Safe Learning by Constraint-Aware Policy Optimization for Robotic Ultrasound Imaging
IEEE Transactions on Automation Science and Engineering(2024)
摘要
Ultrasound-based medical examination usually requires establishing proper contact between an ultrasound probe and a human body that ensures the quality of ultrasound images. The scanning skills are quite challenging for a robot to learn primarily due to the complex coupling between the applied force profile and the resulting ultrasound image quality. While reinforcement learning appears as a powerful tool for learning complex robot skills, the deployment of these algorithms in medical robots demands special attention due to the evident safety concerns that arise from physical probe-tissue interactions. In this paper, we explicitly consider external constraints on the force magnitude when searching for the optimal policy parameters to enhance safety during ultrasound-guided robotic interventions. In particular, we study policy optimization under the framework of a constrained Markov decision process. The resulting gradient-based policy update is then subject to the involved constraints, which can be readily addressed by the primal-dual interior-point technique. In addition, upon the observation that policy update requires consecutive policies to be close to each other to have stable and robust performance with reinforcement learning algorithms, we design the learning rate of policy gradient from an imitation perspective. The performance of the proposed constraint-aware policy optimization method is validated with experiments of robotic ultrasound imaging for spinal diagnosis
Note to Practitioners
—This paper was motivated by the problem of safely learning the optimal interaction force strategy to facilitate robotic ultrasound imaging. Existing approaches to robotic ultrasound imaging usually empirically set a constant value for the scanning force, despite the fact the force strategy plays an important role in the quality of the ultrasound images. This paper suggests the usage of reinforcement learning to identify the optimal interaction force due to the complex acoustic coupling between the force and the ultrasound image quality. Specifically, we propose constraint-aware reinforcement learning in view of the safety-critical issues as a result of physical human-probe interaction. We then conduct a theoretical analysis of the proposed safe reinforcement learning, including monotonic improvement and policy value bound under mild assumptions. Preliminary real experiments on ultrasound imaging of the spine of a phantom for scoliosis assessment suggest that the proposed approach can safely learn the optimal scanning force without violating the prescribed force threshold. In the future, we would like to apply our approach to learning the optimal scanning force on different organs of interest of human subjects.
更多查看译文
关键词
Medical robotics,reinforcement learning,imitation learning,optimization,sequential decision making
AI 理解论文
溯源树
样例
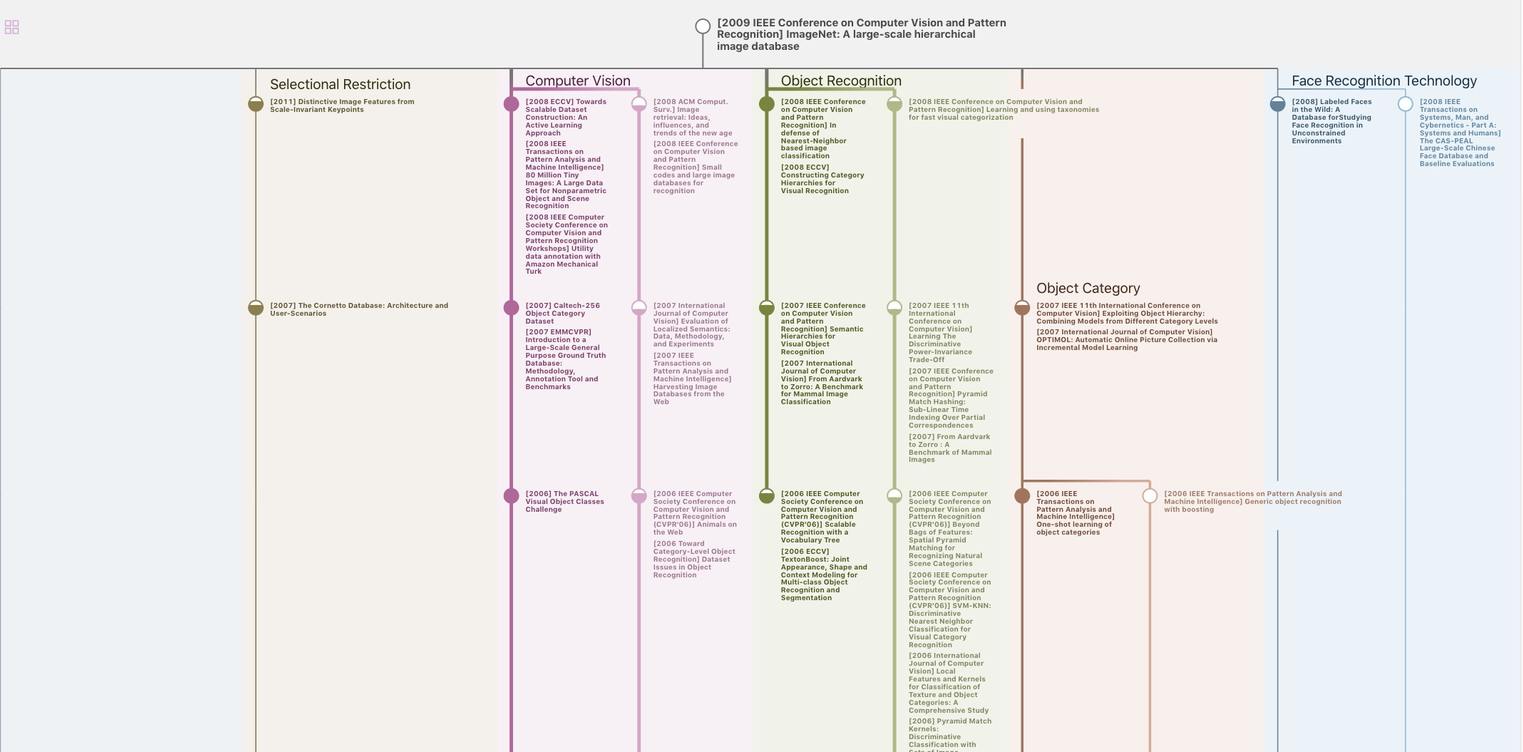
生成溯源树,研究论文发展脉络
Chat Paper
正在生成论文摘要