Convolutional Forecasting of Particulate Matter: Toward a Data-Driven Generalized Model
ATMOSPHERE(2024)
摘要
Air pollution poses a significant threat to human health and ecosystems. Forecasting the concentration of key pollutants like particulate matter can help support air quality planning and prevention measures. Deep learning methods are becoming increasingly popular for predicting air pollution and particulate matter concentration. Architectures like Convolutional Neural Networks can effectively account for the geographical features of the study domain. This work tests a Feed-Forward, a Long Short-Term Memory (LSTM), and a Convolutional Neural Network (CNN) on a polluted geographical domain in northern Italy. The best convolutional architecture was then implemented in two other quite different regions. The results show that the same CNN architecture provides remarkably accurate forecasts in all applications and that a network trained on PM10 data can accurately forecast PM2.5 concentrations up to 10 days ahead. These results suggest that the proposed CNN has high generalization capabilities and can thus be reliably used as a forecasting model for different areas.
更多查看译文
关键词
air quality,particulate matter,deep learning,multi-step forecasting,generalization
AI 理解论文
溯源树
样例
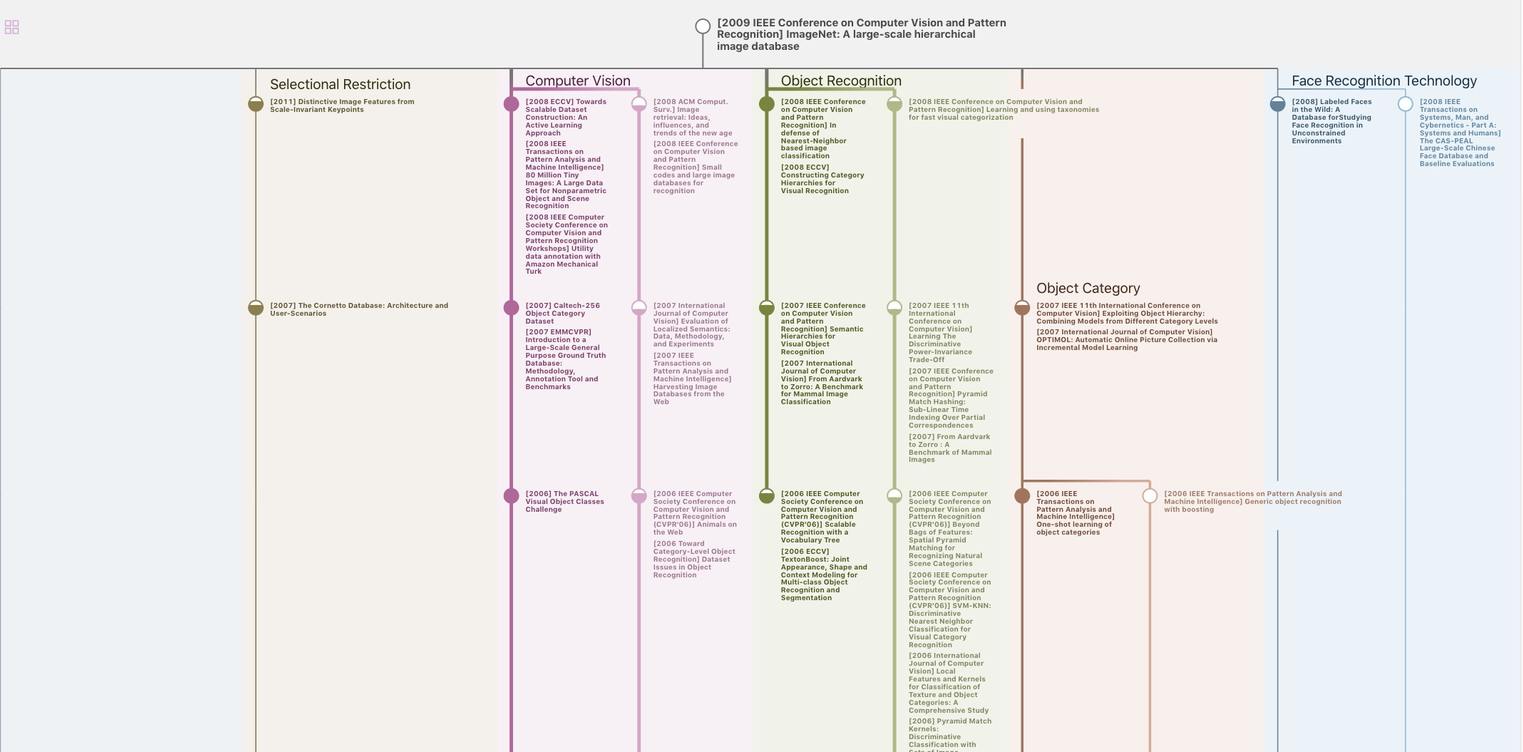
生成溯源树,研究论文发展脉络
Chat Paper
正在生成论文摘要