Fine-Tuning Large Language Model Based Explainable Recommendation with Explainable Quality Reward
Proceedings of the AAAI Conference on Artificial Intelligence(2024)
摘要
Large language model-based explainable recommendation (LLM-based ER) systems can provide remarkable human-like explanations and have widely received attention from researchers. However, the original LLM-based ER systems face three low-quality problems in their generated explanations, i.e., lack of personalization, inconsistency, and questionable explanation data. To address these problems, we propose a novel LLM-based ER model denoted as LLM2ER to serve as a backbone and devise two innovative explainable quality reward models for fine-tuning such a backbone in a reinforcement learning paradigm, ultimately yielding a fine-tuned model denoted as LLM2ER-EQR, which can provide high-quality explanations. LLM2ER-EQR can generate personalized, informative, and consistent high-quality explanations learned from questionable-quality explanation datasets. Extensive experiments conducted on three real-world datasets demonstrate that our model can generate fluent, diverse, informative, and highly personalized explanations.
更多查看译文
AI 理解论文
溯源树
样例
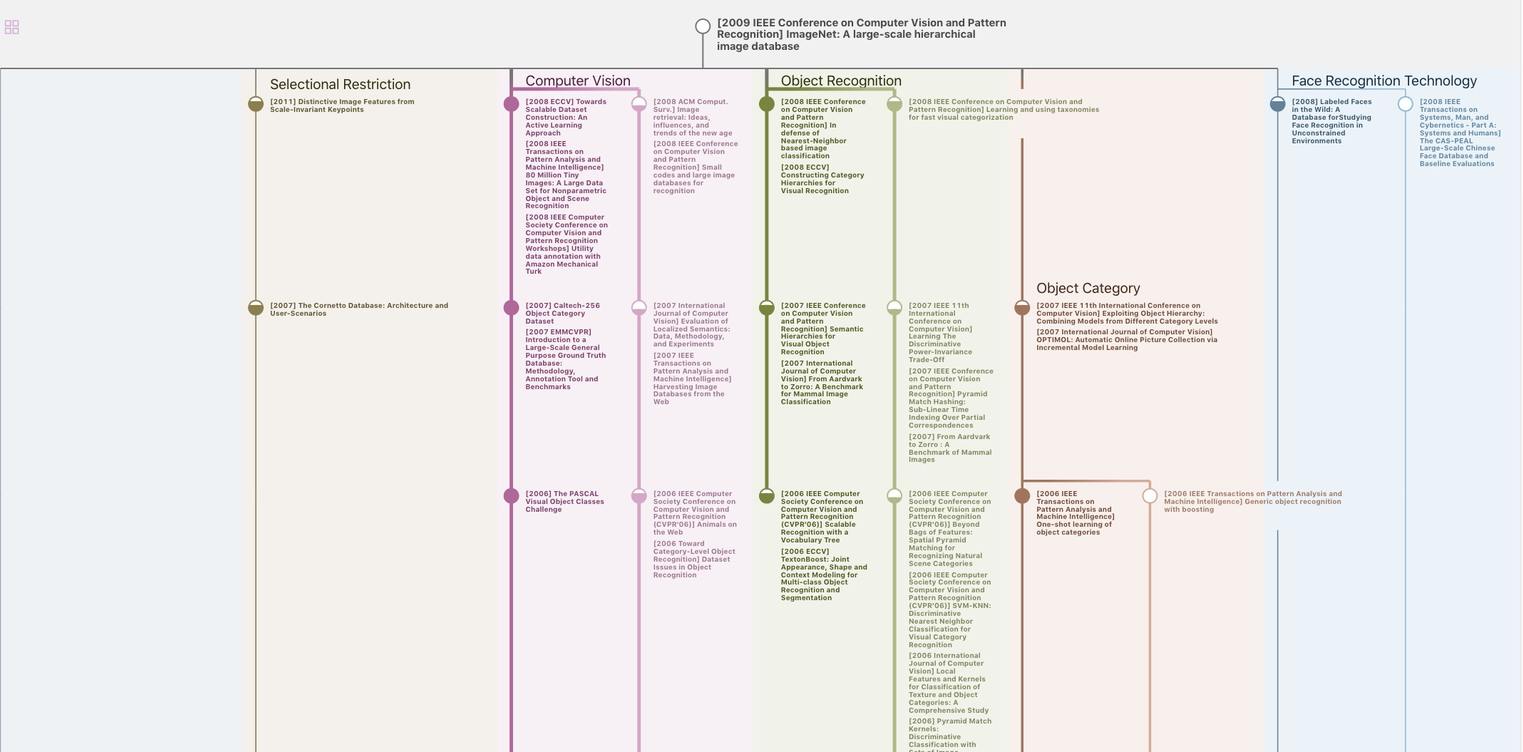
生成溯源树,研究论文发展脉络
Chat Paper
正在生成论文摘要