Autonomous Landing of the Quadrotor on the Mobile Platform via Meta Reinforcement Learning
IEEE TRANSACTIONS ON AUTOMATION SCIENCE AND ENGINEERING(2024)
摘要
Landing a quadrotor on a mobile platform moving with various unknown trajectories presents special challenges, including the requirements of fast trajectory planning/replanning, accurate control, and the adaptability for different target trajectories, especially when the platform is non-cooperative. However, previous works either assume the platform moves along a predefined trajectory or decouple planning from control which may cause a delay in tracking. In this work, we integrate planning and control into a unified framework and present an efficient off-policy Meta-Reinforcement Learning (Meta-RL) algorithm that enables a quadrotor (agent) to land on a mobile platform with various unknown trajectories autonomously. In our approach, we disentangle task-specific policy parameters by a separate adapter network to shared low-level parameters and learn a probabilistic encoder to extract common structures across different tasks. Specifically, during meta-training, we sample different trajectories from the task distribution, and then the probabilistic encoder accumulates the necessary statistics from past experience into the latent variables that enable the policy to perform the task. At meta-testing time, when the quadrotor is faced with an unseen trajectory, the latent variables can be sampled according to past interactions between the quadrotor and the mobile platform and held constant during an episode, enabling rapid trajectory-level adaptation. We assume similar tasks share a common low-dimensional structure in the representation of the policy network and the task-specific information is learned in the head of the policy. Accordingly, we further propose a separate adapter net as a supervised learning problem. The adapter net learns the weights of the policy's output layer for each meta-training task given by the environment interactions from the agent. When adapting to a new task during meta-testing, we fix the shared model layers and predict the head weights for the new task using the trained adapter network. This ensures that the pretrained policy can efficiently adapt to different tasks, which boosts the out-of-distribution performance. Our method can directly control the pitch, roll, yaw angle, and thrust of the quadrotor, yielding a fast response to the trajectory change. Simulation results show the superiority of our method both in success rate and adaptation efficiency over other RL algorithms on meta-testing tasks. The real-world experimental results compared with traditional planning and control algorithms demonstrate the satisfactory performance of our autonomous landing method, especially its robustness in adapting to unknown dynamics.
更多查看译文
关键词
Task analysis,Quadrotors,Trajectory,Reinforcement learning,Robots,Heuristic algorithms,Adaptation models,Quadrotor,meta reinforcement learning,autonomous landing,trajectory planning and control
AI 理解论文
溯源树
样例
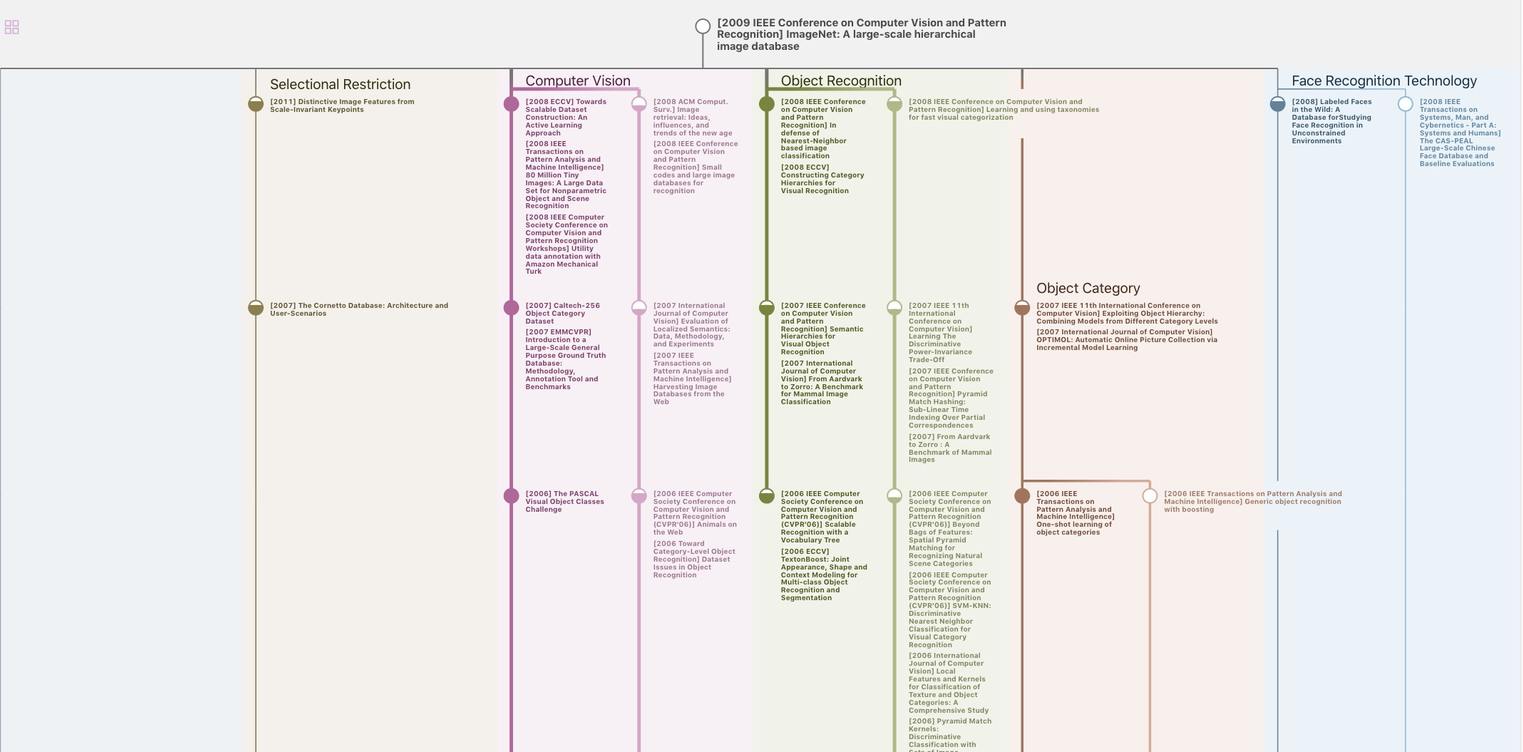
生成溯源树,研究论文发展脉络
Chat Paper
正在生成论文摘要