DAG-Aware Variational Autoencoder for Social Propagation Graph Generation
AAAI 2024(2024)
摘要
Propagation models in social networks are critical, with extensive applications across various fields and downstream tasks. However, existing propagation models are often oversimplified, scenario-specific, and lack real-world user social attributes. These limitations detaching from real-world analysis lead to inaccurate representations of the propagation process in social networks. To address these issues, we propose a User Features Attention-based DAG-Aware Variational Autoencoder (DAVA) for propagation graph generation. First, nearly 1 million pieces of user attributes data are collected. Then DAVA can integrate the analysis of propagation graph topology and corresponding user attributes as prior knowledge. By leveraging a lightweight attention-based framework and a sliding window mechanism based on BFS permutations weighted by user influence, DAVA significantly enhances the ability to generate realistic, large-scale propagation data, yielding graph scales ten times greater than those produced by existing SOTA methods. Every module of DAVA has flexibility and extension that allows for easy substitution to suit other generation tasks. Additionally, we provide a comprehensive evaluation of DAVA, one focus is the effectiveness of generated data in improving the performance of downstream tasks. During the generation process, we discover the Credibility Erosion Effect by modifying the generation rules, revealing a social phenomenon in social network propagation.
更多查看译文
关键词
DMKM: Graph Mining, Social Network Analysis & Community,APP: Social Networks
AI 理解论文
溯源树
样例
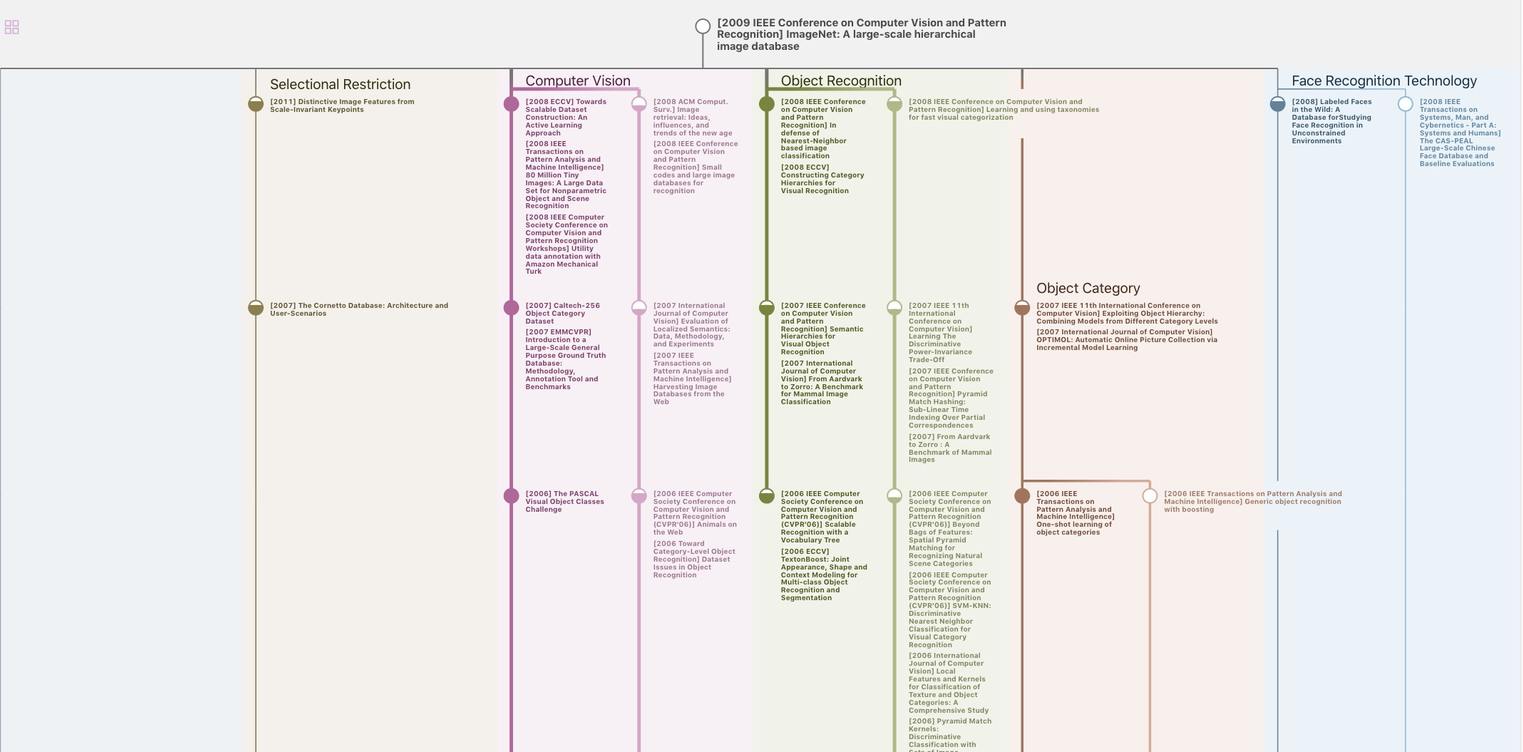
生成溯源树,研究论文发展脉络
Chat Paper
正在生成论文摘要