Improving Panoptic Narrative Grounding by Harnessing Semantic Relationships and Visual Confirmation
AAAI 2024(2024)
摘要
Recent advancements in single-stage Panoptic Narrative Grounding (PNG) have demonstrated significant potential. These methods predict pixel-level masks by directly matching pixels and phrases. However, they often neglect the modeling of semantic and visual relationships between phrase-level instances, limiting their ability for complex multi-modal reasoning in PNG. To tackle this issue, we propose XPNG, a “differentiation-refinement-localization” reasoning paradigm for accurately locating instances or regions. In XPNG, we introduce a Semantic Context Convolution (SCC) module to leverage semantic priors for generating distinctive features. This well-crafted module employs a combination of dynamic channel-wise convolution and pixel-wise convolution to embed semantic information and establish inter-object relationships guided by semantics. Subsequently, we propose a Visual Context Verification (VCV) module to provide visual cues, eliminating potential space biases introduced by semantics and further refining the visual features generated by the previous module. Extensive experiments on PNG benchmark datasets reveal that our approach achieves state-of-the-art performance, significantly outperforming existing methods by a considerable margin and yielding a 3.9-point improvement in overall metrics. Our codes and results are available at our project webpage: https://github.com/TianyuGoGO/XPNG.
更多查看译文
关键词
CV: Multi-modal Vision,CV: Language and Vision
AI 理解论文
溯源树
样例
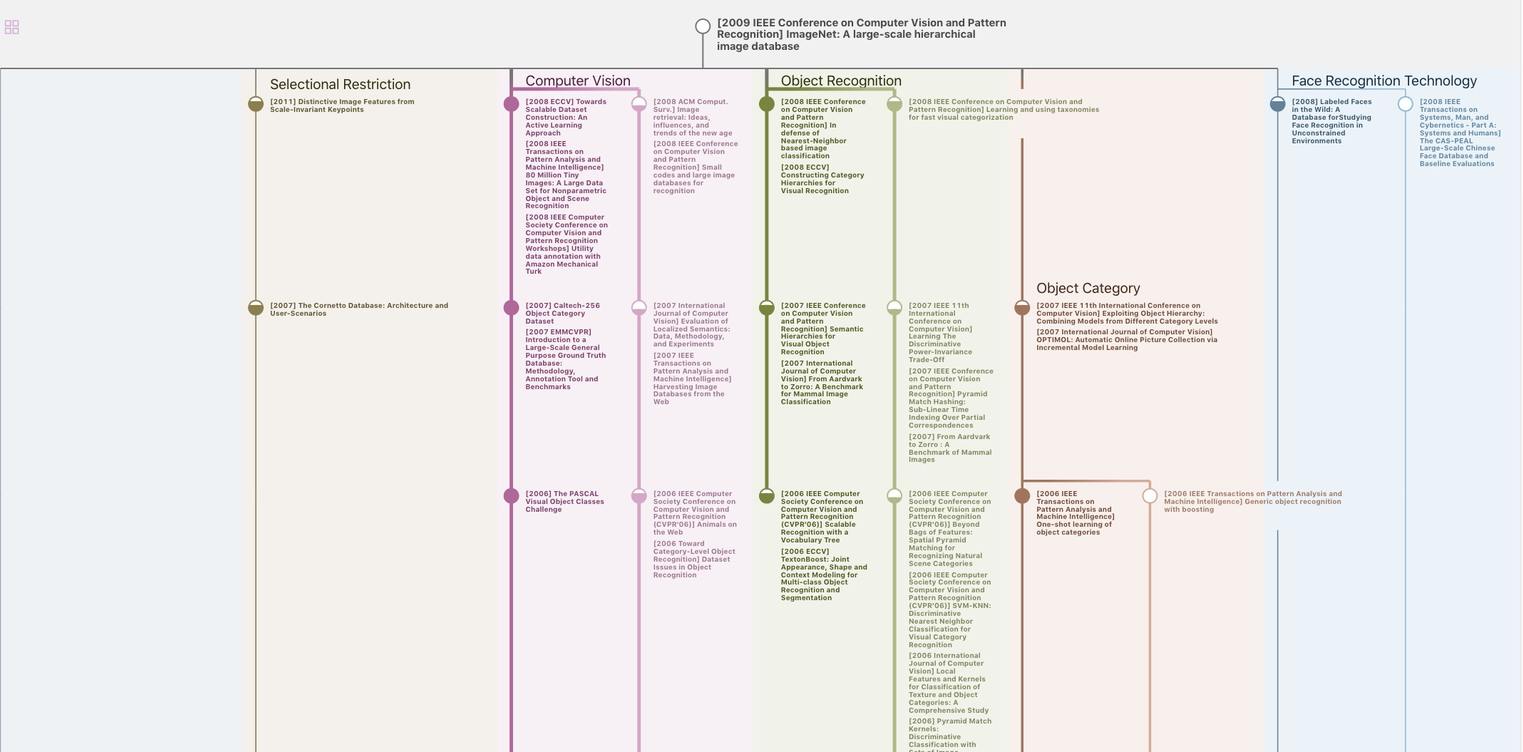
生成溯源树,研究论文发展脉络
Chat Paper
正在生成论文摘要