Unveiling the Future of Human and Machine Coding: A Survey of End-to-End Learned Image Compression
ENTROPY(2024)
Abstract
End-to-end learned image compression codecs have notably emerged in recent years. These codecs have demonstrated superiority over conventional methods, showcasing remarkable flexibility and adaptability across diverse data domains while supporting new distortion losses. Despite challenges such as computational complexity, learned image compression methods inherently align with learning-based data processing and analytic pipelines due to their well-suited internal representations. The concept of Video Coding for Machines has garnered significant attention from both academic researchers and industry practitioners. This concept reflects the growing need to integrate data compression with computer vision applications. In light of these developments, we present a comprehensive survey and review of lossy image compression methods. Additionally, we provide a concise overview of two prominent international standards, MPEG Video Coding for Machines and JPEG AI. These standards are designed to bridge the gap between data compression and computer vision, catering to practical industry use cases.
MoreTranslated text
Key words
deep learning,image compression,video coding,neural compression,coding for machines
AI Read Science
Must-Reading Tree
Example
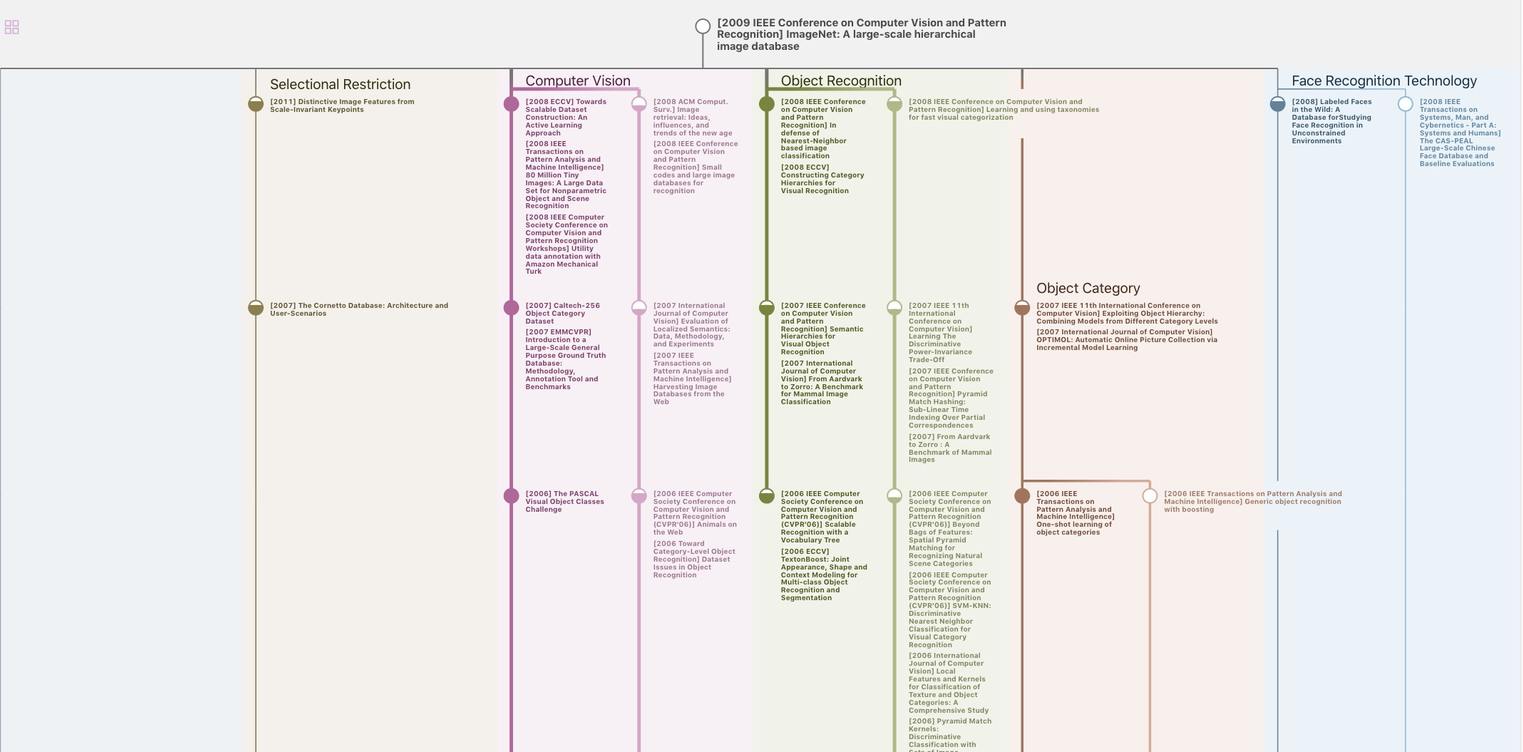
Generate MRT to find the research sequence of this paper
Chat Paper
Summary is being generated by the instructions you defined