Learning to Learn Better Visual Prompts
AAAI 2024(2024)
摘要
Prompt tuning provides a low-cost way of adapting vision-language models (VLMs) for various downstream vision tasks without requiring updating the huge pre-trained parameters. Dispensing with the conventional manual crafting of prompts, the recent prompt tuning method of Context Optimization (CoOp) introduces adaptable vectors as text prompts. Nevertheless, several previous works point out that the CoOp-based approaches are easy to overfit to the base classes and hard to generalize to novel classes. In this paper, we reckon that the prompt tuning works well only in the base classes because of the limited capacity of the adaptable vectors. The scale of the pre-trained model is hundreds times the scale of the adaptable vector, thus the learned vector has a very limited ability to absorb the knowledge of novel classes. To minimize this excessive overfitting of textual knowledge on the base class, we view prompt tuning as learning to learn (LoL) and learn the prompt in the way of meta-learning, the training manner of dividing the base classes into many different subclasses could fully exert the limited capacity of prompt tuning and thus transfer it power to recognize the novel classes. To be specific, we initially perform fine-tuning on the base class based on the CoOp method for pre-trained CLIP. Subsequently, predicated on the fine-tuned CLIP model, we carry out further fine-tuning in an N-way K-shot manner from the perspective of meta-learning on the base classes. We finally apply the learned textual vector and VLM for unseen classes.Extensive experiments on benchmark datasets validate the efficacy of our meta-learning-informed prompt tuning, affirming its role as a robust optimization strategy for VLMs.
更多查看译文
关键词
CV: Language and Vision,CV: Large Vision Models,CV: Multi-modal Vision
AI 理解论文
溯源树
样例
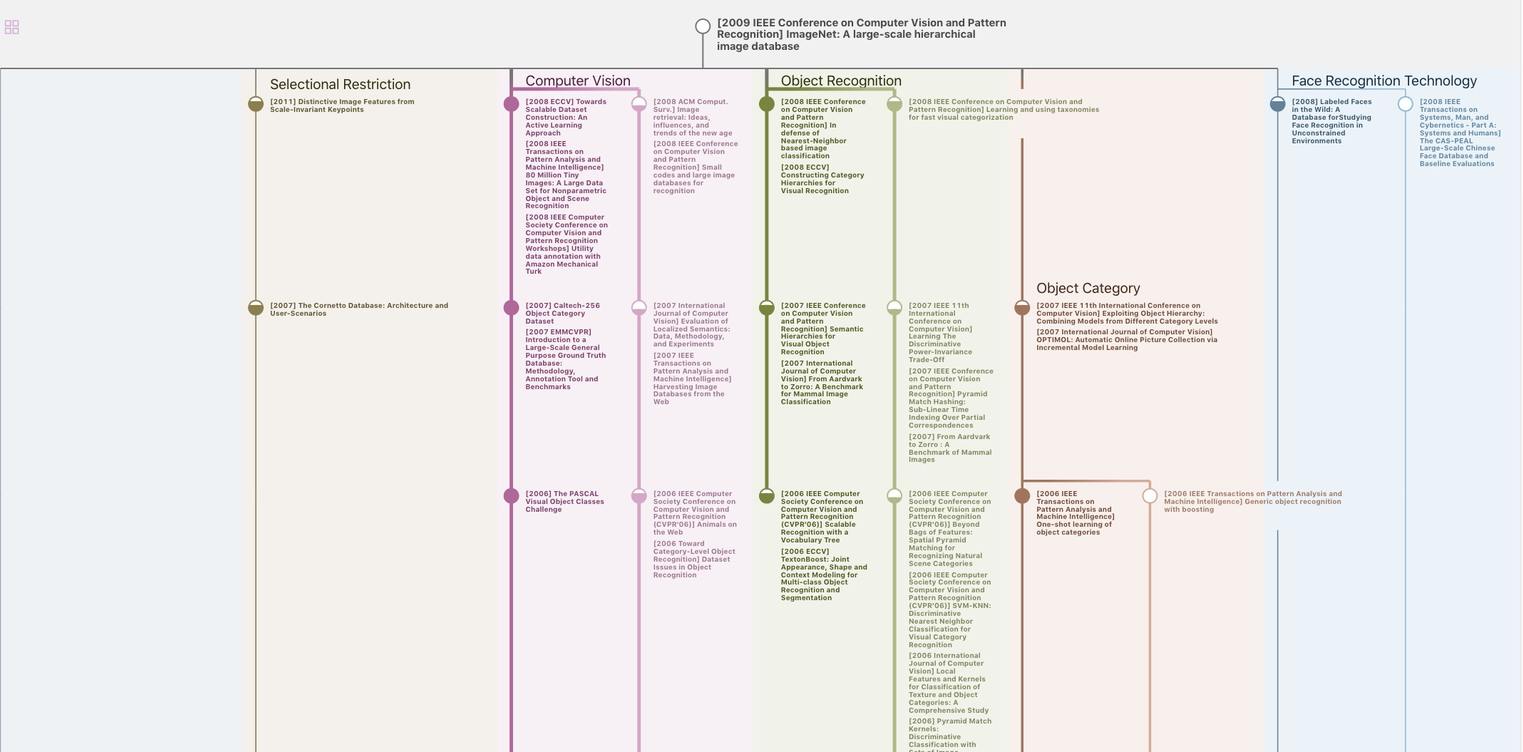
生成溯源树,研究论文发展脉络
Chat Paper
正在生成论文摘要