Optimizing Steering Feedback Torque with Reinforcement Learning guided by Human Reward Model
IEEE Transactions on Transportation Electrification(2024)
Abstract
Steering feel holds a main role in driving, making steering feedback torque (SFT) generation an important research of steer-by-wire (SBW) system. Authentic steering feel provides sensations comparable to conventional steering systems, while the SFT model aligns with driver preferences, reducing cognitive load and fatigue, thereby improving vehicle safety. Current research on driver-preferred SFT is limited to real-world trials, a method often time-intensive and suboptimal. This paper presents an innovative approach for obtaining optimal SFT with reinforcement learning (RL) guided by human reward model. The human reward model, trained on human feedback data, is introduced to guide SFT model’s optimization. Given complexity of assessing human evaluations, the reward model is validated with virtual data. Building upon previous data-driven SFT modeling, a fine-tuning method leveraging the RL method is presented, using human reward model’s outputs. This approach retains the nonlinear predictive capabilities of the pre-trained model while aligning with human evaluations. Results confirm the human reward model’s ability to replicate human SFT standards. Models adapted using this network retain the nonlinear predictive capabilities of the initial network, even under high-frequency inputs. Overall, this work pioneers the integration of human feedback into SFT optimization, providing novel insights for the advancement of SBW systems.
MoreTranslated text
Key words
Steering Feedback Torque,Human Feedback,Model Fine-tuning,Reward Model Generalization,Reinforcement Learning
AI Read Science
Must-Reading Tree
Example
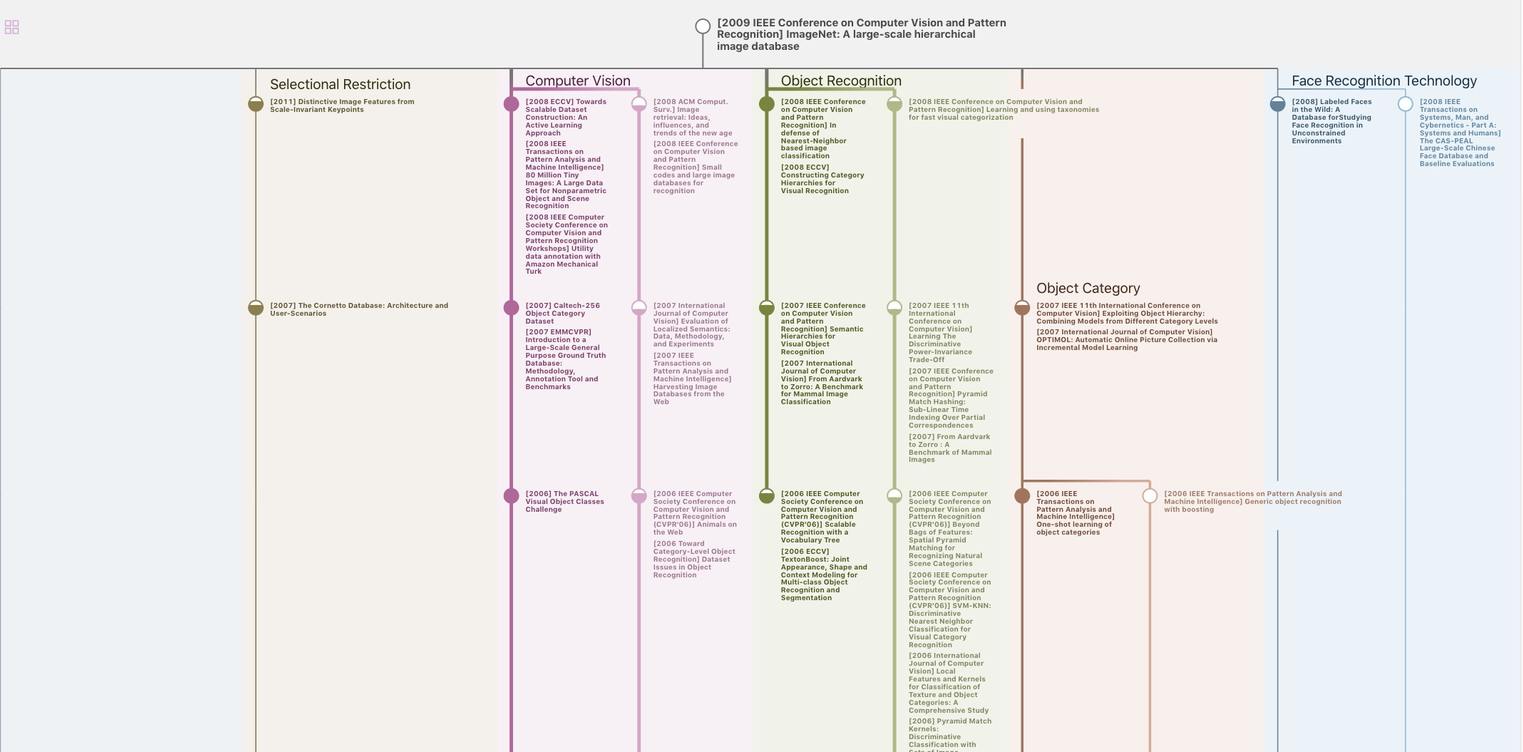
Generate MRT to find the research sequence of this paper
Chat Paper
Summary is being generated by the instructions you defined