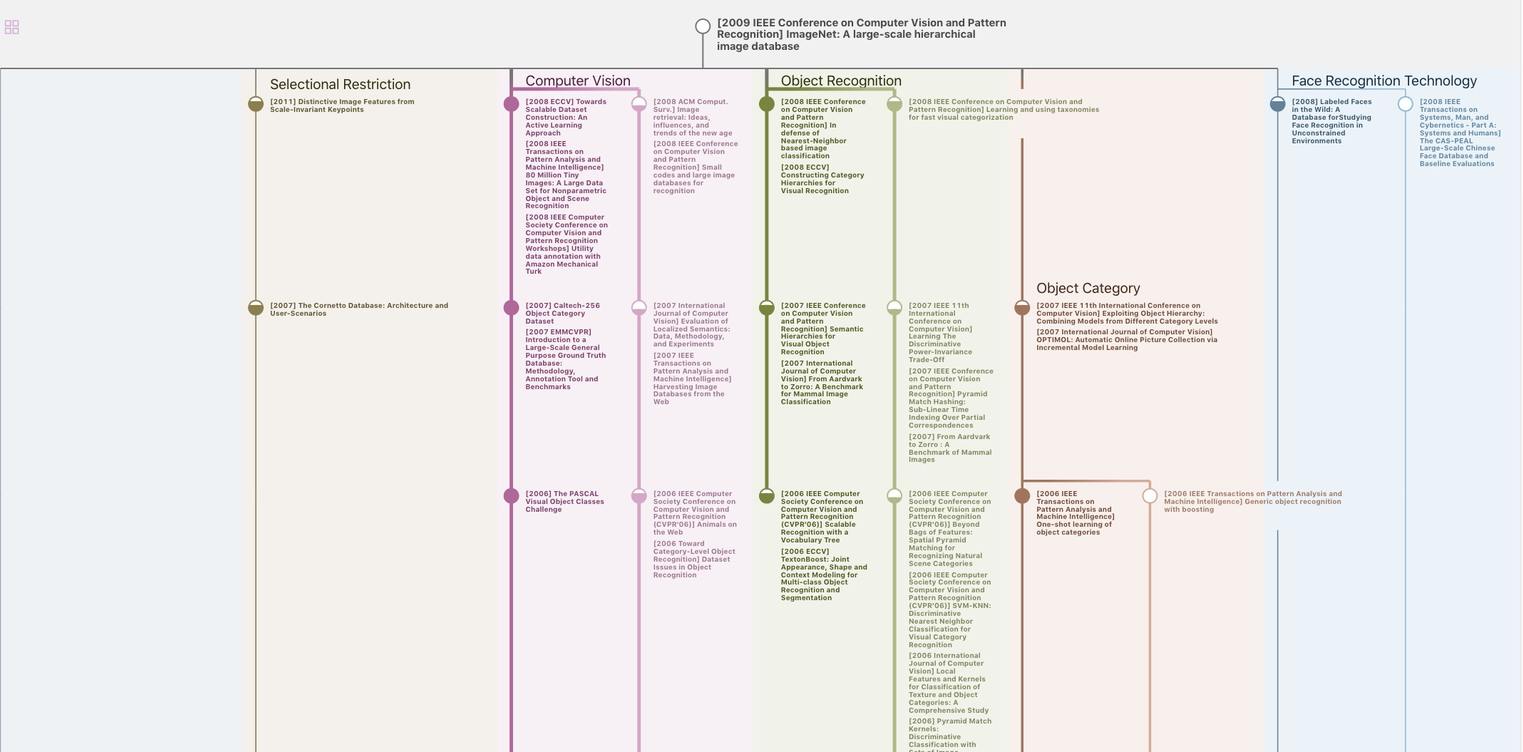
生成溯源树,研究论文发展脉络
Chat Paper
正在生成论文摘要