Urban Damage-Level Estimation With Reconstructed Quad-Pol SAR Data From Dual-Pol SAR Mode
IEEE GEOSCIENCE AND REMOTE SENSING LETTERS(2024)
摘要
Urban damage investigation is an important application for a polarimetric synthetic aperture radar (SAR), which is capable of sensing the target scattering mechanism changes before and after a natural disaster. A quad-pol SAR with fully polarimetric acquisition capability can better sense the scattering mechanism changes. Meanwhile, a dual-pol SAR with wider swath is suitable for large area monitoring. In this vein, this work dedicates to generating pseudo-quad-pol SAR data from dual-pol SAR mode to partially reconstruct fully polarimetric information. The main contributions contain two aspects. First, a multiscale feature aggregation convolutional neural network (CNN) has been proposed to reconstruct quad-pol SAR data, which includes a feature extraction (FE) module to collect multiscale features from dual-pol SAR data in spatial and polarimetric domain and a feature translation (FT) network aggregated with attention modules to deeply fuse the stacked multiscale features and map them to quad-pol SAR covariance matrices. Then, an urban damage-level estimation approach has been established with reconstructed quad-pol SAR data based on polarimetric coherence pattern interpretation tool. Experimental studies have been carried out in terms of both pseudo-quad-pol SAR data reconstruction and urban damage-level estimation. Comparison results demonstrate that the proposed method achieves better quad-pol SAR data reconstruction accuracy and urban damage-level estimation accuracy. Moreover, compared with the urban damage-level estimated by real quad-pol SAR data, the proposed method can achieve 99.83% estimation consistency (EC) within 5% error tolerance (ET).
更多查看译文
关键词
Convolutional neural network (CNN),dual polarization,polarimetric coherence pattern,synthetic aperture radar (SAR),urban damage-level estimation
AI 理解论文
溯源树
样例
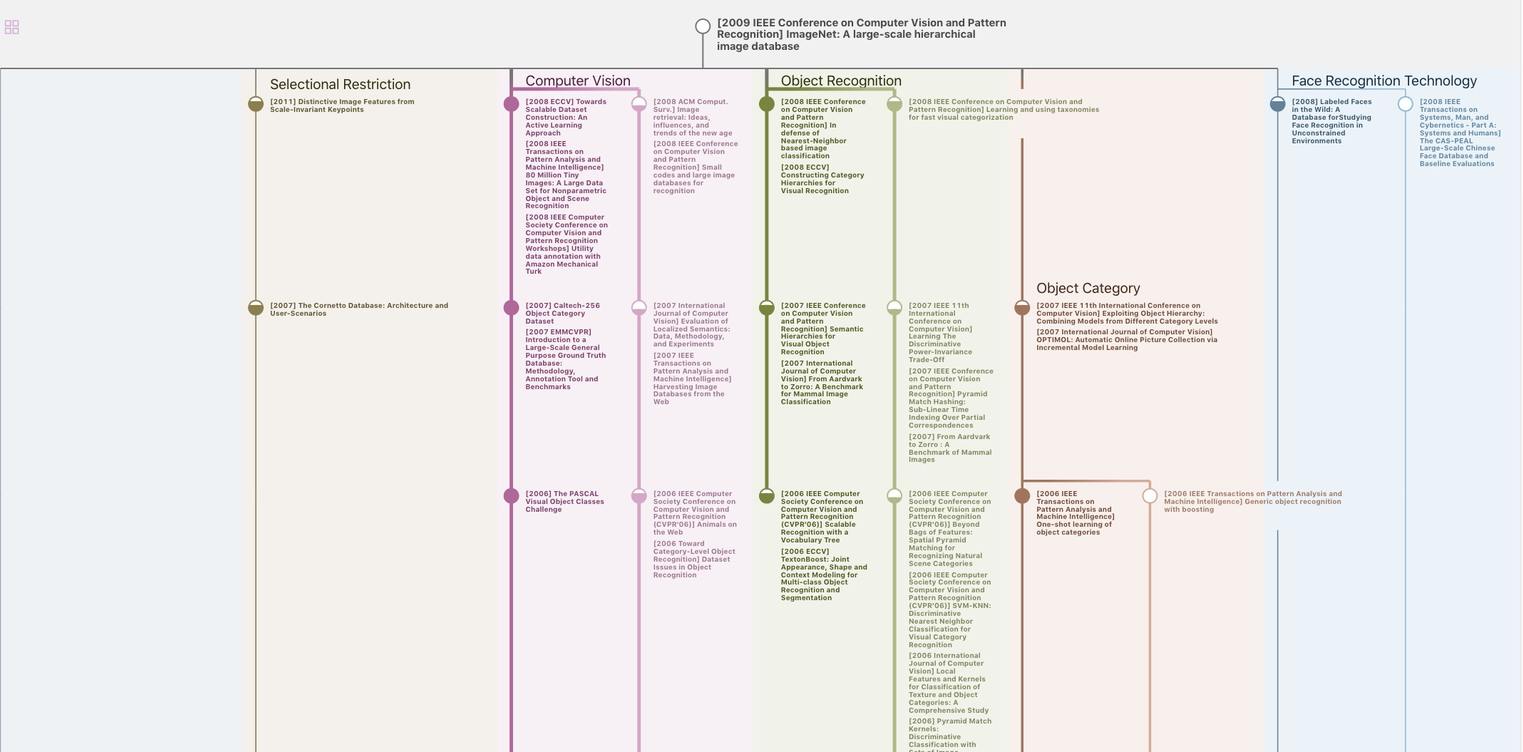
生成溯源树,研究论文发展脉络
Chat Paper
正在生成论文摘要