Resource- and Workload-aware Malware Detection through Distributed Computing in IoT Networks
2024 29th Asia and South Pacific Design Automation Conference (ASP-DAC)(2024)
摘要
Networked IoT systems have emerged in recent years to facilitate seamless connectivity, portability, and smarter functionality. Despite lending a plethora of benefits, IoT devices are exploited by adversaries for various illicit purposes. IoT systems are a popular target due to the lack of security traits in the design, and minimal available computational and storage resources on the devices. Among multiple threats, malicious applications a.k.a malware are seen as a pivotal security threat on IoT devices and networks. Many malware detection techniques have been proposed recently. However, the existing techniques focus either on general-purpose systems or assume the availability of abundant resources at their disposal for malware detection. However, for IoT devices, the ongoing workloads such as sensing, and on-device computations further minimize the available resources for malware detection. We propose a novel resource- and workload-aware malware detection integrated with distributed computing for IoT networked systems to address these challenges. The device analyzes the available resources for malware detection using a lightweight regression model. Depending on the available resources, ongoing workload executions, and communication cost the malware detection task is either performed on-device or offloaded to neighboring IoT nodes with sufficient resources. To ensure data integrity and user privacy, instead of offloading the whole malware detection, the classifier is partitioned and distributed over multiple nodes and further integrated at the parent node for malware detection. Experimental analysis shows that the proposed technique can achieve a speed-up of $9.8 \times$ compared to on-device inference while maintaining a malware detection accuracy of 96.7%.
更多查看译文
关键词
Distributed Computing,Internet Of Things Networks,Detection Techniques,Sufficient Resources,Root Node,Internet Of Things Devices,Internet Of Things Nodes,Learning Algorithms,Dynamic Analysis,Convolutional Neural Network,Artificial Neural Network,Convolutional Layers,Real-time Detection,Image Classification,Resource Consumption,Grayscale Images,Convolutional Neural Network Model,Machine Learning Classifiers,Single Node,Benign Samples,Inference Task,Binary Files,Static Analysis,Context Switching,Birth Order,Executable File,First Child,Stochastic Gradient Descent,Language Model
AI 理解论文
溯源树
样例
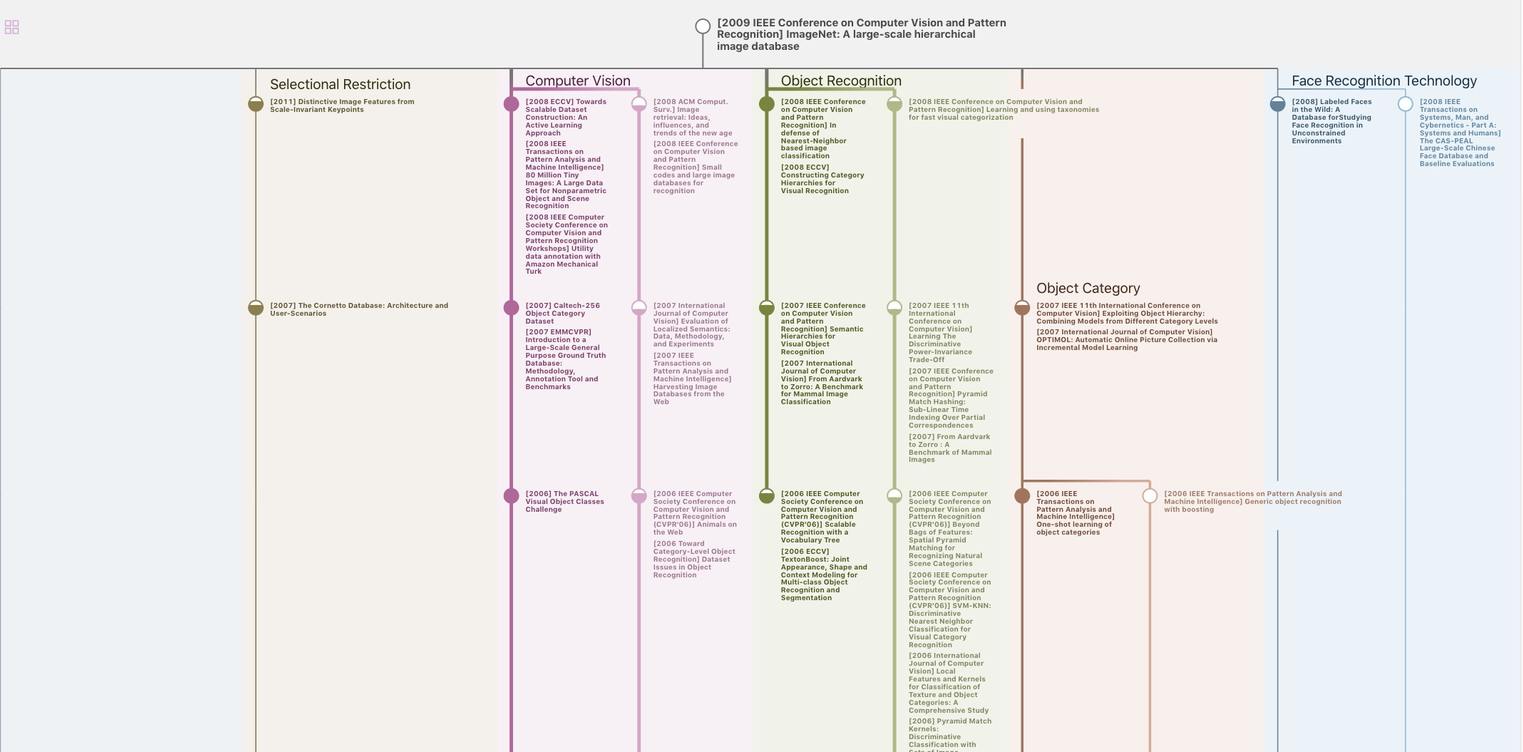
生成溯源树,研究论文发展脉络
Chat Paper
正在生成论文摘要