Moderately Dense Adaptive Feature Fusion Network for Infrared Small Target Detection
IEEE TRANSACTIONS ON GEOSCIENCE AND REMOTE SENSING(2024)
Key words
Coarse-to-fine detection head (CFHead),data augmentation,infrared small target detection,moderately dense adaptive feature fusion (MDAF) module,real-time detection
AI Read Science
Must-Reading Tree
Example
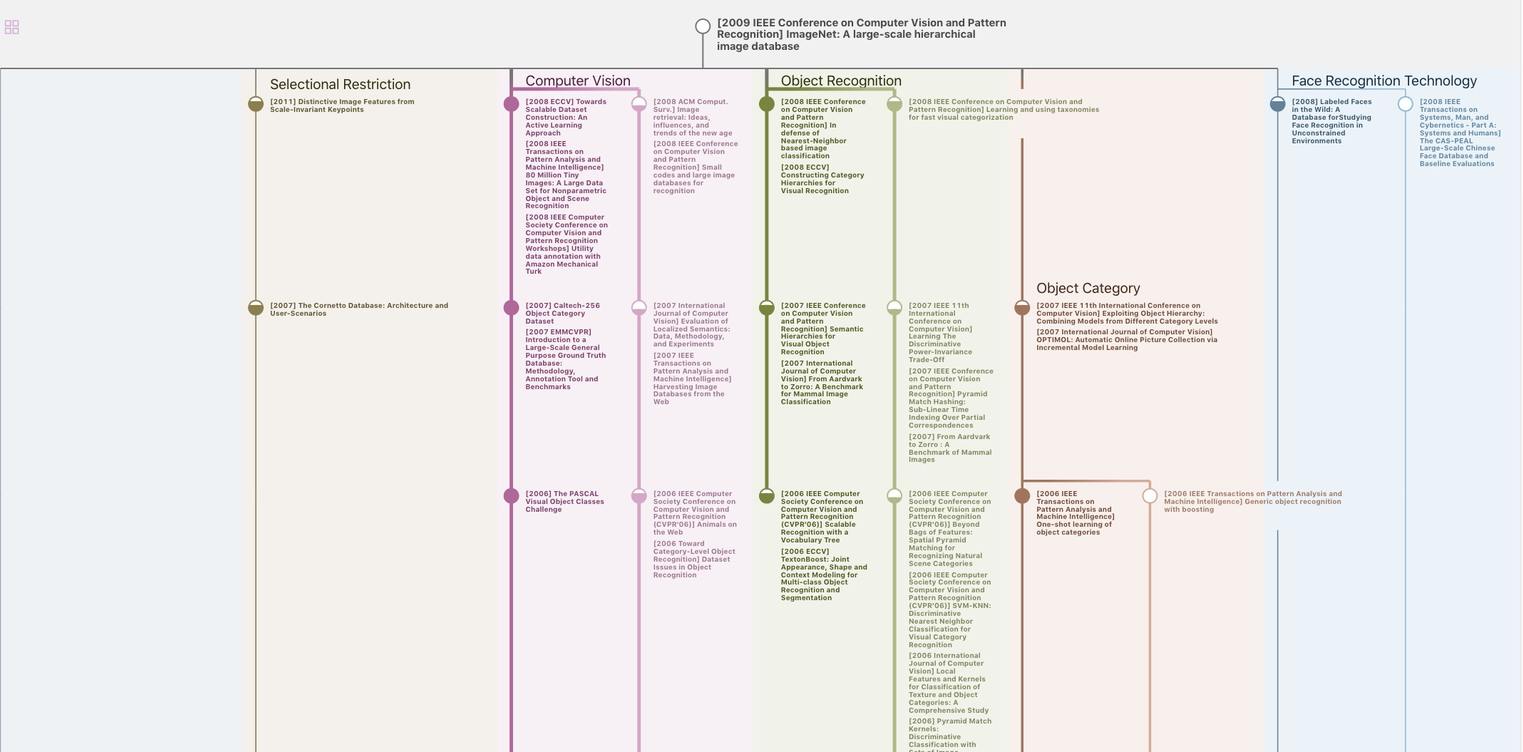
Generate MRT to find the research sequence of this paper
Chat Paper
Summary is being generated by the instructions you defined