Generative Model Perception Rectification Algorithm for Trade-Off between Diversity and Quality
Proceedings of the AAAI Conference on Artificial Intelligence(2024)
摘要
How to balance the diversity and quality of results from generative models through perception rectification poses a significant challenge. Abnormal perception in generative models is typically caused by two factors: inadequate model structure and imbalanced data distribution. In response to this issue, we propose the dynamic model perception rectification algorithm (DMPRA) for generalized generative models. The core idea is to gain a comprehensive perception of the data in the generative model by appropriately highlighting the low-density samples in the perception space, also known as the minor group samples. The entire process can be summarized as "search-evaluation-adjustment". To identify low-density regions in the data manifold within the perception space of generative models, we introduce a filtering method based on extended neighborhood sampling. Based on the informational value of samples from low-density regions, our proposed mechanism generates informative weights to assess the significance of these samples in correcting the models' perception. By using dynamic adjustment, DMPRA ensures simultaneous enhancement of diversity and quality in the presence of imbalanced data distribution. Experimental results indicate that the algorithm has effectively improved Generative Adversarial Nets (GANs), Normalizing Flows (Flows), Variational Auto-Encoders (VAEs), and Diffusion Models (Diffusion).
更多查看译文
AI 理解论文
溯源树
样例
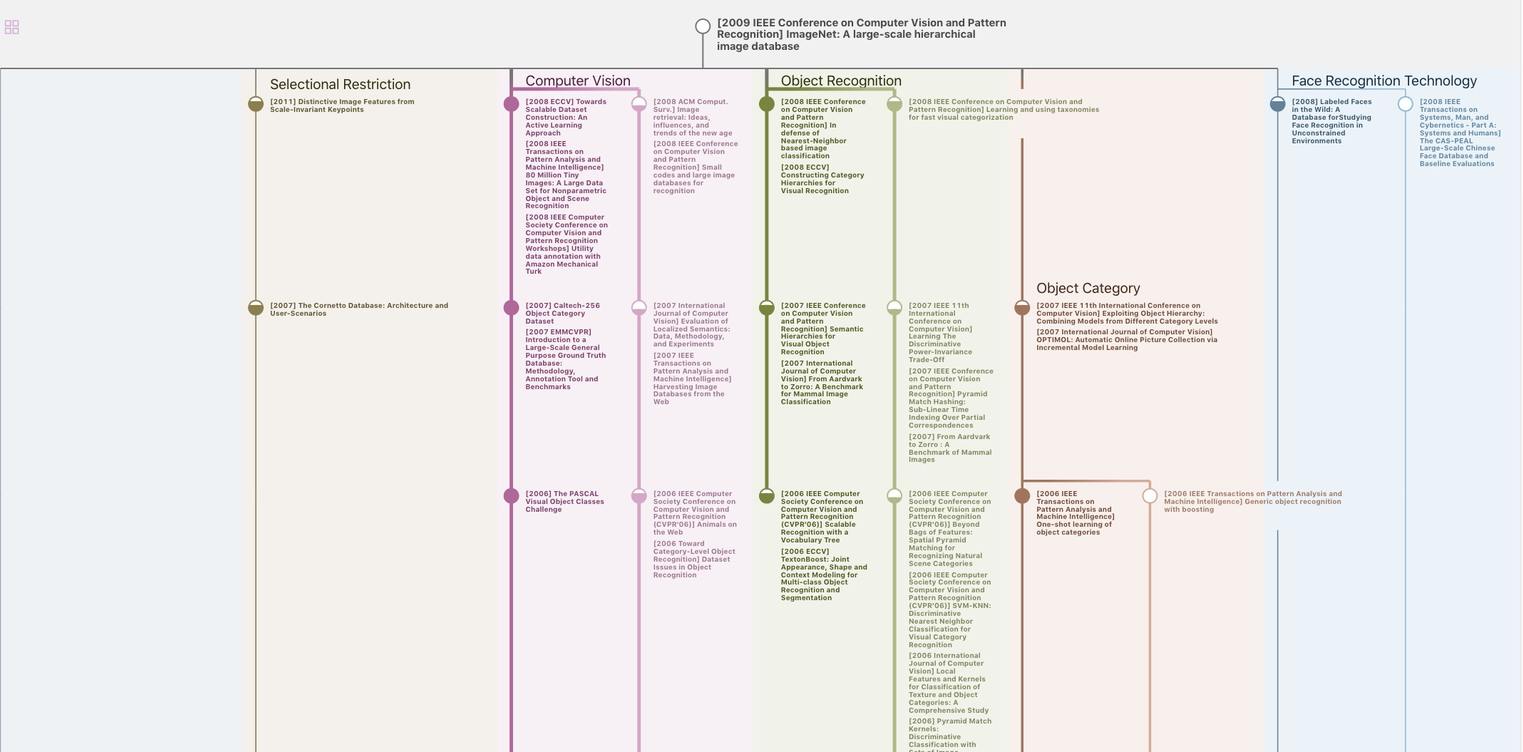
生成溯源树,研究论文发展脉络
Chat Paper
正在生成论文摘要