No Two Users Are Alike: Generating Audiences with Neural Clustering for Temporal Point Processes
Doklady Mathematics(2023)
摘要
Identifying the right user to target is a common problem for different Internet platforms. Although numerous systems address this task, they are heavily tailored for specific environments and settings. It is challenging for practitioners to apply these findings to their problems. The reason is that most systems are designed for settings with millions of highly active users and with personal information, as is the case in social networks or other services with high virality. There exists a gap in the literature for systems that are for medium-sized data and where the only data available are the event sequences of a user. It motivates us to present Look-A-Liker (LAL) as an unsupervised deep cluster system. It uses temporal point processes to identify similar users for targeting tasks. We use data from the leading Internet marketplace for the gastronomic sector for experiments. LAL generalizes beyond proprietary data. Using event sequences of users, it is possible to obtain state-of-the-art results compared to novel methods such as Transformer architectures and multimodal learning. Our approach produces the up to 20% ROC AUC score improvement on real-world datasets from 0.803 to 0.959. Although LAL focuses on hundreds of thousands of sequences, we show how it quickly expands to millions of user sequences. We provide a fully reproducible implementation with code and datasets in https://github.com/adasegroup/sequence_clusterers .
更多查看译文
关键词
applications,clustering,unsupervised,temporal point processes
AI 理解论文
溯源树
样例
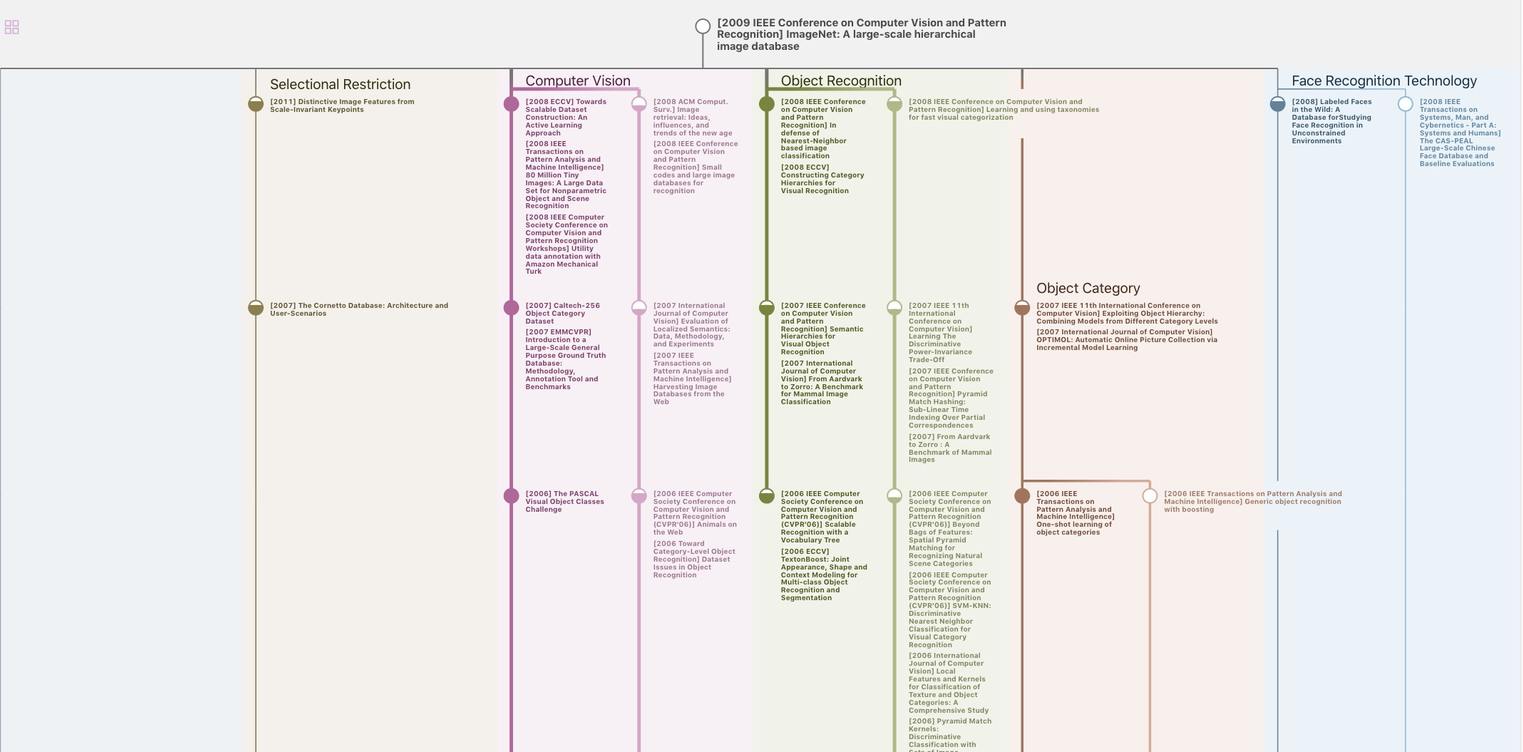
生成溯源树,研究论文发展脉络
Chat Paper
正在生成论文摘要