Layer Compression of Deep Networks with Straight Flows
AAAI 2024(2024)
摘要
Very deep neural networks lead to significantly better performance on various real tasks. However, it usually causes slow inference and is hard to be deployed on real-world devices. How to reduce the number of layers to save memory and to accelerate the inference is an eye-catching topic.
In this work, we introduce an intermediate objective, a continuous-time network, before distilling deep networks into shallow networks.
First, we distill a given deep network into a continuous-time neural flow model, which can be discretized with an ODE solver and the inference requires passing through the network multiple times.
By forcing the flow transport trajectory to be straight lines, we find that it is easier to compress the infinite step model into a one-step neural flow model, which only requires passing through the flow model once.
Secondly, we refine the one-step flow model together with the final head layer with knowledge distillation and finally, we can replace the given deep network with this one-step flow network.
Empirically, we demonstrate that our method outperforms direct distillation and other baselines on different model architectures (e.g. ResNet, ViT) on image classification and semantic segmentation tasks.
We also manifest that our distilled model naturally serves as an early-exit dynamic inference model.
更多查看译文
关键词
ML: Applications,ML: Deep Learning Algorithms,CV: Applications
AI 理解论文
溯源树
样例
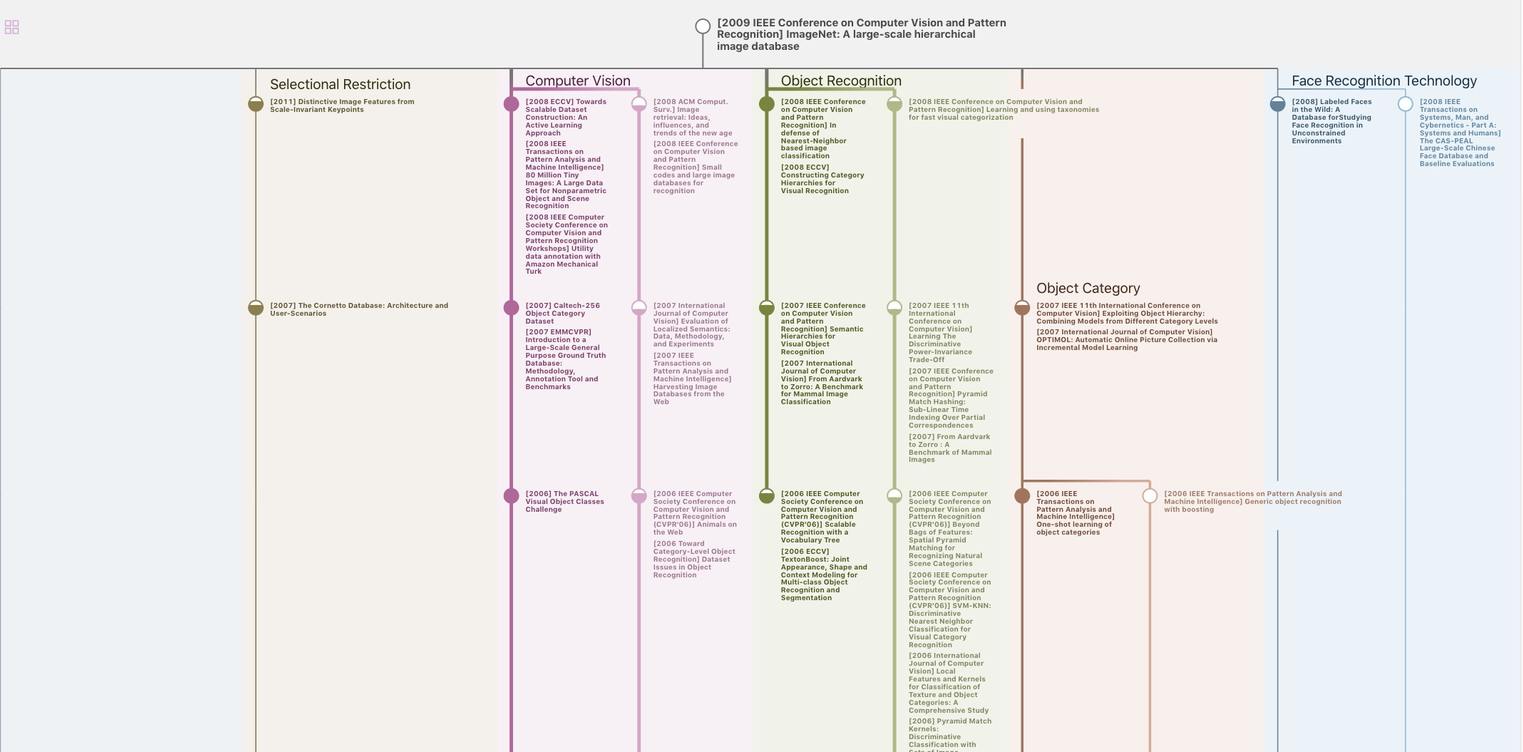
生成溯源树,研究论文发展脉络
Chat Paper
正在生成论文摘要