Employing Reinforcement Learning to Construct a Decision-making Environment for Image Forgery Localization
IEEE Transactions on Information Forensics and Security(2024)
摘要
The widespread misuse of advanced image editing tools and deep generative techniques has led to a proliferation of images with altered content in real-life scenarios, often without any discernible traces of tampering. This has created a potential threat to security and credibility of images. Image forgery localization is an urgent technique. In this paper, we propose a novel reinforcement learning-based framework CoDE (Construct Decision-making Environment) that can provide reliable localization result of tampered area in forged images. We model the forgery localization task as a Markov Decision Process (MDP), where each pixel is equipped with an agent that performs Gaussian distribution-based continuous action to iteratively update the respective forgery probability, so as to achieve pixel-level image forgery localization. In order to construct the state transitions within MDP, we propose a twin-flow state encoder to handle the updated state, which consists of the forged image and its corresponding forgery probability map. What’s more, considering that the tampered area is often sparse in practical image tampering scenarios, we design a reward function specifically for these sparse tampered area. This reward function can guide the agent to more effectively learn the optimal strategy for maximizing the cumulative reward. Extensive experiments conducted on a variety of benchmark datasets demonstrate CoDE’s superior localization accuracy and robustness against image degradation caused by transmission through Online Social Networks (OSNs) and various post-processing attacks.
更多查看译文
关键词
Information security,pixel-level image forgery localization,reinforcement learning,twin-flow state encoder,robustness
AI 理解论文
溯源树
样例
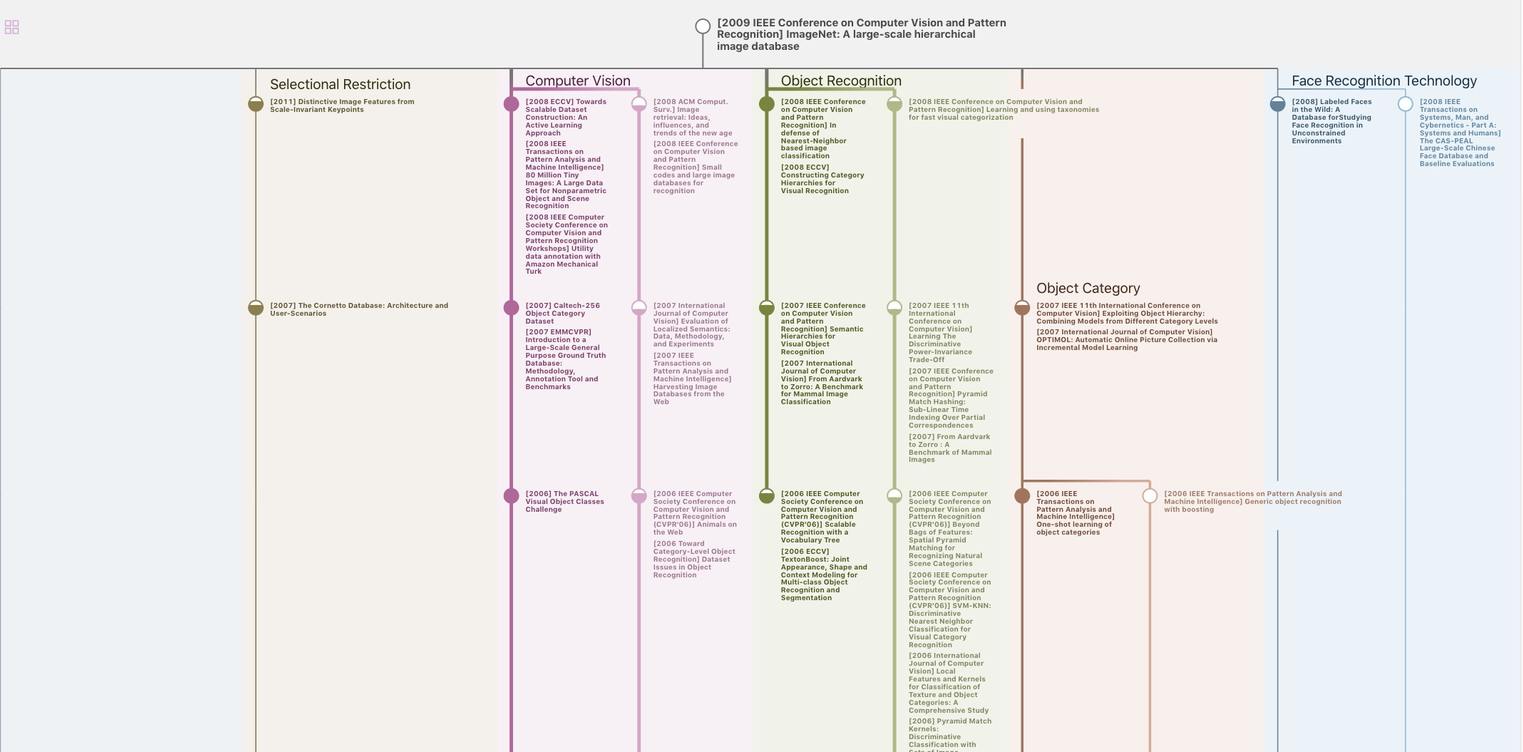
生成溯源树,研究论文发展脉络
Chat Paper
正在生成论文摘要